Exploring Implicit and Explicit Relations with the Dual Relation-Aware Network for Image Captioning
MULTIMEDIA MODELING, MMM 2022, PT II(2022)
摘要
Recently, Transformer based architectures using object region features and graph convolutional networks using scene graphs have made significant progress in the image captioning task. However, previous works paid little attention to discovering the high-level semantic relations in visual space. Specifically, they typically neglected the problem of relation mismatching between sentences and images, which may result in generating a pale list of image objects. From the perspective of alignment, there are elements such as objects, attributes, and relations in a sentence, but in visual space, there are only objects and their attributes that can be directly detected. Previous works merely focused on aligning objects and attributes between sentences and images while ignoring the relations that just appeared in sentences but cannot be visually observed in images. In this paper, we introduce a novel dual relation-aware network (DRAN) for image captioning which composes of a dual-path relation encoder and an adaptive context relation decoder to alleviate this problem. Concretely, the dual-path relation encoder in DRAN learns to encode implicit relations and explicit relations between objects into relation-aware features. Then the contextual gated fusion module in the decoder fuses adaptively two types of relation-aware features to help the decoder generate semantically richer captions. Experimental results on the MSCOCO dataset demonstrate the superiority of DRAN in relation encoding and learning, which indicates that the proposed DRAN can capture more semantic relations and details. These conclusions are reflected by the best performance of SPICE score and also by the visual examples illustrated qualitatively.
更多查看译文
关键词
Image captioning, Implicit and explicit relations, Dual relation-aware network, Transformer, Scene graph
AI 理解论文
溯源树
样例
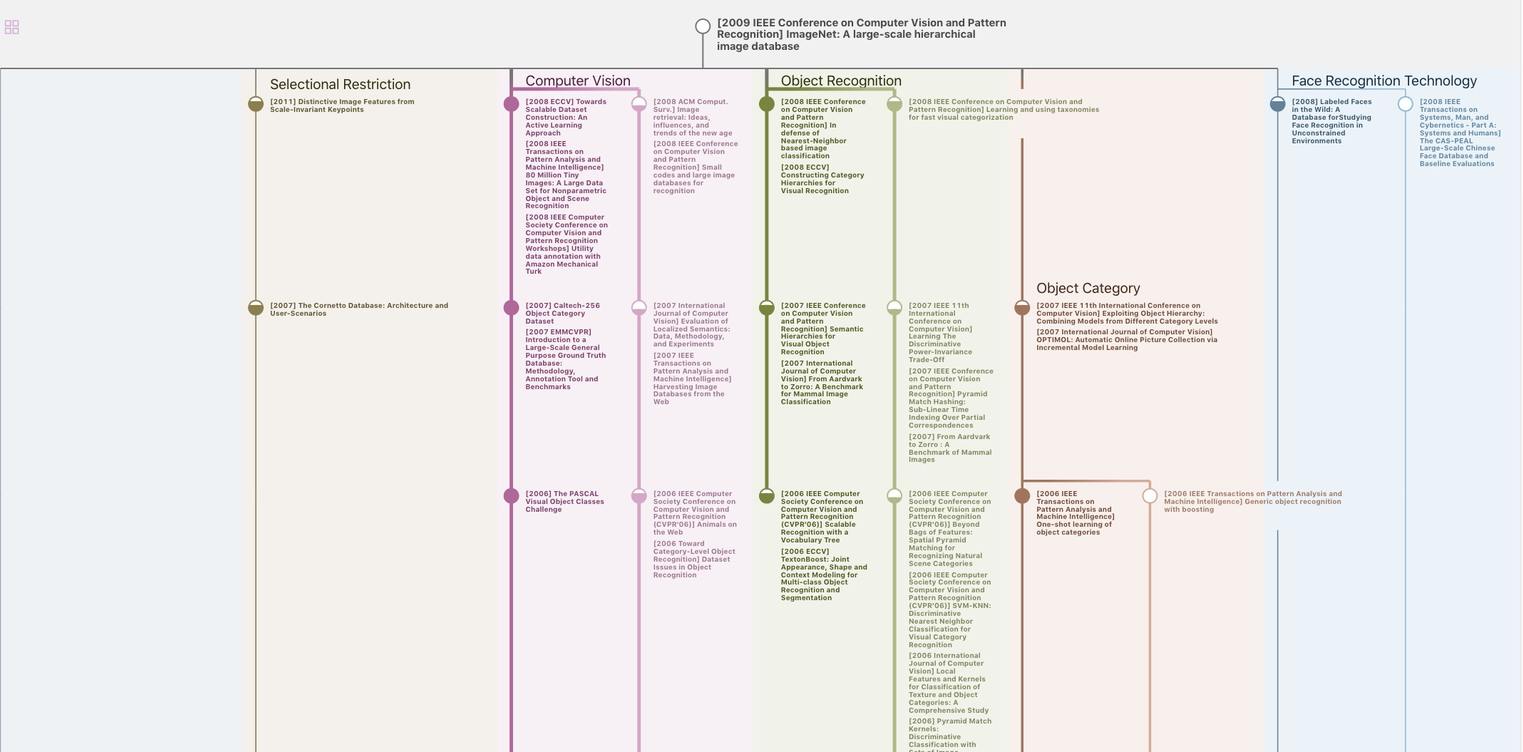
生成溯源树,研究论文发展脉络
Chat Paper
正在生成论文摘要