Mitigating the Multicollinearity Problem and Its Machine Learning Approach: A Review
MATHEMATICS(2022)
摘要
Technologies have driven big data collection across many fields, such as genomics and business intelligence. This results in a significant increase in variables and data points (observations) collected and stored. Although this presents opportunities to better model the relationship between predictors and the response variables, this also causes serious problems during data analysis, one of which is the multicollinearity problem. The two main approaches used to mitigate multicollinearity are variable selection methods and modified estimator methods. However, variable selection methods may negate efforts to collect more data as new data may eventually be dropped from modeling, while recent studies suggest that optimization approaches via machine learning handle data with multicollinearity better than statistical estimators. Therefore, this study details the chronological developments to mitigate the effects of multicollinearity and up-to-date recommendations to better mitigate multicollinearity.
更多查看译文
关键词
multicollinearity, variable selection methods, optimization approaches, neural network, machine learning
AI 理解论文
溯源树
样例
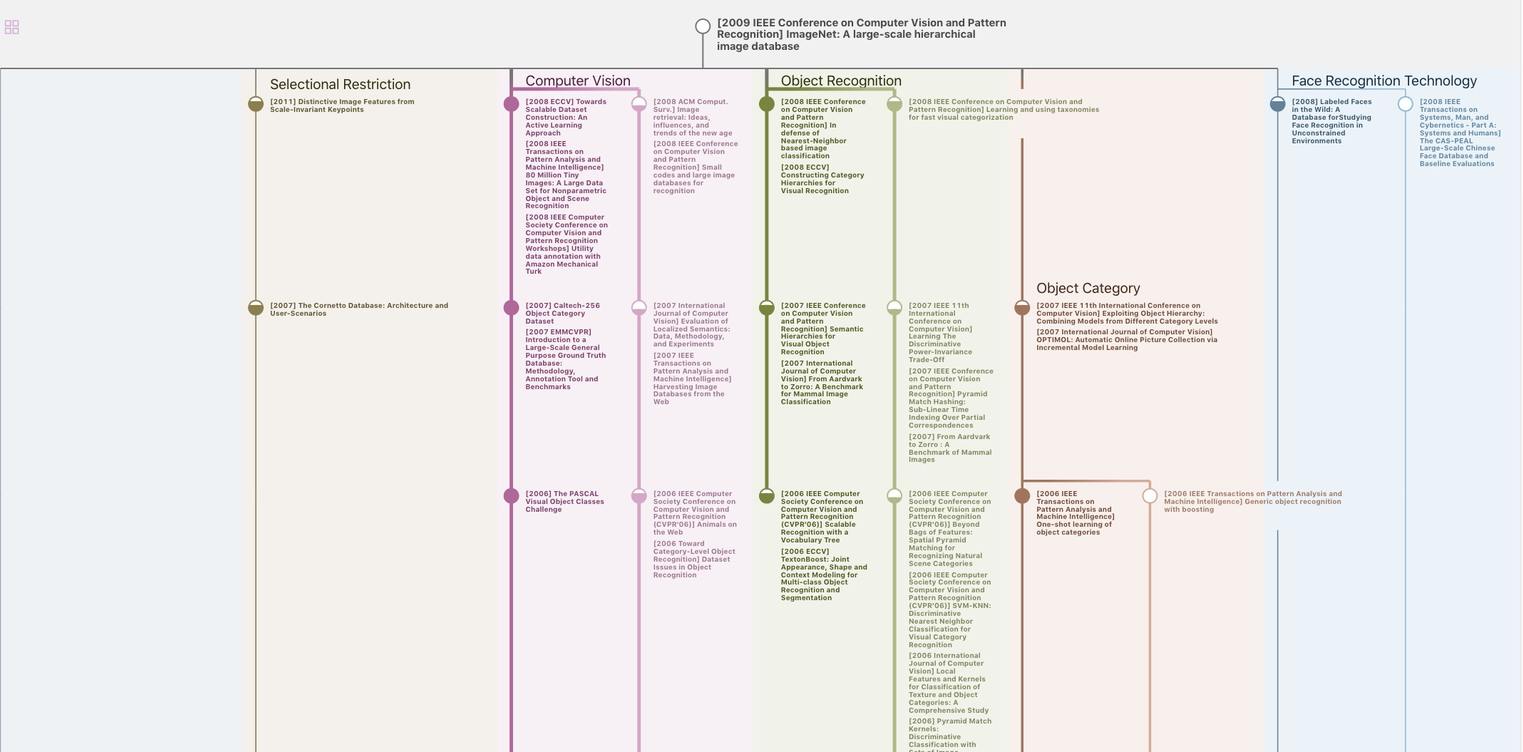
生成溯源树,研究论文发展脉络
Chat Paper
正在生成论文摘要