Braid-DB: Toward AI-Driven Science with Machine Learning Provenance
DRIVING SCIENTIFIC AND ENGINEERING DISCOVERIES THROUGH THE INTEGRATION OF EXPERIMENT, BIG DATA, AND MODELING AND SIMULATION(2022)
摘要
Next-generation scientific instruments will collect data at unprecedented rates: multiple GB/s and exceeding TB/day. Such runs will benefit from automation and steering via machine learning methods, but these methods require new data management and policy techniques. We present here the Braid Provenance Engine (Braid-DB), a system that embraces AI-for-science automation in how and when to analyze and retain data, and when to alter experimental configurations. Traditional provenance systems automate record-keeping so that humans and/or machines can recover how a particular result was obtained and, when failures occur, diagnose causes and enable rapid restart. Related workflow automation efforts need additional recording about model training inputs, including experiments, simulations, and the structures of other learning and analysis activities. Braid-DB combines provenance and version control concepts to provide a robust and usable solution.
更多查看译文
关键词
Provenance, Machine learning, Version control, Database
AI 理解论文
溯源树
样例
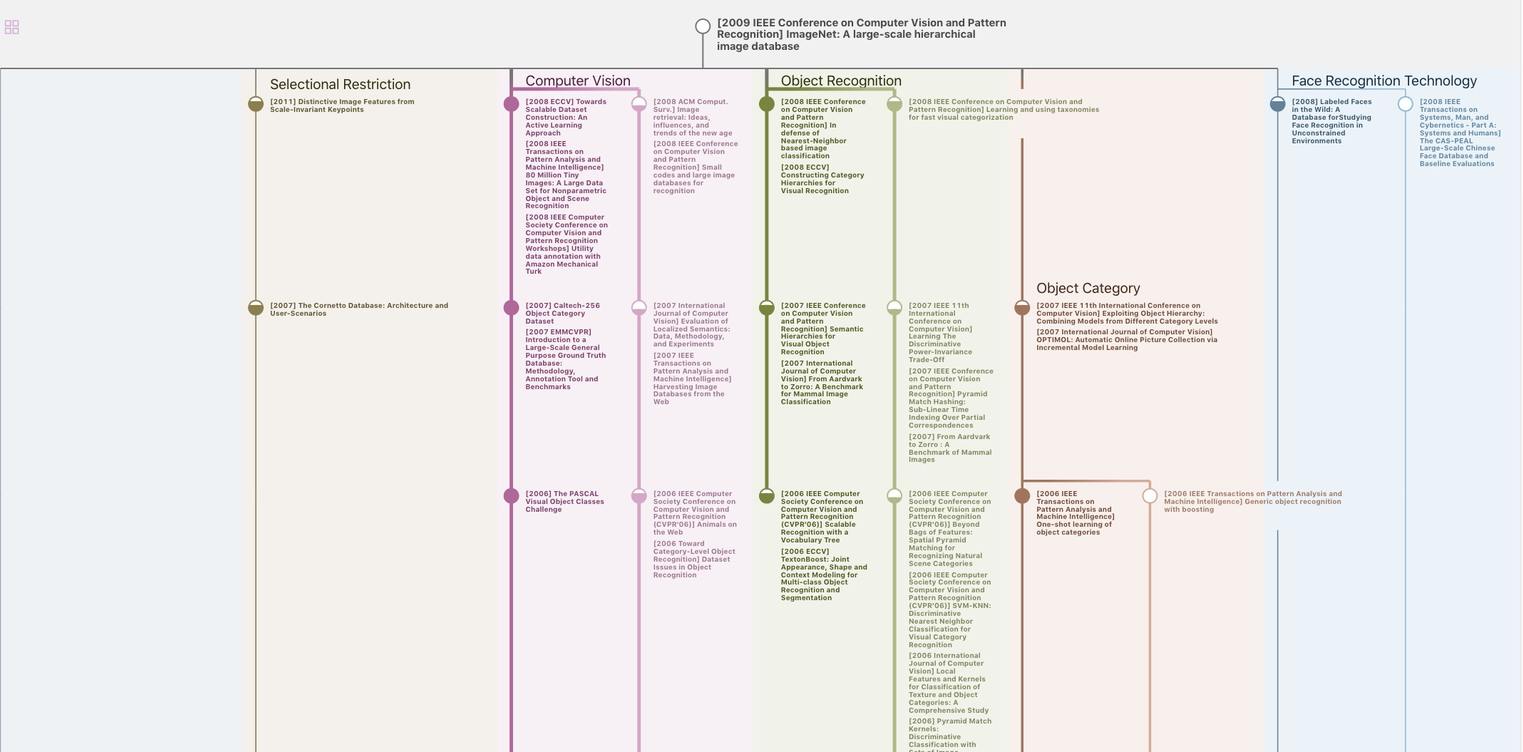
生成溯源树,研究论文发展脉络
Chat Paper
正在生成论文摘要