Jointly Super Resolution and Degradation Learning on Unpaired Real-World Images
PARALLEL AND DISTRIBUTED COMPUTING, APPLICATIONS AND TECHNOLOGIES, PDCAT 2021(2022)
摘要
Recently super-resolution methods based on CNN have achieved amazing success. However, the effects of these methods on real-world images are not available. The main reason is that most of them use bicubic downsampling by default to obtain degraded low-resolution images, while the degradation process of real-world images is unknown. In our work, we argue that image degradation and super-resolution are tightly coupled. In order to complete this cycle, we propose a framework to jointly learn the degradation and super-resolution of real-world images. At the same time, in order to stabilize learning and optimize performance, we have combined a variety of image content losses. Our framework can not only achieve real-world super-resolution, but also generate paired unknown degraded datasets for other super-resolution methods. The experiments on the NTIRE2020 real-world SR dataset show the effectiveness of our model.
更多查看译文
关键词
Super-resolution, Real-world images, Deep learning
AI 理解论文
溯源树
样例
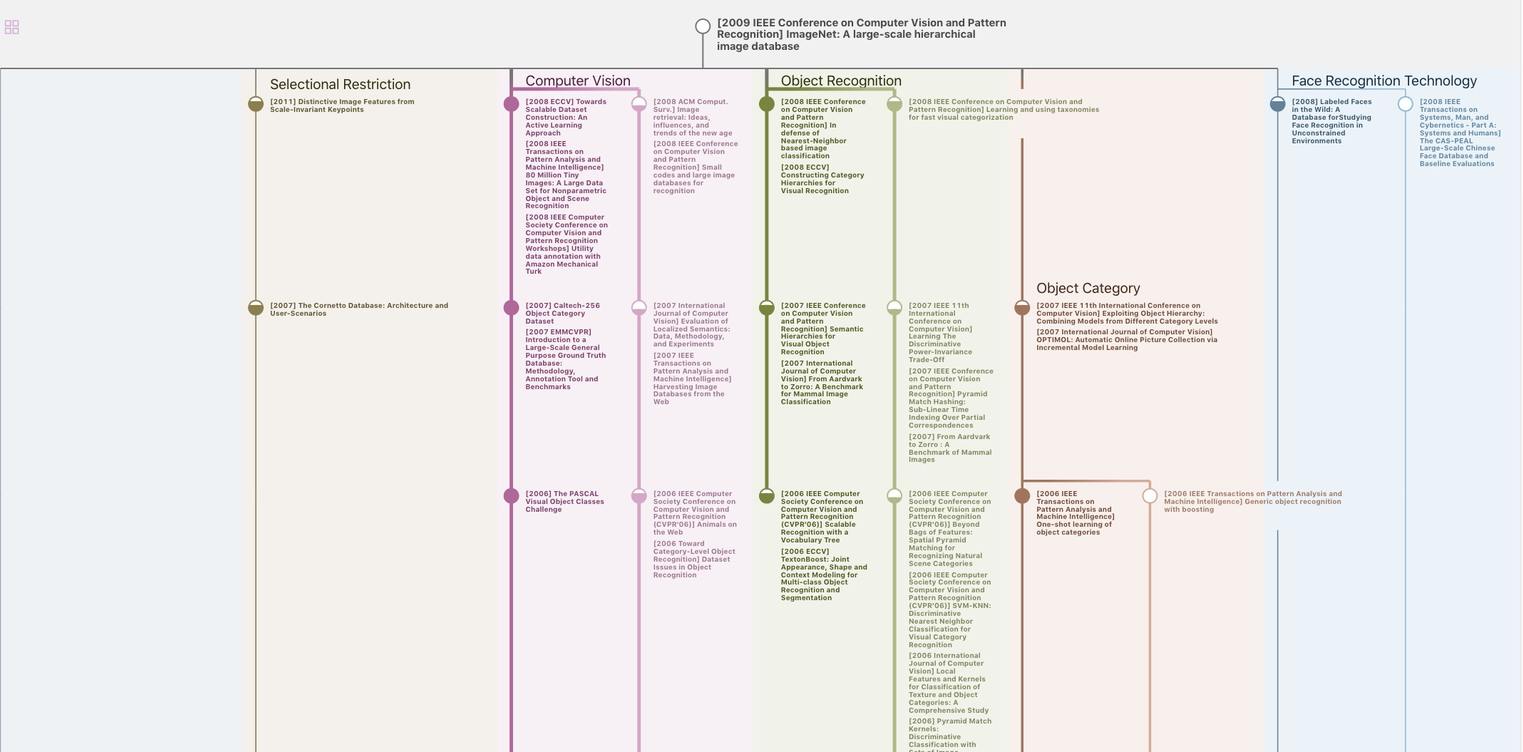
生成溯源树,研究论文发展脉络
Chat Paper
正在生成论文摘要