Best-Arm Identification Using Extreme Value Theory Estimates of the CVaR
JOURNAL OF RISK AND FINANCIAL MANAGEMENT(2022)
摘要
We consider a risk-aware multi-armed bandit framework with the goal of avoiding catastrophic risk. Such a framework has multiple applications in financial risk management. We introduce a new conditional value-at-risk (CVaR) estimation procedure combining extreme value theory with automated threshold selection by ordered goodness-of-fit tests, and we apply this procedure to a pure exploration best-arm identification problem under a fixed budget. We empirically compare our results with the commonly used sample average estimator of the CVaR, and we show a significant performance improvement when the underlying arm distributions are heavy-tailed.
更多查看译文
关键词
sequential decision making, multi-armed bandits, conditional value-at-risk, extreme value theory, heavy-tailed distributions, risk-aware reinforcement learning
AI 理解论文
溯源树
样例
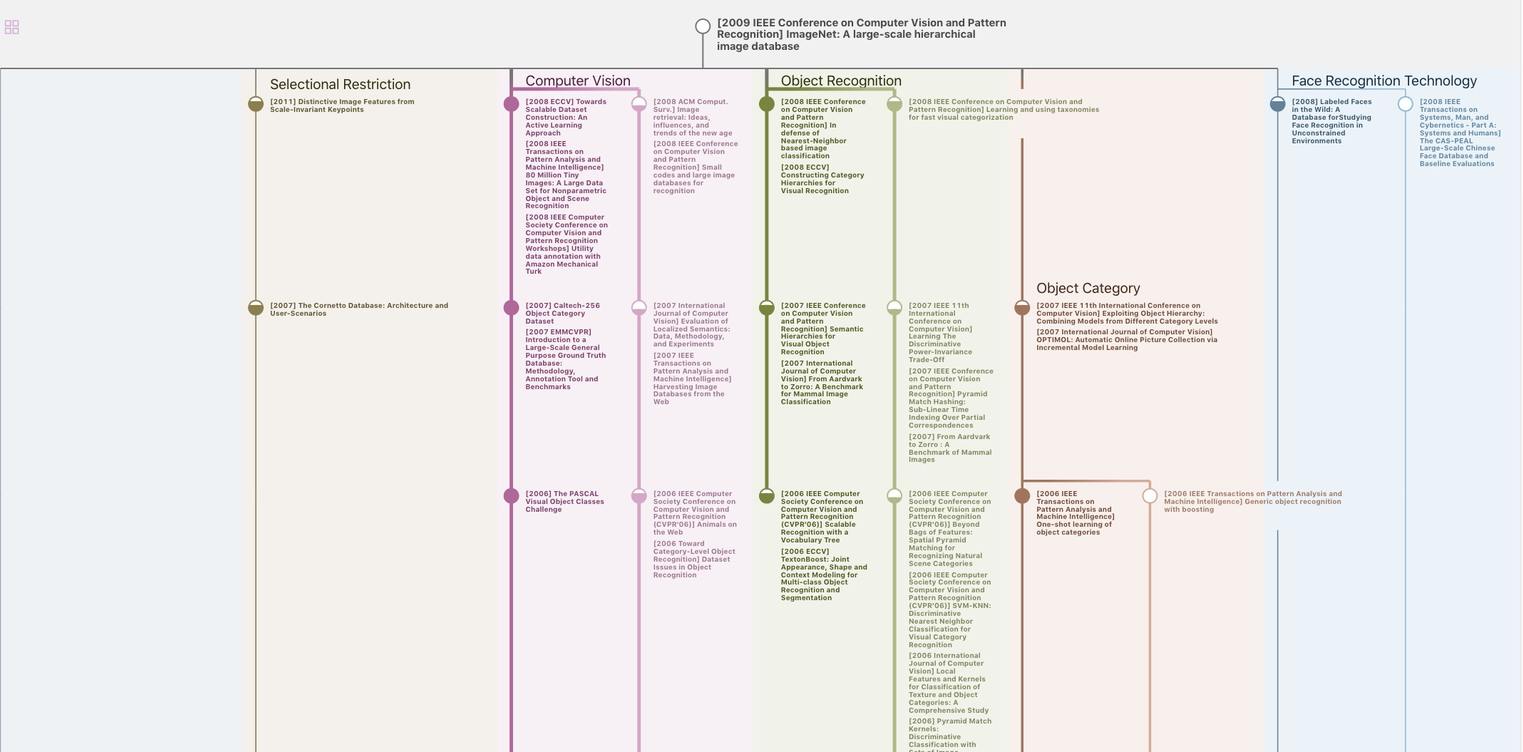
生成溯源树,研究论文发展脉络
Chat Paper
正在生成论文摘要