Combining Knowledge and Multi-modal Fusion for Meme Classification
MULTIMEDIA MODELING (MMM 2022), PT I(2022)
摘要
Internet memes are widespread on social media platforms such as Twitter and Facebook. Recently, meme classification has been an active research topic, especially meme sentiment classification and meme offensive classification. Internet memes contain multi-modal information, and the meme text is embedded in the meme image. The existing methods classify memes by simply concatenating global visual and textual features to generate a multi-modal representation. However, these approaches ignored the noise introduced by global visual features and the potential common information of meme multi-modal representation. In this paper, we propose a model for meme classification named MeBERT. Our method enhances the semantic representation of the meme by introducing conceptual information through external Knowledge Bases (KBs). Then, to reduce noise, a concept-image attention module is designed to extract concept-sensitive visual representation. In addition, a deep convolution tensor fusion module is built to effectively integrate multi-modal information. To verify the effectiveness of the model in the tasks of meme sentiment classification and meme offensive classification, we designed experiments on the Memotion and MultiOFF datasets. The experimental results show that the MeBERT model achieves better performance than state-of-the-art techniques for meme classification.
更多查看译文
关键词
Meme classification, Multi-modal fusion, Self-attention mechanism
AI 理解论文
溯源树
样例
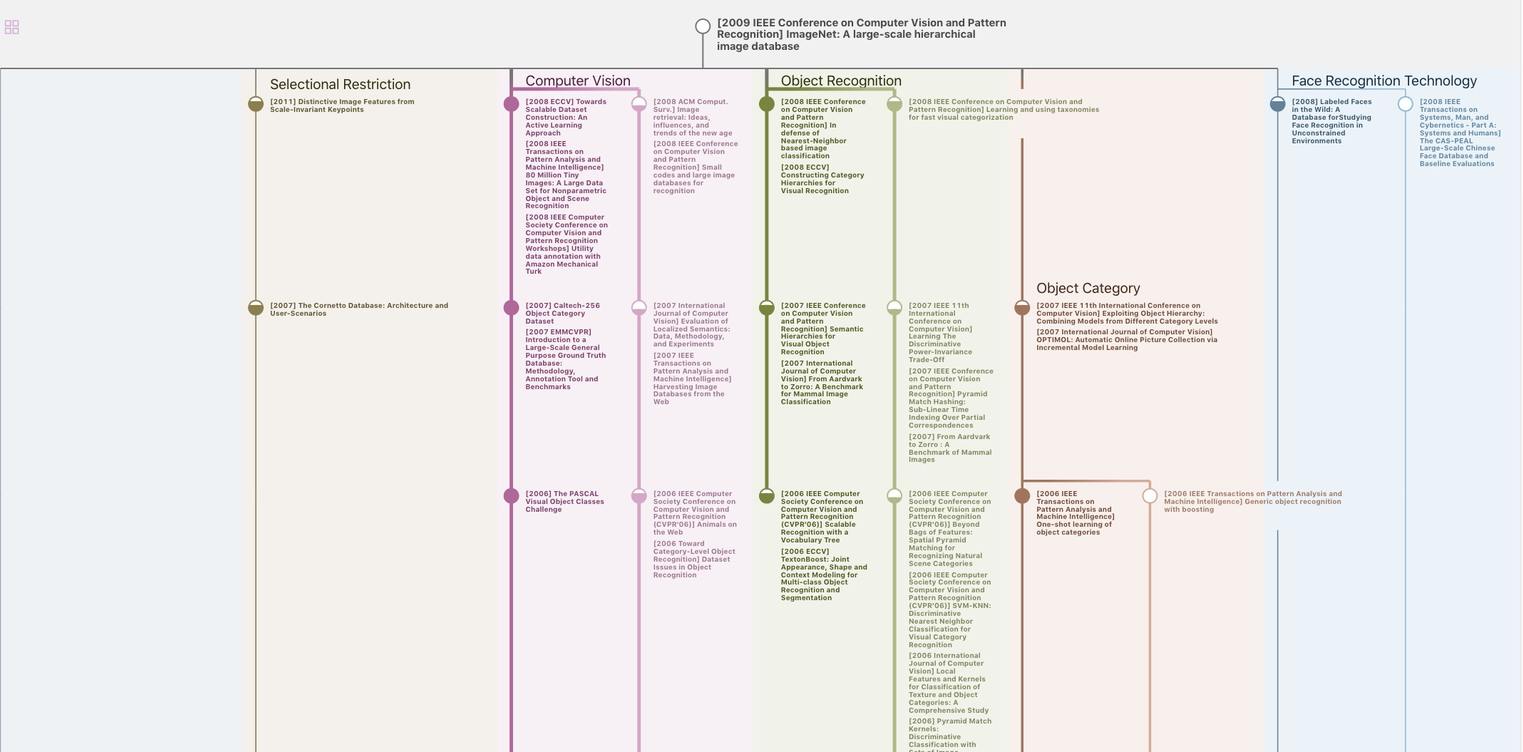
生成溯源树,研究论文发展脉络
Chat Paper
正在生成论文摘要