Fault detection of electrolyzer plate based on improved Mask R-CNN and infrared images
MEASUREMENT SCIENCE AND TECHNOLOGY(2022)
摘要
Non-ferrous metals are very important strategic resources, and electrolysis is an essential step in refining non-ferrous metals. In the electrolysis process, plate short circuit is the most common fault, which seriously affects output and energy consumption. The rapid and accurate detection of faulty plates is of great significance to the metal refining process. Given the weak generalization ability and complex feature rule design of traditional object detection algorithms, and the poor detection effect of existing deep learning models in infrared images with many interference factors, an improved Mask R-CNN-based fault detection algorithm is proposed to improve the generation strategy and non-maximum suppression algorithm of proposals to reduce the missed detection. We also propose a globally generalized intersection over union loss function to characterize better the position and scale relationship between the predicted box and target box, which is beneficial to the bounding box regression. The experimental results show that the improved model has an accuracy rate 10.4% higher than the original model, reaching 86.8%. Compared with the common one- and two-stage object detection models, the improved model has a stronger detection ability. This algorithm has some reference value for the accurate detection and location of electrolytic cell faults.
更多查看译文
关键词
plate fault detection, non-maximum suppression (NMS), Mask R-CNN, G2-IoU loss
AI 理解论文
溯源树
样例
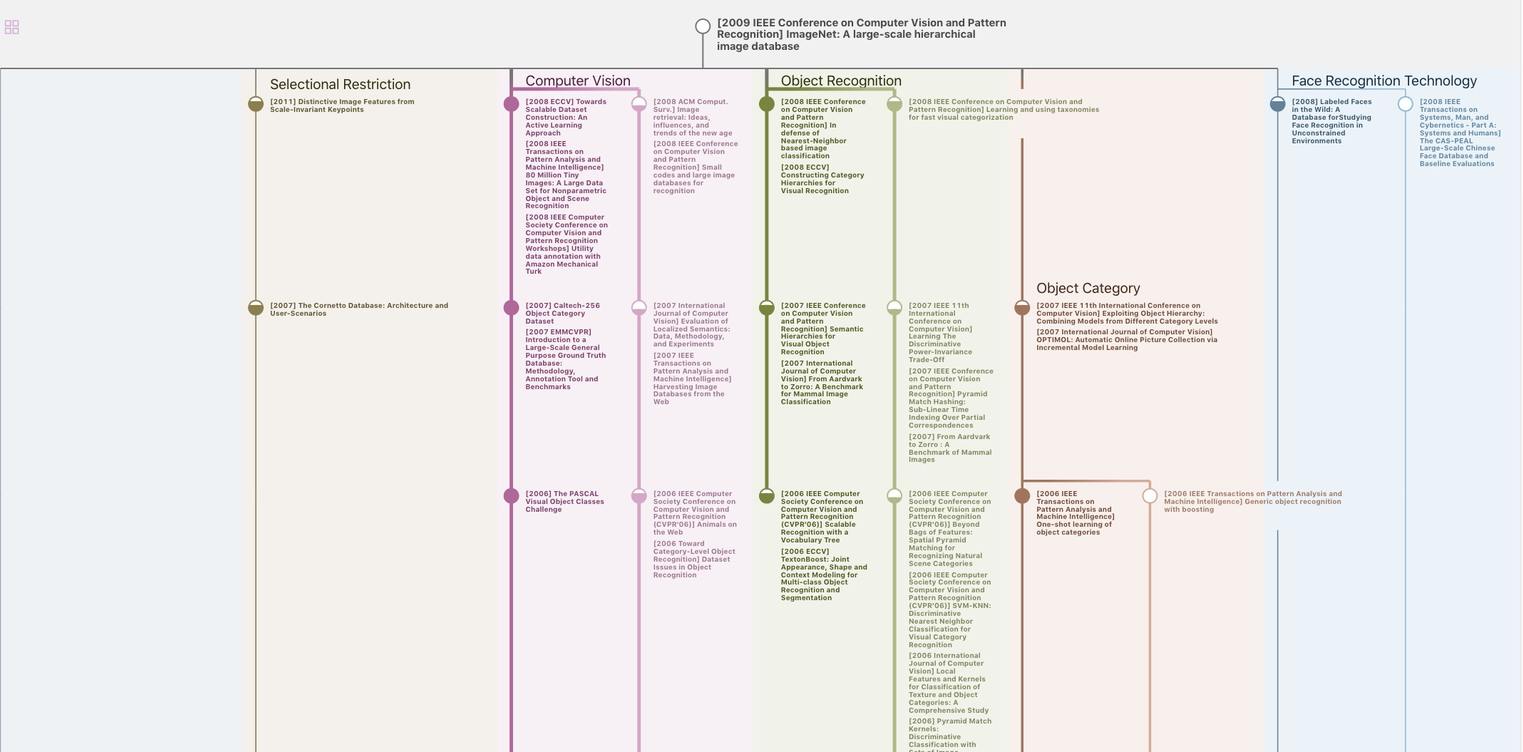
生成溯源树,研究论文发展脉络
Chat Paper
正在生成论文摘要