Zero-Shot Face Swapping with De-identification Adversarial Learning
PARALLEL AND DISTRIBUTED COMPUTING, APPLICATIONS AND TECHNOLOGIES, PDCAT 2021(2022)
摘要
In this paper, we propose a Zero-shot Face Swapping Network (ZFSNet) to swap novel identities where no training data is available, which is very practical. In contrast to many existing methods that consist of several stages, the proposed model can generate images containing the unseen identity in a single forward pass without fine-tuning. To achieve it, based on the basic encoder-decoder framework, we propose an additional de-identification (De-ID) module after the encoder to remove the source identity information, which contributes to removing the source identity retaining in the encoding stream and improves the model's generalization capability. Then we introduce an attention component (ASSM) to blend the encoded source feature and the target identity feature adaptively. It amplifies proper local details and helps the decoder attend to the related identity feature. Extensive experiments evaluated on the synthesized and real images demonstrate that the proposed modules are effective in zero-shot face swapping. In addition, we also evaluate our framework on zero-shot facial expression translation to show its versatility and flexibility.
更多查看译文
关键词
Face swapping, Facial expression translation, Adversarial learning
AI 理解论文
溯源树
样例
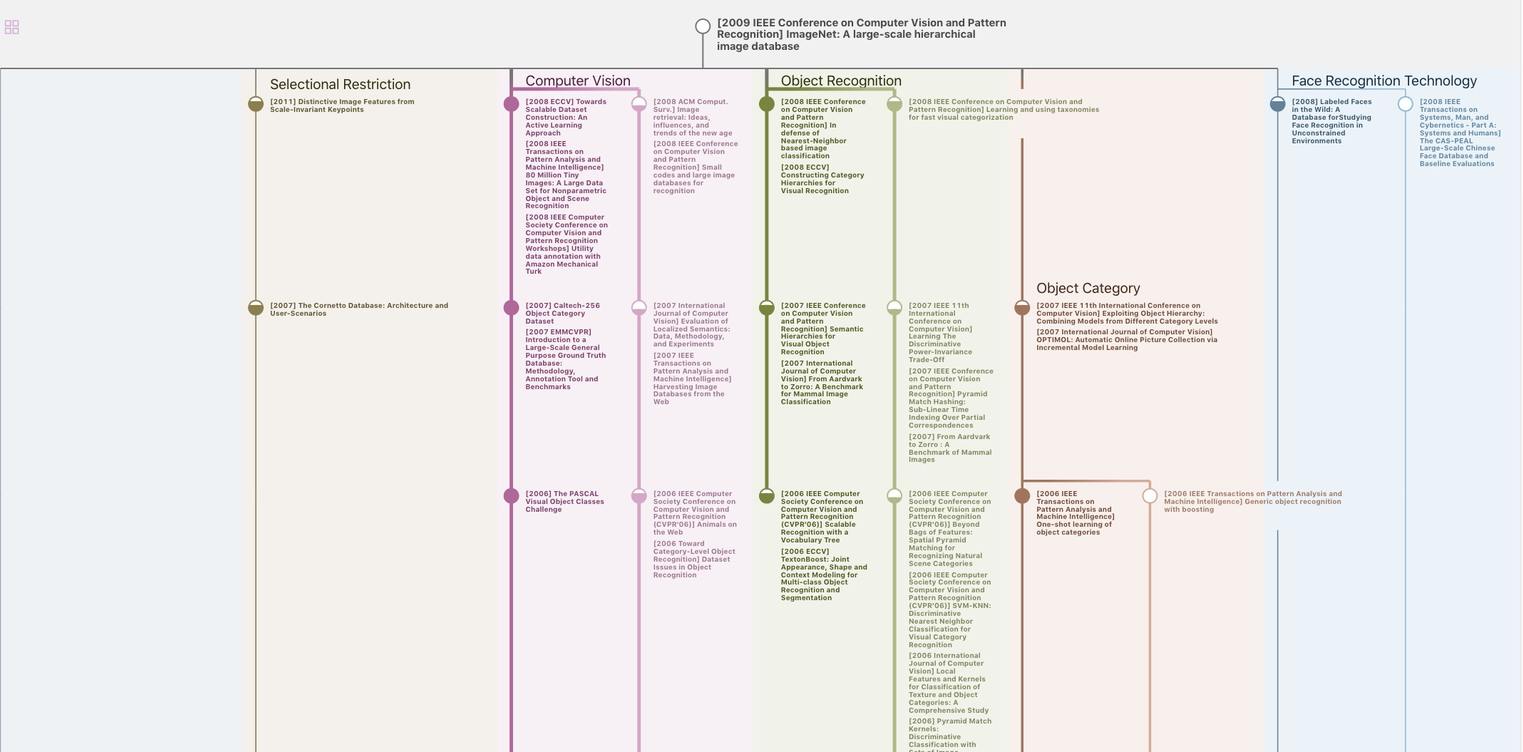
生成溯源树,研究论文发展脉络
Chat Paper
正在生成论文摘要