Spatiotemporal Deep Learning Model for Prediction of Taif Rose Phenotyping
AGRONOMY-BASEL(2022)
摘要
Despite being an important economic component of Taif region and the Kingdom of Saudi Arabia (KSA) as a whole, Taif rose experiences challenges because of uncontrolled conditions. In this study, we developed a phenotyping prediction model using deep learning (DL) that used simple and accurate methods to obtain and analyze data collected from ten rose farms. To maintain broad applicability and minimize computational complexity, our model utilizes a complementary learning approach in which both spatial and temporal instances of each dataset are processed simultaneously using three state-of-the-art deep neural networks: (1) convolutional neural network (CNN) to treat the image, (2) long short-term memory (LSTM) to treat the timeseries and (3) fully connected multilayer perceptions (MLPs)to obtain the phenotypes. As a result, this approach not only consolidates the knowledge gained from processing the same data from different perspectives, but it also leverages on the predictability of the model under incomplete or noisy datasets. An extensive evaluation of the validity of the proposed model has been conducted by comparing its outcomes with comprehensive phenotyping measurements taken from real farms. This evaluation demonstrates the ability of the proposed model to achieve zero mean absolute percentage error (MAPE) and mean square percentage error (MSPE) within a small number of epochs and under different training to testing schemes.
更多查看译文
关键词
Taif rose, machine learning, phenotypic traits, breeding, sustainable agriculture
AI 理解论文
溯源树
样例
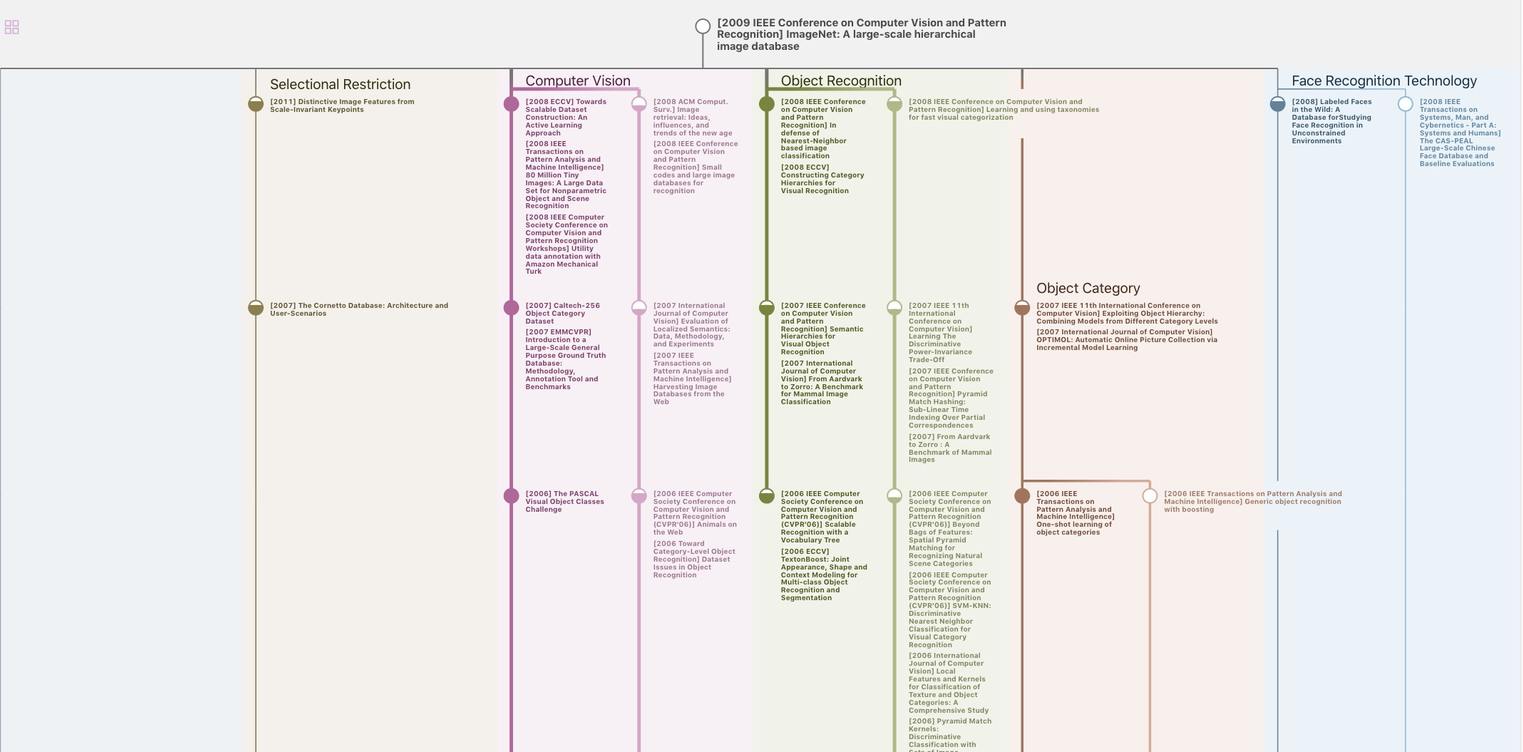
生成溯源树,研究论文发展脉络
Chat Paper
正在生成论文摘要