Traversability Transfer Learning Between Robots with Different Cost Assessment Policies
MODELLING AND SIMULATION FOR AUTONOMOUS SYSTEMS (MESAS 2021)(2022)
摘要
Predicting mobile robots' traversability over terrains is crucial to select safe and efficient paths through rough and unstructured environments. In multi-robot missions, knowledge transfer techniques can enable learning terrain traversability assessment the robots did not experience individually. The knowledge can be incrementally aggregated for homogeneous robots since they can treat foreign knowledge as their own. However, robots with different perceptions might experience the same terrain differently, so it is impossible to aggregate the shared knowledge directly. In this paper, we show how to learn a model that transfers the experience between heterogeneous robots, enabling each robot to use the whole sum of the experience of the multi-robot team. The proposed approach uses correlation to combine individual neural networks that assess the traversability of individual robots. The presented method has been verified in a real-world deployment of multi-legged walking robots with different cost assessment policies.
更多查看译文
关键词
Transfer learning, Traversability assessment, Mobile robot
AI 理解论文
溯源树
样例
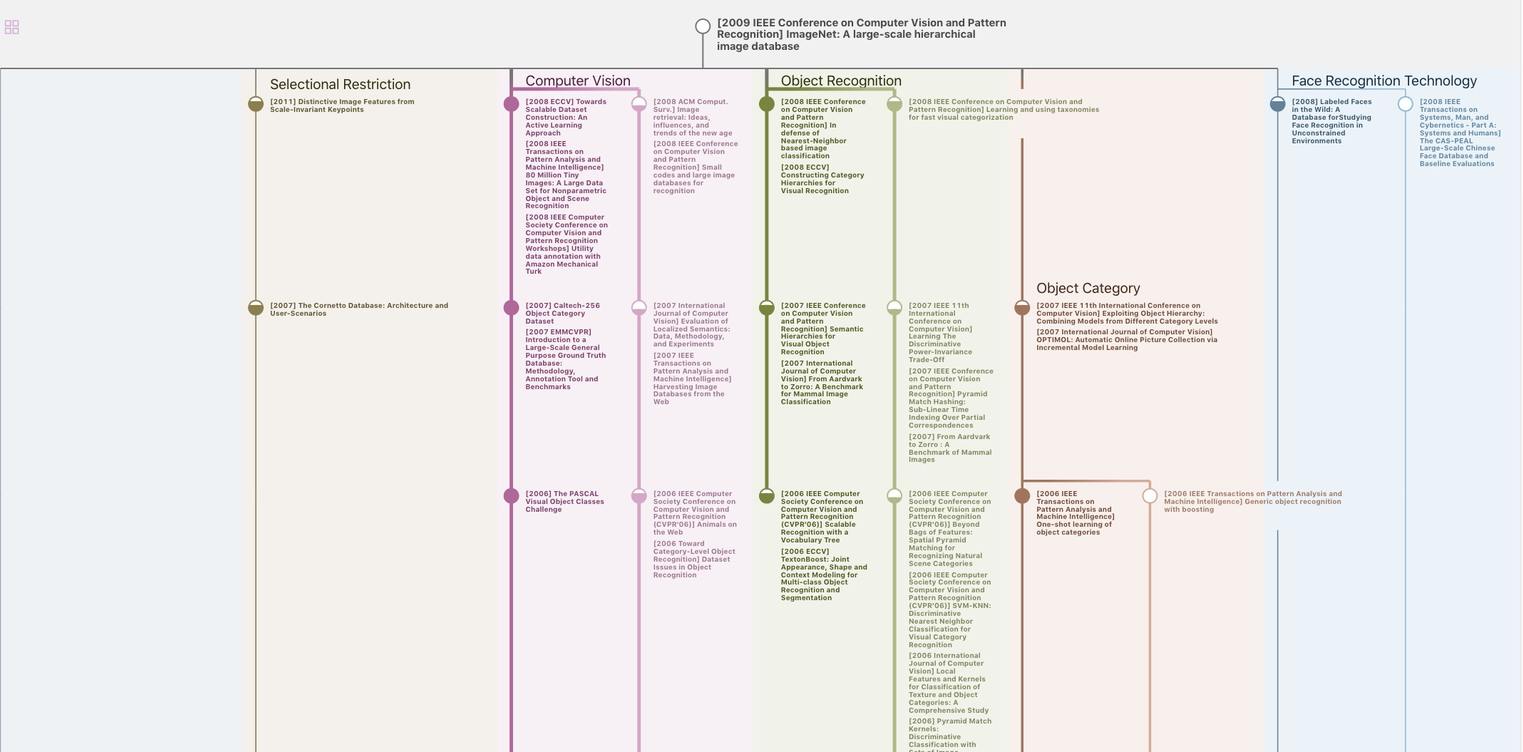
生成溯源树,研究论文发展脉络
Chat Paper
正在生成论文摘要