Soft measurement of ball mill load under variable working conditions based on deep transfer learning
MEASUREMENT SCIENCE AND TECHNOLOGY(2022)
摘要
In actual industrial production, labeled sample data of a ball mill is difficult to obtain under variable working conditions. Aiming to realize the soft measurement of ball mill load under variable working conditions, a joint discriminative high-order moment alignment network (JDMAN) is proposed, based on the deep transfer learning in this paper. With this method, discriminative features were learned through jointly training labeled samples belonging to the source domain and unlabeled samples belonging to the target domain. Simultaneously, the features learned by a deep convolution network were aligned and clustered through central moment discrepancy and center loss to accomplish transfer. The comparison with other transfer methods indicates that the proposed JDMAN can effectively promote the accuracy of soft measurement under variable working conditions.
更多查看译文
关键词
deep transfer learning, variable working condition, ball mill load, soft measurement
AI 理解论文
溯源树
样例
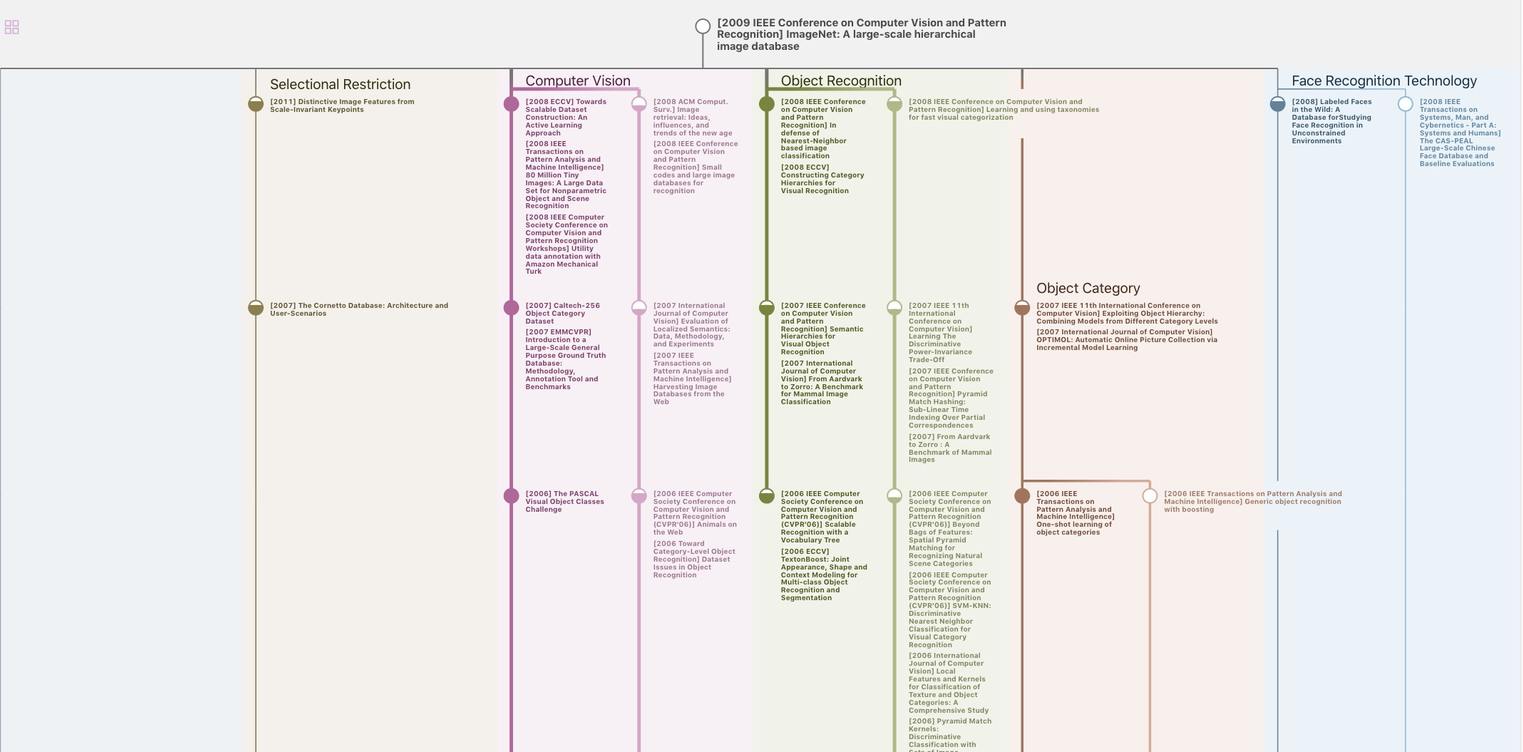
生成溯源树,研究论文发展脉络
Chat Paper
正在生成论文摘要