Bayesian Calibration of Performance Degradation in a Gas Turbine-Driven Compressor Unit for Prognosis Health Management
JOURNAL OF ENGINEERING FOR GAS TURBINES AND POWER-TRANSACTIONS OF THE ASME(2022)
摘要
Prognosis health management is an effective way to improve the operational safety and economy of industrial equipment. The development of an accurate and quick response model to monitor equipment health status, predict performance, and diagnose faults is key to its implementation. However, the inevitable performance degradation of industrial equipment over time poses a significant challenge to such a model. In this work, we adopt a Bayesian approach to calibrate thermodynamic simulations with time-dependent parameters that account for performance degradation. The relationship between degradation and time is modeled through an assumed functional form, referred to as a health indicator. The proposed health indicator calibration method gives a rapid assessment of degraded equipment performance and elucidates how degradation relates to time. The novelty of this paper is that it regards performance degradation as an uncertainty quantification problem rather than a deterministic problem. The health indicator calibration method is validated on a natural gas compressor and a gas turbine. The results show that when severe degradation occurs, functional calibration improves predictive performance over nonfunctional calibration (i.e., independent of time). The introduced method provides valuable decision support to extend the service life and reduce maintenance costs for industrial equipment. Its feedback in operation can also develop the service assessment criteria and inform the design of subsequent generations of industrial equipment.
更多查看译文
关键词
functional Bayesian calibration, performance degradation, uncertainty quantification, Gaussian process, prognosis health management
AI 理解论文
溯源树
样例
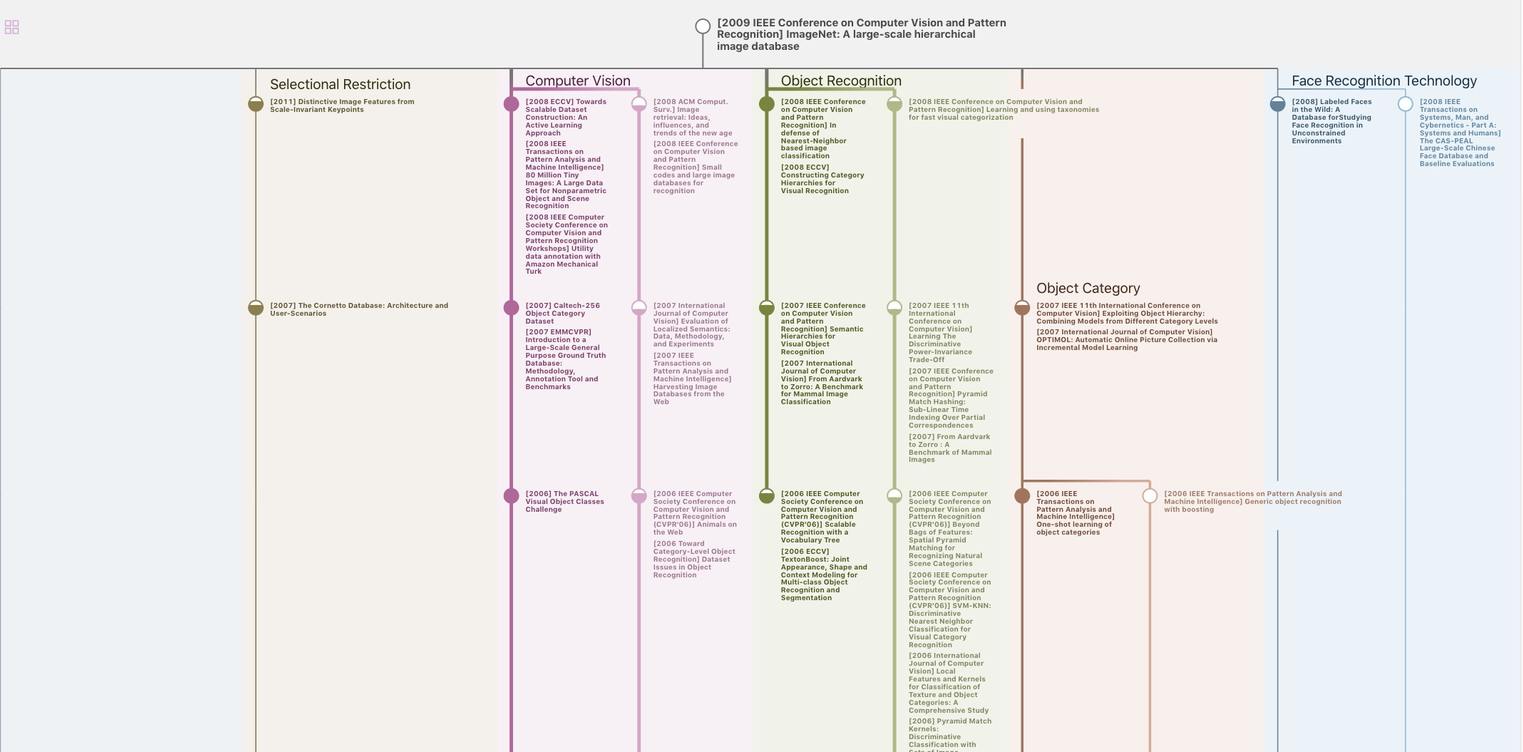
生成溯源树,研究论文发展脉络
Chat Paper
正在生成论文摘要