Multimode process monitoring based on hierarchical mode identification and stacked denoising autoencoder
CHEMICAL ENGINEERING SCIENCE(2022)
摘要
In modern plants, industrial processes generally operate under different modes, and reliable monitoring for such processes is highly important. One of the key challenges is how to accurately identify the various modes including steady modes and transitions modes. In this paper, a novel monitoring scheme based on hierarchical mode identification strategy and stacked denoising autoencoder (HMI-SDAE) is proposed for multimode processes. First, a novel mode division strategy called HMI is presented. In HMI, the Gaussian mixture model (GMM) is adopted to realize the preliminary identification of various modes by extracting the global distribution features of the variables. In this way, the whole multimode process is divided into multiple steady modes. An improved density peaks clustering algorithm based on local density relation search (LDRSDPC) is proposed to achieve the transition mode identification by fully utilizing the local distribution features of the process variables involved in any two adjacent steady modes and transition mode between them. A decision criterion combined with local density relation is constructed to automatically determine the clustering center. In this hierarchical way, multiple steady modes and transition modes are divided automatically and accurately. Secondly, the deep nonlinear features embedded in process variables are mined by SDAE, and the robust monitoring model is established for each steady mode. A monitoring statistic is constructed using the reconstruction error for detecting faults. The effectiveness and feasibility of the proposed HMI-SDAE monitoring scheme are illustrated with a numerical example and Tennessee Eastman (TE) process.(c) 2022 Elsevier Ltd. All rights reserved.
更多查看译文
关键词
Mode identification, Gaussian mixture model, Density peaks clustering, Stacked denoising autoencoder, Multimode process monitoring, Industrial process
AI 理解论文
溯源树
样例
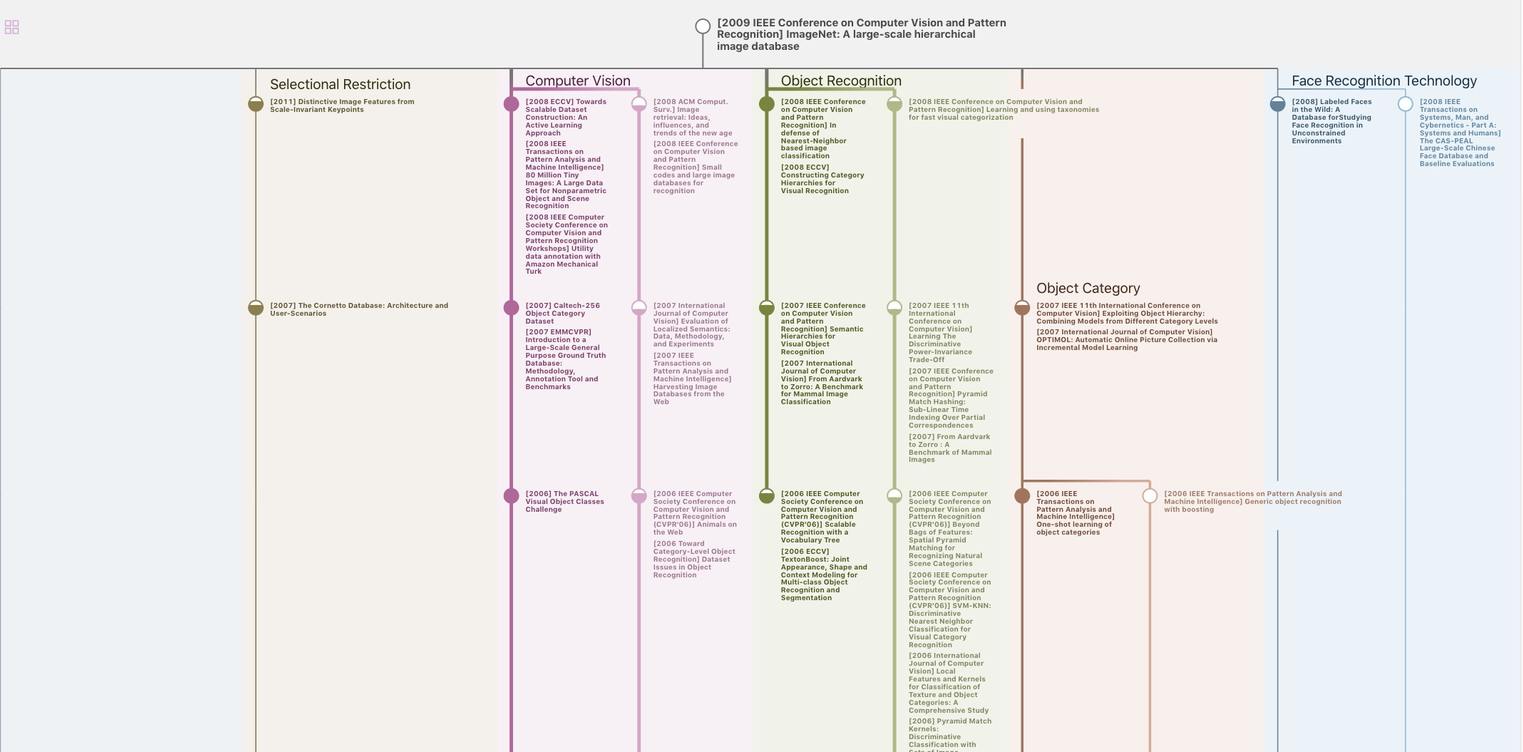
生成溯源树,研究论文发展脉络
Chat Paper
正在生成论文摘要