Bathymetric mapping and estimation of water storage in a shallow lake using a remote sensing inversion method based on machine learning
INTERNATIONAL JOURNAL OF DIGITAL EARTH(2022)
摘要
Accurate lake depth mapping and estimation of changes in water level and water storage are fundamental significance for understanding the lake water resources on the Tibetan Plateau. In this study, combined with satellite images and bathymetric data, we comprehensively evaluate the accuracy of a multi-factor combined linear regression model (MLR) and machine learning models, create a depth distribution map and compare it with the spatial interpolation, and estimate the change of water level and water storage based on the inverted depth. The results indicated that the precision of the random forest (RF) was the highest with a coefficient of determination (R (2)) value (0.9311) and mean absolute error (MAE) values (1.13 m) in the test dataset and had high reliability in the overall depth distribution. The water level increased by 9.36 m at a rate of 0.47 m/y, and the water storage increased by 1.811 km(3) from 1998 to 2018 based on inversion depth. The water level change was consistent with that of the Shuttle Radar Topography Mission (SRTM) method. Our work shows that this method may be employed to study the water depth distribution and its changes by combining with bathymetric data and satellite imagery in shallow lakes.
更多查看译文
关键词
Remote sensing inversion, lake bathymetry, Sentinel-2, machine learning (ML), random forest (RF), water storage
AI 理解论文
溯源树
样例
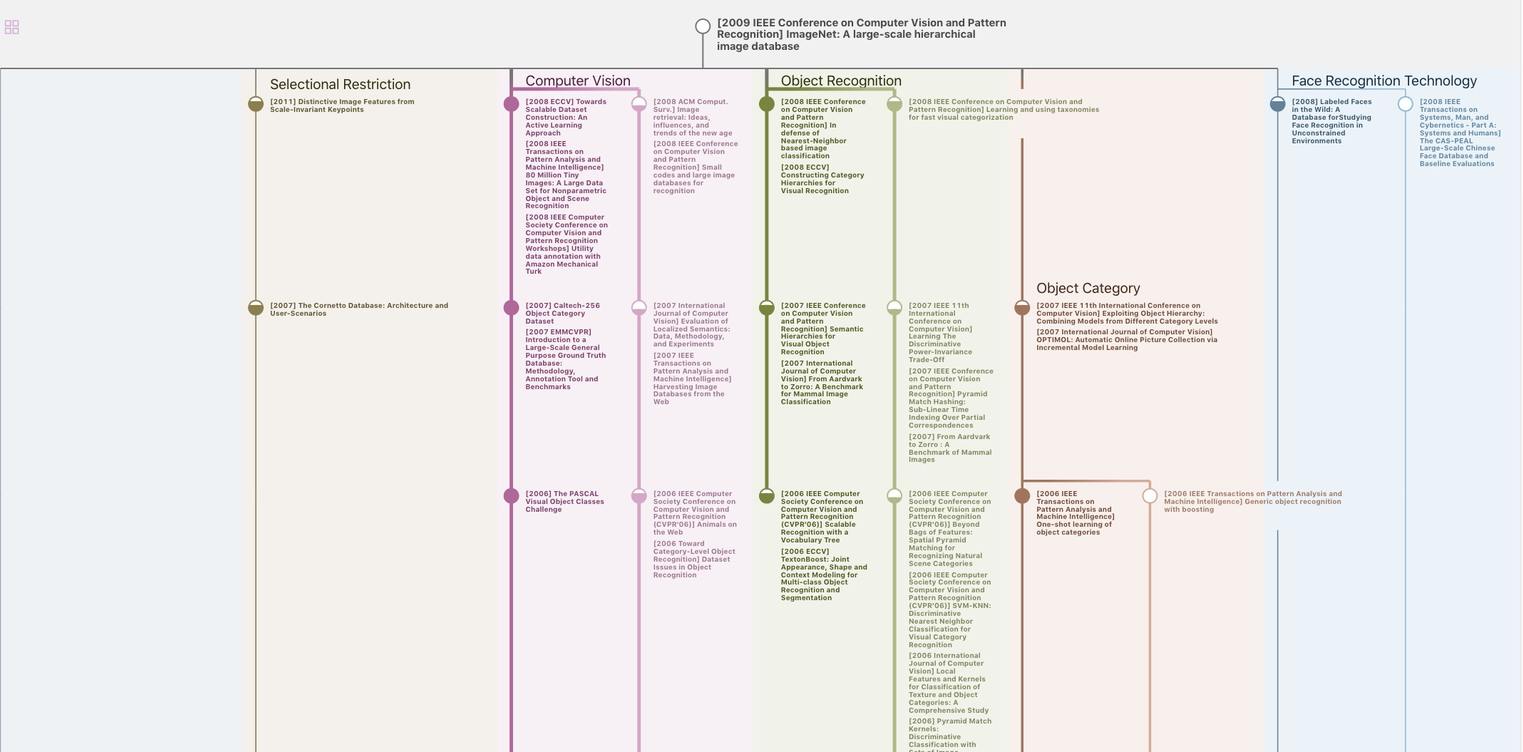
生成溯源树,研究论文发展脉络
Chat Paper
正在生成论文摘要