A Fusion Transformer for Multivariable Time Series Forecasting: The Mooney Viscosity Prediction Case
ENTROPY(2022)
摘要
Multivariable time series forecasting is an important topic of machine learning, and it frequently involves a complex mix of inputs, including static covariates and exogenous time series input. A targeted investigation of this input data is critical for improving prediction performance. In this paper, we propose the fusion transformer (FusFormer), a transformer-based model for forecasting time series data, whose framework fuses various computation modules for time series input and static covariates. To be more precise, the model calculation consists of two parallel stages. First, it employs a temporal encoder-decoder framework for extracting dynamic temporal features from time series data input, which analyzes and integrates the relative position information of sequence elements into the attention mechanism. Simultaneously, the static covariates are fed to the static enrichment module, which is inspired by gated linear units, to suppress irrelevant information and control the extent of nonlinear processing. Finally, the prediction results are calculated by fusing the outputs of the above two stages. Using Mooney viscosity forecasting as a case study, we demonstrate considerable forecasting performance improvements over existing methodologies and verify the effectiveness of each component of FusFormer via ablation analysis, and an interpretability use case is conducted to visualize temporal patterns of time series. The experimental results prove that FusFormer can achieve accurate Mooney viscosity prediction and improve the efficiency of the tire production process.
更多查看译文
关键词
multivariate time series forecasting,deep learning,positional encoding,static covariates,transformer,Mooney viscosity
AI 理解论文
溯源树
样例
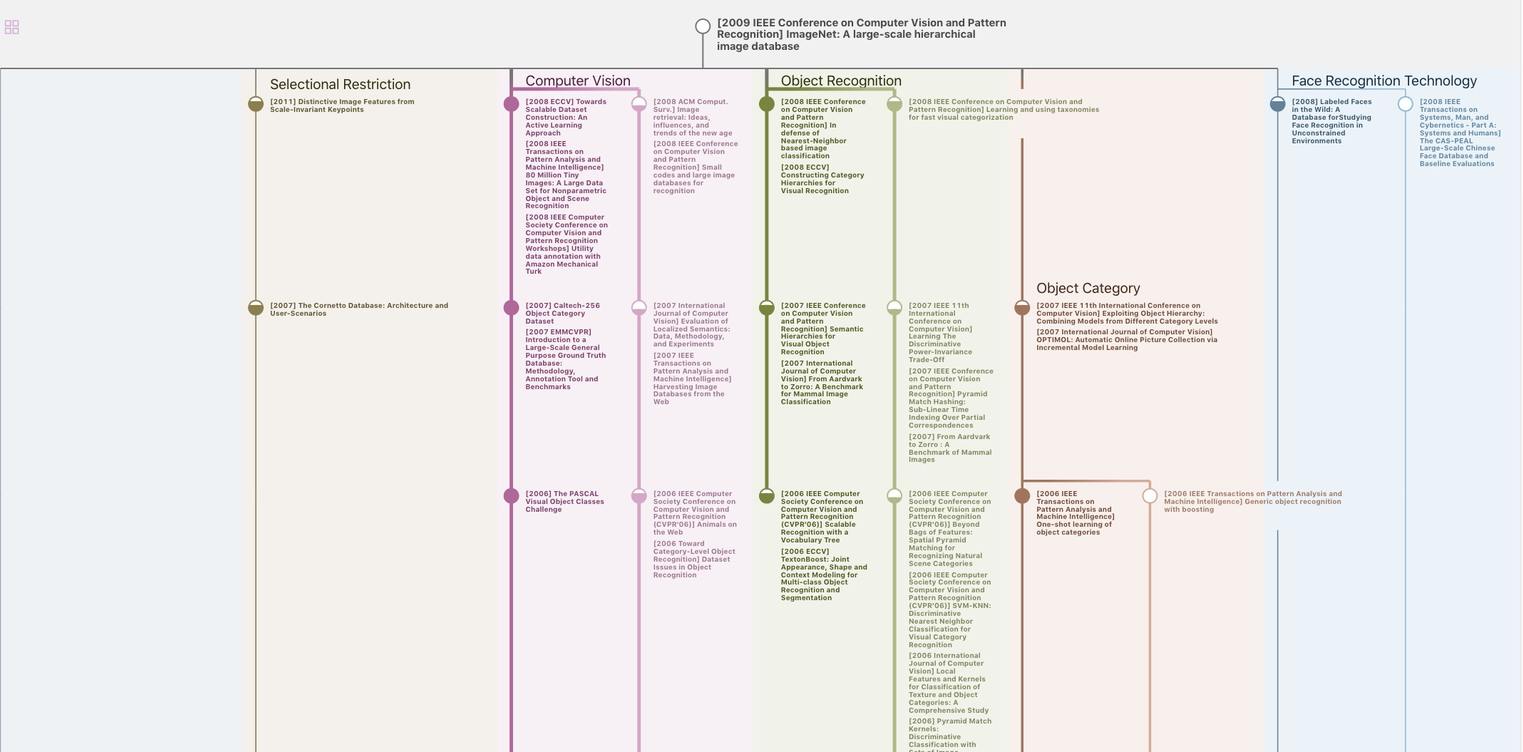
生成溯源树,研究论文发展脉络
Chat Paper
正在生成论文摘要