Multi-source and multivariate ozone prediction based on fuzzy cognitive maps and evidential reasoning theory
APPLIED SOFT COMPUTING(2022)
摘要
Ozone prediction, a key role for ozone pollution control, is facing the following challenges, i.e., the complex evolution trend of ozone, the cross-interference phenomena between ozone and other pollutants, and the low-quality monitoring data. To overcome the above challenges, we propose a multi-source and multivariate ozone prediction model based on fuzzy cognitive maps (FCMs) and evidential reasoning theory from the perspective of spatio-temporal fusion, termed as ERC-FCM. In this framework, an FCM-based prediction model is introduced to solve the ozone forecasting problem. Inspired by the multivariate time series forecasting, a multivariate ozone prediction problem is modeled as an FCM learned by the real-coded genetic algorithm, in which each node denotes a variable (pollutant). Thus, both the complex evolution trend of ozone and the cross-interference phenomena can be reflected by the FCM. Further, we propose an ensemble theoretical framework based on evidence reasoning theory and the matrix 2 norm. This theoretical framework relieves the negative factors from the low-quality monitoring data and improves the prediction accuracy when facing multi-source and multivariate time series. The performance of ERC-FCM is validated on two real-world datasets. The experimental results demonstrate that our method yields the best prediction performance by comparison with the other classical FCM-based methods on mean absolute error (MAE), mean square error (MSE), and root mean square error (RMSE). In addition, the Friedman test and Nemenyi test show that ERC-FCM gets relatively better prediction accuracy than other models.(c) 2022 Elsevier B.V. All rights reserved.
更多查看译文
关键词
Ozone prediction, Time series analysis, Fuzzy cognitive map, Ensemble learning, Evidential reasoning theory
AI 理解论文
溯源树
样例
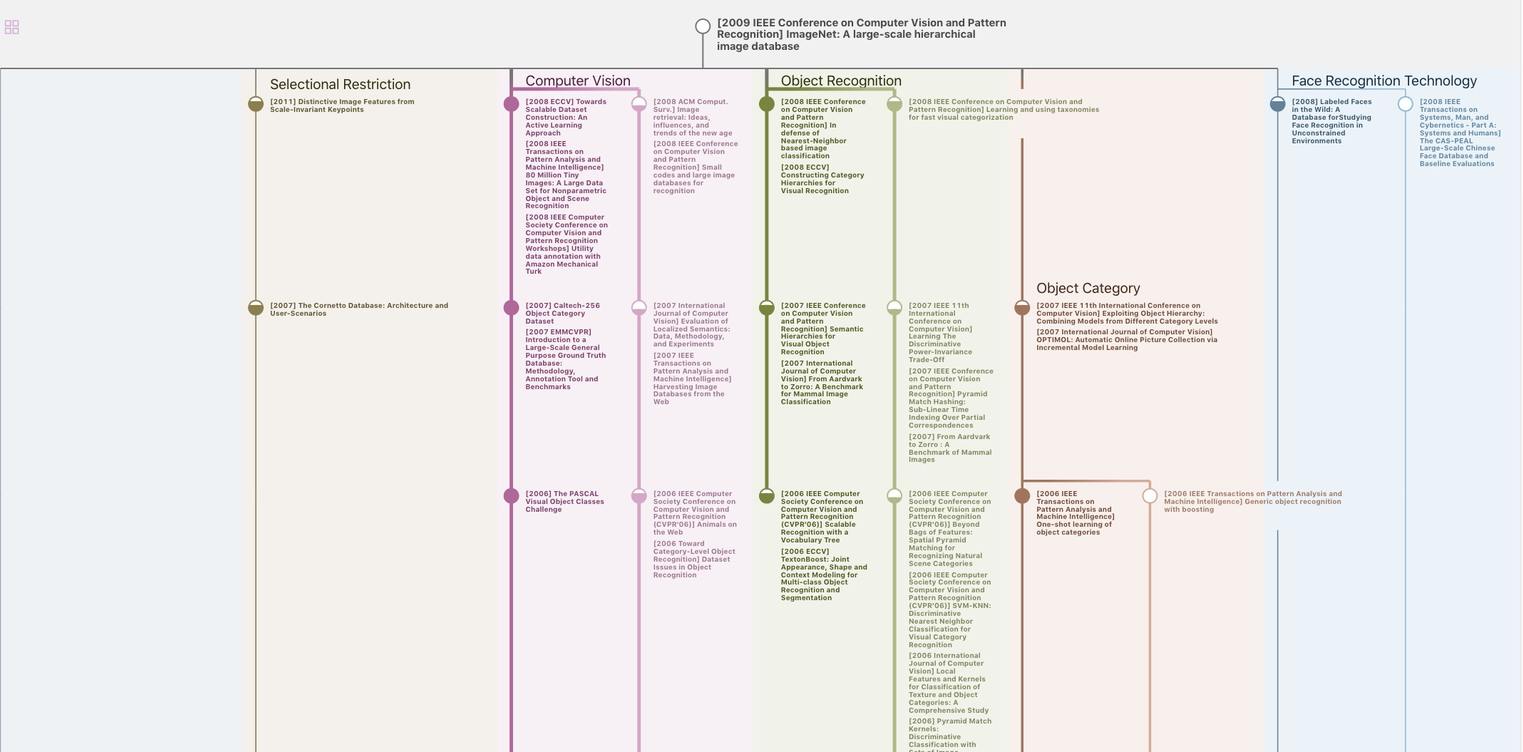
生成溯源树,研究论文发展脉络
Chat Paper
正在生成论文摘要