Classification of myocardial blood flow based on dynamic contrast-enhanced magnetic resonance imaging using hierarchical Bayesian models
JOURNAL OF THE ROYAL STATISTICAL SOCIETY SERIES C-APPLIED STATISTICS(2022)
摘要
Dynamic contrast-enhanced magnetic resonance imaging (DCE-MRI) is a promising approach to assess microvascular blood flow (perfusion) within the myocardium, and the Fermi microvascular perfusion model is widely applied to extract estimates of the myocardial blood flow (MBF) from DCE-MRI data sets. The classification of myocardial tissues into normal (healthy) and hypoperfused (lesion) regions provides new opportunities for the diagnosis of coronary heart disease and for advancing our understanding of the aetiology of this highly prevalent disease. In the present paper, the Fermi model is combined with a hierarchical Bayesian model (HBM) and a Markov random fields prior to automate this classification. The proposed model exploits spatial context information to smooth the MBF estimates while sharpening the edges between lesions and healthy tissues. The model parameters are approximately sampled from the posterior distribution with Markov chain Monte Carlo (MCMC), and we demonstrate that this enables robust classification of myocardial tissue elements based on estimated MBF, along with sound uncertainty quantification. A well-established traditional method, based on a Gaussian mixture model (GMM) trained with the expectation-maximisation algorithm, is used as a benchmark for comparison.
更多查看译文
关键词
classification, dynamic contrast-enhanced magnetic resonance imaging, hierarchical Bayesian model, Markov random fields prior, myocardial blood flow, myocardial perfusion
AI 理解论文
溯源树
样例
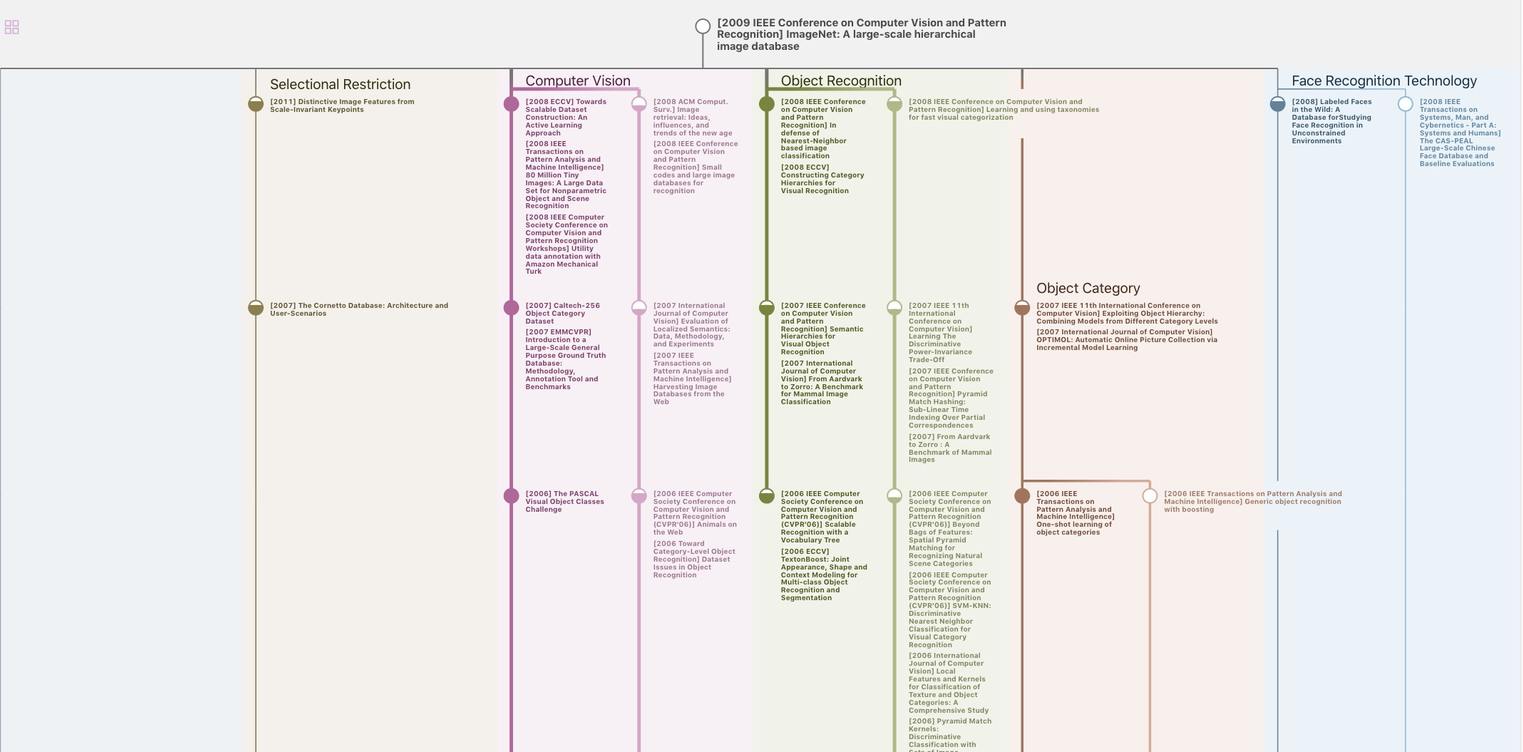
生成溯源树,研究论文发展脉络
Chat Paper
正在生成论文摘要