A Multi-Scale Multi-Task Learning Model for Continuous Dimensional Emotion Recognition from Audio
ELECTRONICS(2022)
摘要
Due to the advantages of many aspects of the dimensional emotion model, continuous dimensional emotion recognition from audio has attracted increasing attention in recent years. Features and dimensional emotion labels on different time scales have different characteristics and contain different information. To make full use of the advantages of features and emotion representations from multiple time scales, a novel multi-scale multi-task (MSMT) learning model is proposed in this paper. The MSMT model is constructed by a deep belief network (DBN) with only one hidden layer. The same hidden layer parameters and linear layer parameters are shared by all features. Multiple temporal pooling operations are inserted between the hidden layer and the linear layer to obtain information on multiple time scales. The mean squared error (MSE) of the main and the secondary task are combined to form the final objective function. Extensive experiments were conducted on RECOLA and SEMAINE datasets to illustrate the effectiveness of our model. The results for the two sets show that even adding a secondary scale to the scale with optimal single-scale single-task performance can achieve significant performance improvements.
更多查看译文
关键词
continuous dimensional emotion recognition, multi-task learning, deep belief network
AI 理解论文
溯源树
样例
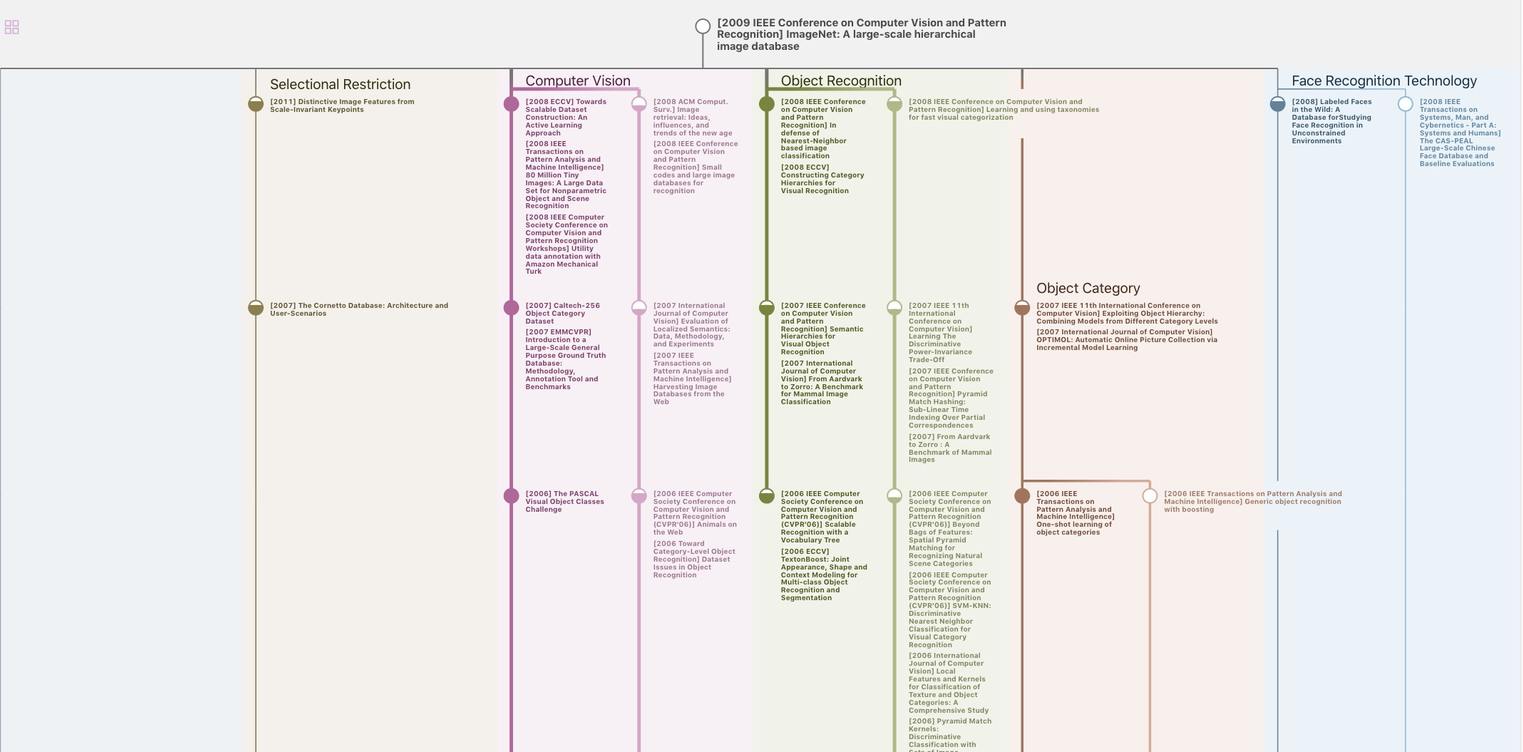
生成溯源树,研究论文发展脉络
Chat Paper
正在生成论文摘要