Automatic soil crack recognition under uneven illumination condition with the application of artificial intelligence
Engineering Geology(2022)
摘要
Drought-induced soil desiccation cracking has attracted great attention in various disciplines with the advent of global climate change. Accurately obtaining soil crack networks is essential to understand the cracking mechanism. Inspired by recent advances of artificial intelligence (AI) in computer vision, we propose a new automatic soil crack recognition method based a novel network architecture, named Attention Res-UNet. Deep Res-UNet inherits both the advantages from residual learning for training deeper networks and U-Net for semantic segmentation. Moreover, attention mechanism is utilized to alleviate the influences caused by the uneven illumination conditions. Firstly, the soil crack images under different uneven illumination conditions are collected to create a new soil cracking image dataset. Then, traditional method and multiple state-of-the-art deep learning based different semantic segmentation models are tested on our collected dataset. Finally, a professional evaluation standard, which considers both the overall metrics (precision, recall, dice, surface crack ratio) and details (crack total length, average crack width, number of crack segments) of the soil crack features is proposed to evaluate the recognition results of the different models. Extensive experimental results demonstrate the superiority of our proposed Attention Res-UNet approach compared with traditional methods and other deep learning models in recognizing soil cracks under complex environmental conditions. Our method is also suitable for crack recognition of other materials under complex environmental conditions.
更多查看译文
关键词
Soil desiccation cracking,Artificial intelligence,Uneven illumination,Deep learning,Attention gate,Evaluation standard
AI 理解论文
溯源树
样例
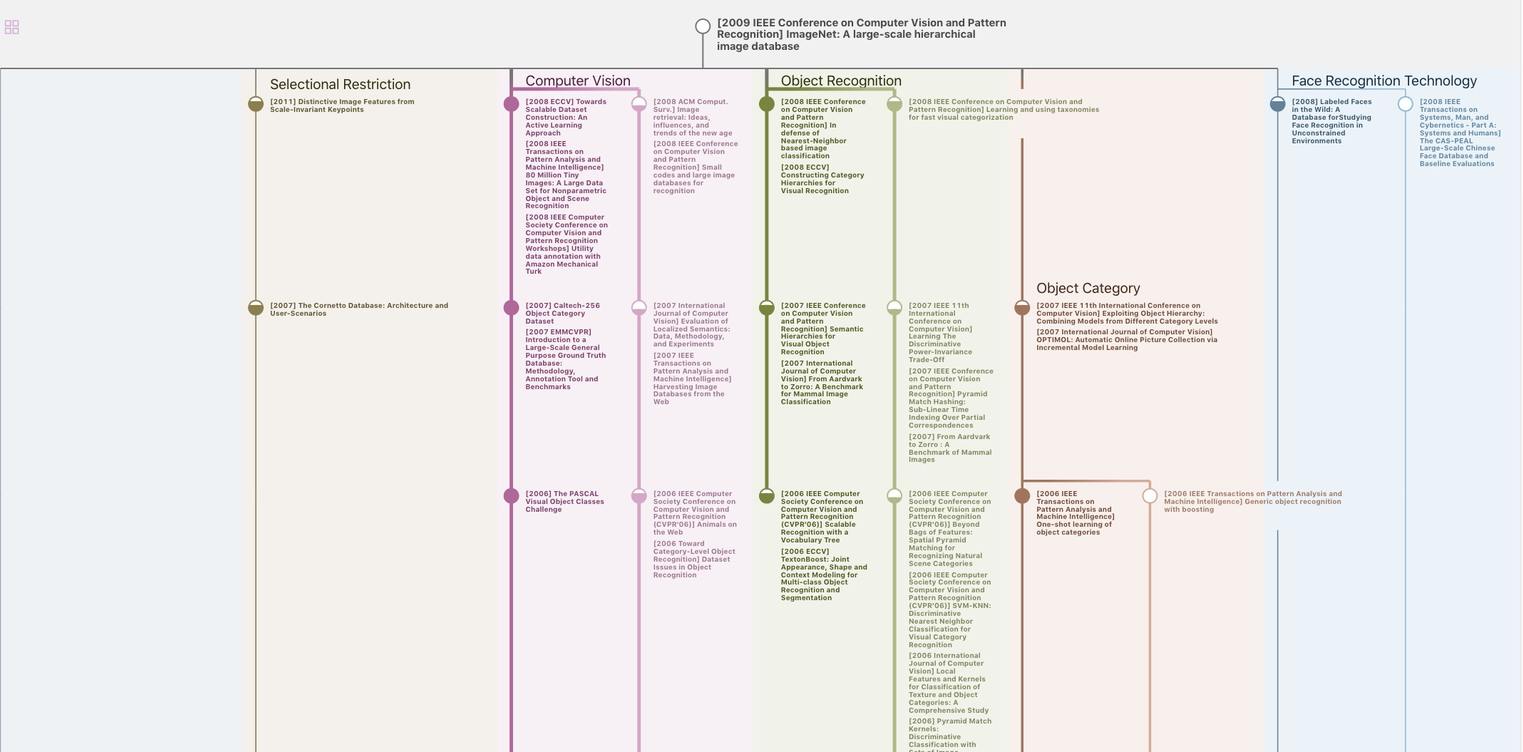
生成溯源树,研究论文发展脉络
Chat Paper
正在生成论文摘要