Parallel adaptive guidance network for image inpainting
Applied Intelligence(2022)
摘要
Motivated by human behavior, dividing inpainting tasks into structure reconstruction and texture generation helps to simplify restoration process and avoid distorted structures and blurry textures. However, most of tasks are ineffective for dealing with large continuous holes. In this paper, we devise a parallel adaptive guidance network(PAGN), which repairs structures and enriches textures through parallel branches, and several intermediate-level representations in different branches guide each other via the vertical skip connection and the guidance filter, ensuring that each branch only leverages the desirable features of another and outputs high-quality contents. Considering that the larger the missing regions are, less information is available. We promote the joint-contextual attention mechanism(Joint-CAM), which explores the connection between unknown and known patches by measuring their similarity at the same scale and at different scales, to utilize the existing messages fully. Since strong feature representation is essential for generating visually realistic and semantically reasonable contents in the missing regions, we further design attention-based multiscale perceptual res2blcok(AMPR) in the bottleneck that extracts features of various sizes at granular levels and obtains relatively precise object locations. Experiments on the public datasets CelebA-HQ, Places2, and Paris show that our proposed model is superior to state-of-the-art models, especially for filling large holes.
更多查看译文
关键词
Image inpainting, Parallel adaptive guidance network, Joint-contextual attention mechanism, Multiscale receptive fields
AI 理解论文
溯源树
样例
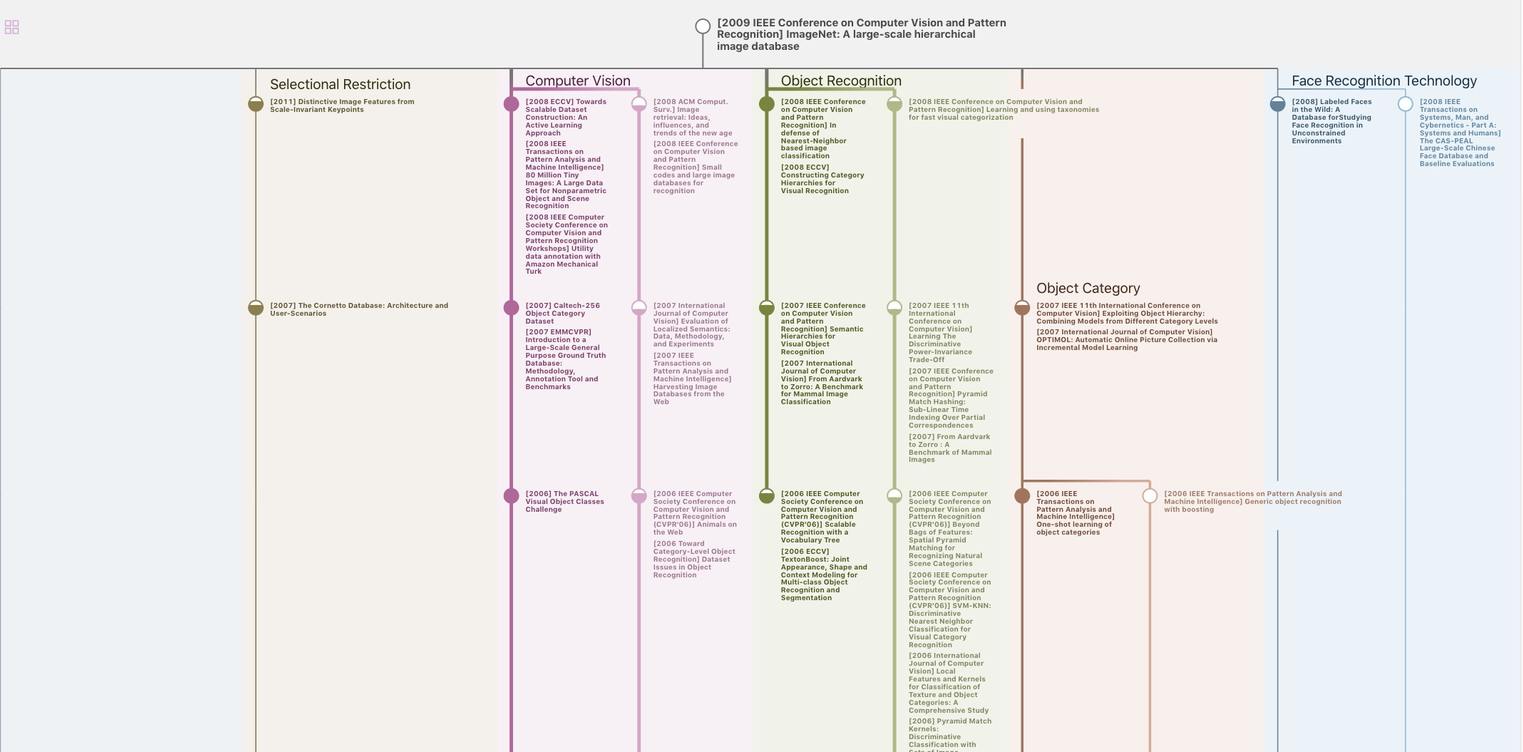
生成溯源树,研究论文发展脉络
Chat Paper
正在生成论文摘要