Variable Decomposition for Large-Scale Constrained Optimization Problems Using a Grouping Genetic Algorithm
MATHEMATICAL AND COMPUTATIONAL APPLICATIONS(2022)
摘要
Several real optimization problems are very difficult, and their optimal solutions cannot be found with a traditional method. Moreover, for some of these problems, the large number of decision variables is a major contributing factor to their complexity; they are known as Large-Scale Optimization Problems, and various strategies have been proposed to deal with them. One of the most popular tools is called Cooperative Co-Evolution, which works through a decomposition of the decision variables into smaller subproblems or variables subgroups, which are optimized separately and cooperate to finally create a complete solution of the original problem. This kind of decomposition can be handled as a combinatorial optimization problem where we want to group variables that interact with each other. In this work, we propose a Grouping Genetic Algorithm to optimize the variable decomposition by reducing their interaction. Although the Cooperative Co-Evolution approach is widely used to deal with unconstrained optimization problems, there are few works related to constrained problems. Therefore, our experiments were performed on a test benchmark of 18 constrained functions under 100, 500, and 1000 variables. The results obtained indicate that a Grouping Genetic Algorithm is an appropriate tool to optimize the variable decomposition for Large-Scale Constrained Optimization Problems, outperforming the decomposition obtained by a state-of-the-art genetic algorithm.
更多查看译文
关键词
Grouping Genetic Algorithm, variable decomposition, Large-Scale Constrained Optimization
AI 理解论文
溯源树
样例
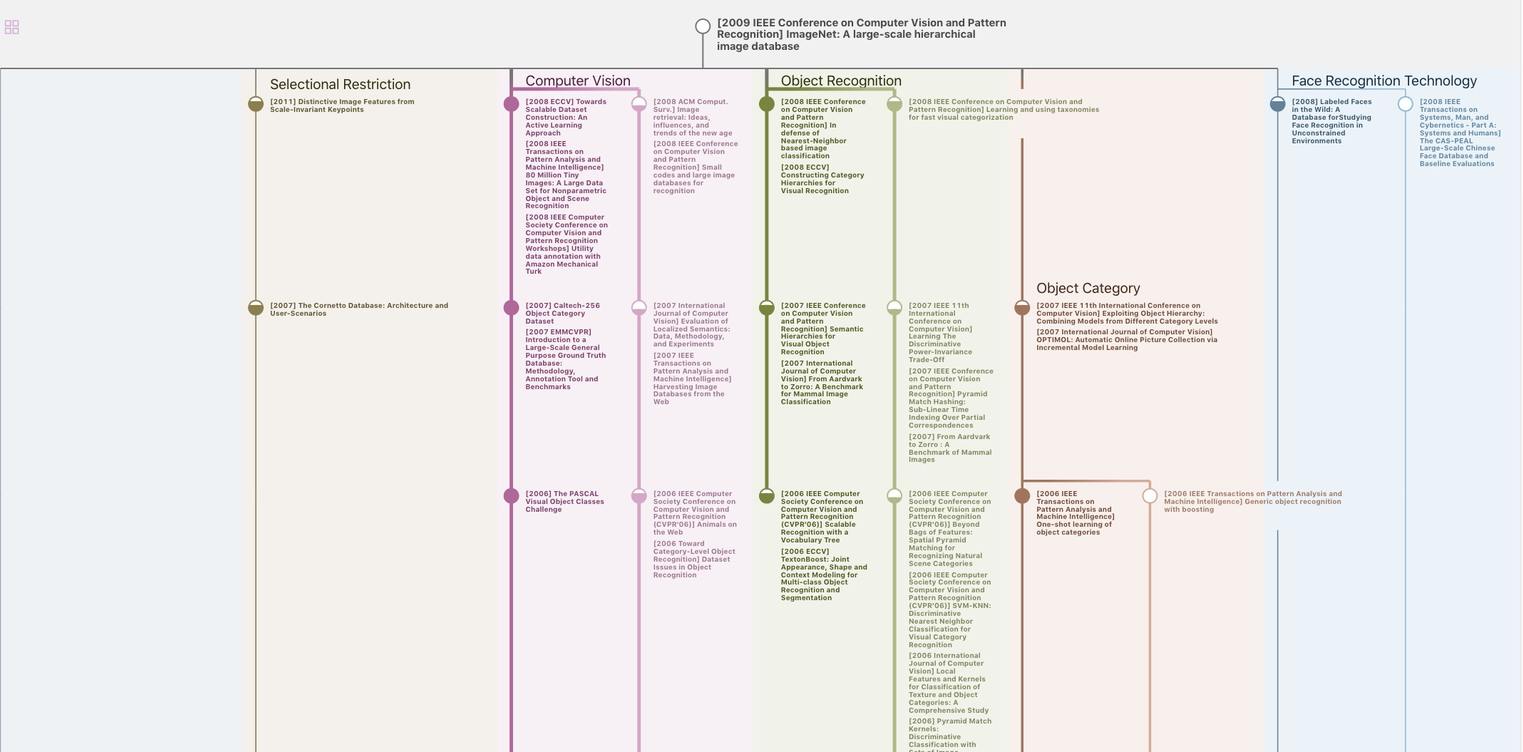
生成溯源树,研究论文发展脉络
Chat Paper
正在生成论文摘要