Few-Shot Generative Learning by Modeling Stereoscopic Priors
PARALLEL AND DISTRIBUTED COMPUTING, APPLICATIONS AND TECHNOLOGIES, PDCAT 2021(2022)
摘要
Few-shot image generation, which aims to generate images from only a few images for a new category, has attracted some research interest in recent years. However, existing few-shot generation methods only focus on 2D images, ignoring 3D information. In this work, we propose a few-shot generative network which leverages 3D priors to improve the diversity and quality of generated images. Inspired by classic graphics rendering pipelines, we unravel the image generation process into three factors: shape, viewpoint and texture. This disentangled representation enables us to make the most of both 3D and 2D information in few-shot generation. To be specific, by changing the viewpoint and extracting textures from different real images, we can generate various new images even in data-scarce settings. Extensive experiments show the effectiveness of our method.
更多查看译文
关键词
Computer vision, Few-shot image generation, Generative adversarial network, Data augmentation
AI 理解论文
溯源树
样例
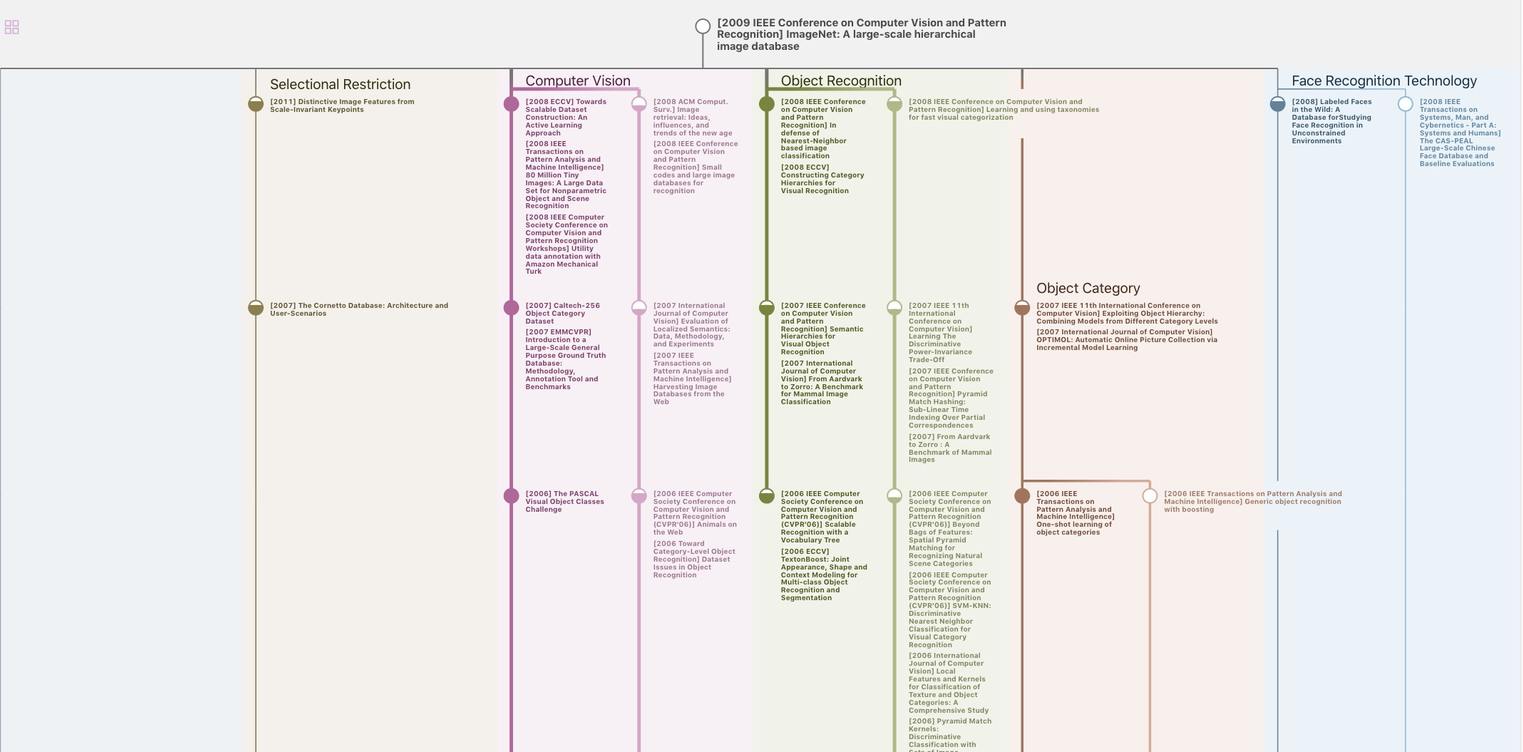
生成溯源树,研究论文发展脉络
Chat Paper
正在生成论文摘要