End-to-End Deep Learning CT Image Reconstruction for Metal Artifact Reduction
APPLIED SCIENCES-BASEL(2022)
摘要
Metal artifacts are common in CT-guided interventions due to the presence of metallic instruments. These artifacts often obscure clinically relevant structures, which can complicate the intervention. In this work, we present a deep learning CT reconstruction called iCTU-Net for the reduction of metal artifacts. The network emulates the filtering and back projection steps of the classical filtered back projection (FBP). A U-Net is used as post-processing to refine the back projected image. The reconstruction is trained end-to-end, i.e., the inputs of the iCTU-Net are sinograms and the outputs are reconstructed images. The network does not require a predefined back projection operator or the exact X-ray beam geometry. Supervised training is performed on simulated interventional data of the abdomen. For projection data exhibiting severe artifacts, the iCTU-Net achieved reconstructions with SSIM = 0.970 & PLUSMN;0.009 and PSNR = 40.7 & PLUSMN;1.6. The best reference method, an image based post-processing network, only achieved SSIM = 0.944 & PLUSMN;0.024 and PSNR = 39.8 & PLUSMN;1.9. Since the whole reconstruction process is learned, the network was able to fully utilize the raw data, which benefited from the removal of metal artifacts. The proposed method was the only studied method that could eliminate the metal streak artifacts.
更多查看译文
关键词
image reconstruction, deep learning, metal artifacts, computed tomography
AI 理解论文
溯源树
样例
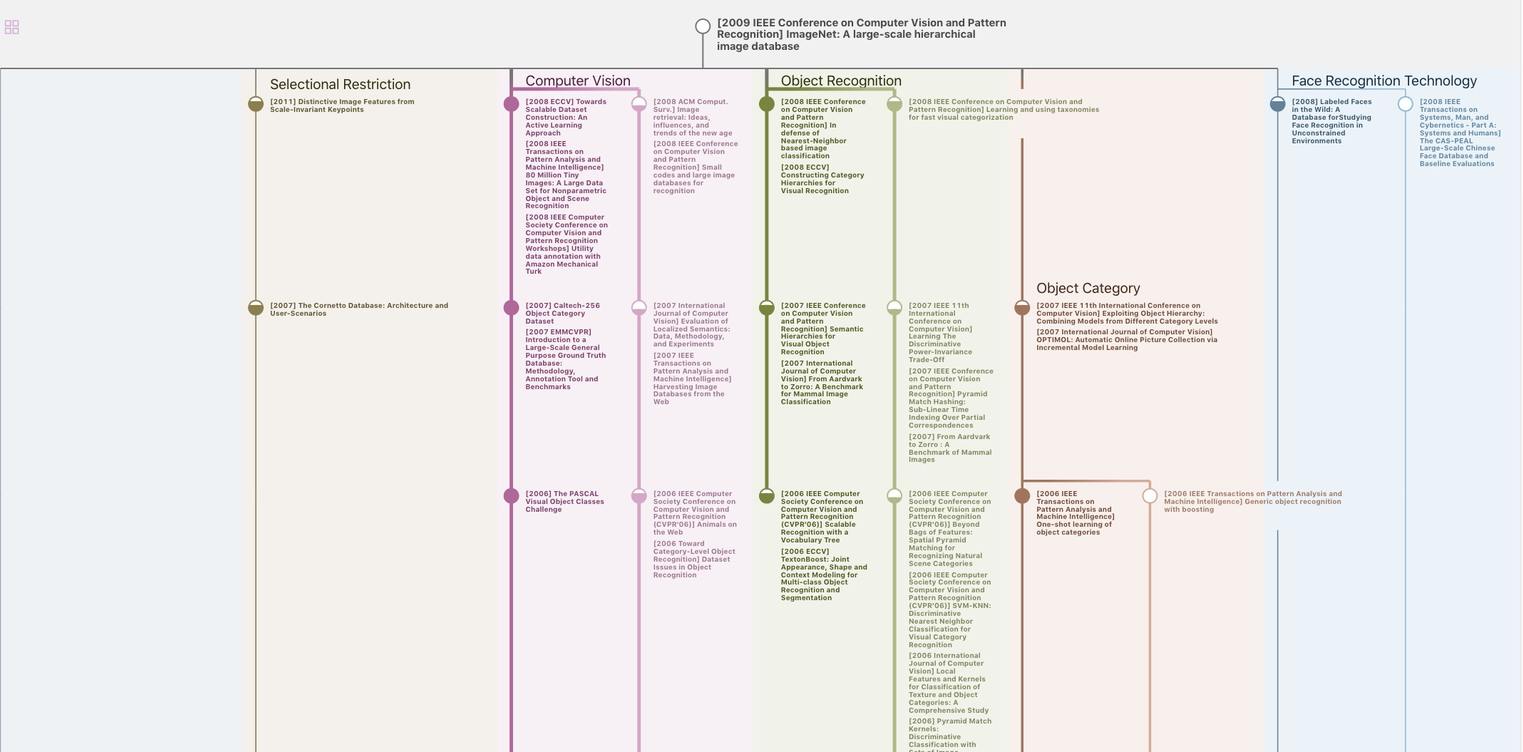
生成溯源树,研究论文发展脉络
Chat Paper
正在生成论文摘要