Hourly rolling correction of precipitation forecast via convolutional and long short-term memory networks
ATMOSPHERIC SCIENCE LETTERS(2022)
摘要
In order to improve precipitation forecast from GRAPES_Meso V4.0 in China, we propose a 1-6-h rolling correction solution, based on infrared (IR) channels from geostationary meteorological satellite and surface observation data. In particular, we design a deep learning extrapolation model to predict the evolution of cloud clusters based on convolutional neural networks and long short-term memory networks. The predicted cloud clusters, together with the relationship between the rainfall area and the cloud position, are applied to correct the 1-6-h precipitation forecast. We conduct comprehensive experiments to evaluate the proposed solution over China. Experimental results show that the deep learning model can successfully capture spatial characteristics and temporal variations between the sequences, and achieve reliable predictions of cloud clusters. The analysis further indicates that the rolling correction solution via the predicted cloud clusters has improved the precipitation forecast in China. The distribution of corrected precipitation forecast is more consistent with the observed precipitation compared to GRAPES_Meso forecast. In particular, the rolling correction model could enhance the forecast on "rain/no-rain" events, light rain, and moderate rain according to TS, ETS, BIAS, and FAR metrics.
更多查看译文
关键词
convolutional neural network, LSTM, precipitation forecast, rolling correction
AI 理解论文
溯源树
样例
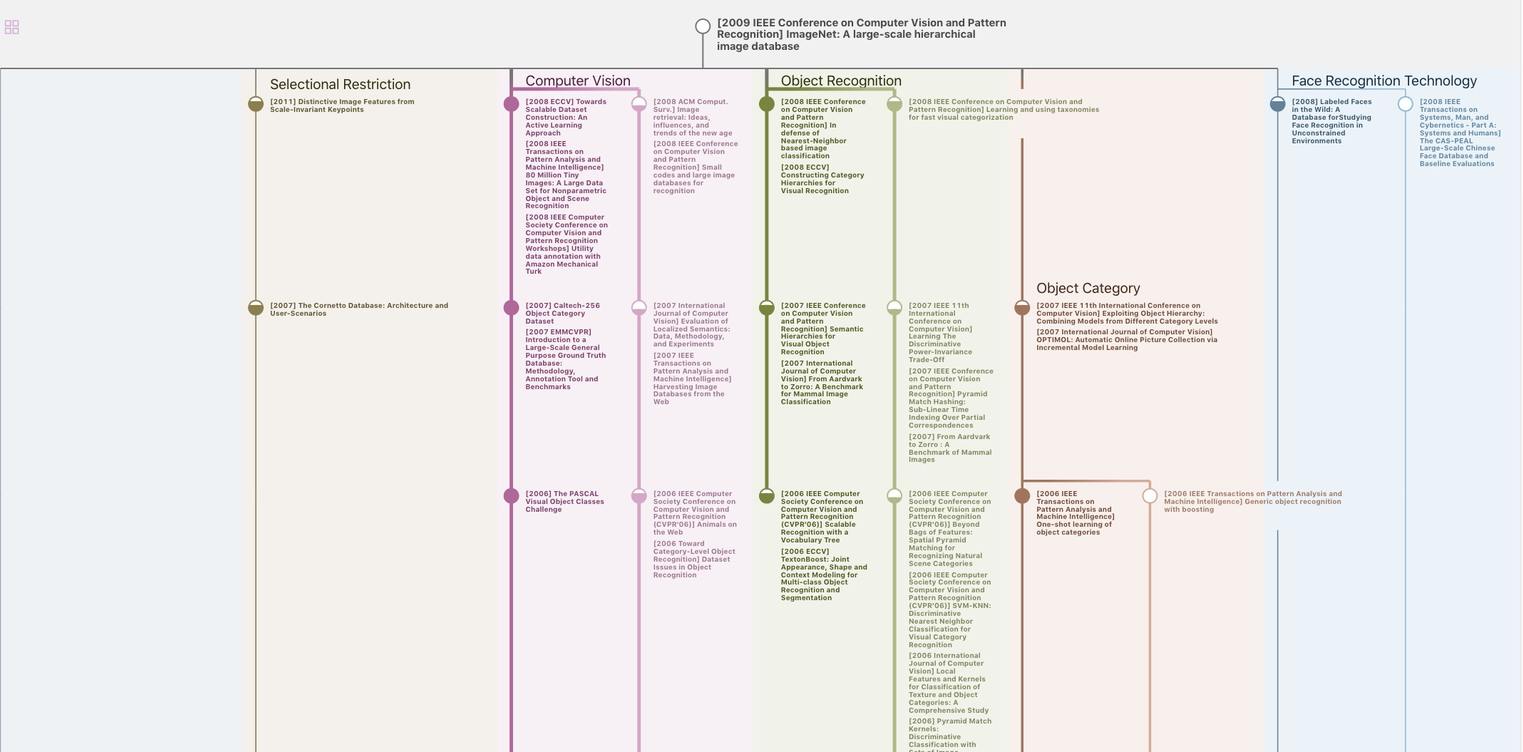
生成溯源树,研究论文发展脉络
Chat Paper
正在生成论文摘要