Beyond Coarray MUSIC: Harnessing the Difference Sets of Nested Arrays With Limited Snapshots
IEEE SIGNAL PROCESSING LETTERS(2021)
摘要
We proposea new framework for leveraging the degrees of freedom in the difference set of a nested array with limited snapshots. Typically, this difference set (or coarray) is realized by first estimating a virtual coarray covariance matrix from the sample covariance matrix. However, with only a few snapshots, these techniques incur large estimation error, which saturates away from zero even as the signal-to-noise ratio (SNR) tends to infinity. We address this issue by moving away from estimating the coarray covariance matrix when snapshots are limited, and instead proposing a "proxy covariance matrix" (Prox-Cov) that provides an alternate estimate of the coarray subspace (but does not attempt to estimate the source powers). (Prox-Cov) is shown to outperform coarray MUSIC with limited snapshots when the number of sources exceeds the number of sensors. Moreover, when the number of sources is fewer than sensors, we prove that (Prox-Cov) leads to exact identification of the coarray subspace with very few snapshots in the absence of noise, while the error of coarray MUSIC provably saturates in this regime.
更多查看译文
关键词
Multiple signal classification, Covariance matrices, Sensor arrays, Signal to noise ratio, Signal processing algorithms, Sensor phenomena and characterization, Direction-of-arrival estimation, Sparse arrays, nested sampling, super-resolution, toeplitz covariance estimation, direction-of-arrival estimation
AI 理解论文
溯源树
样例
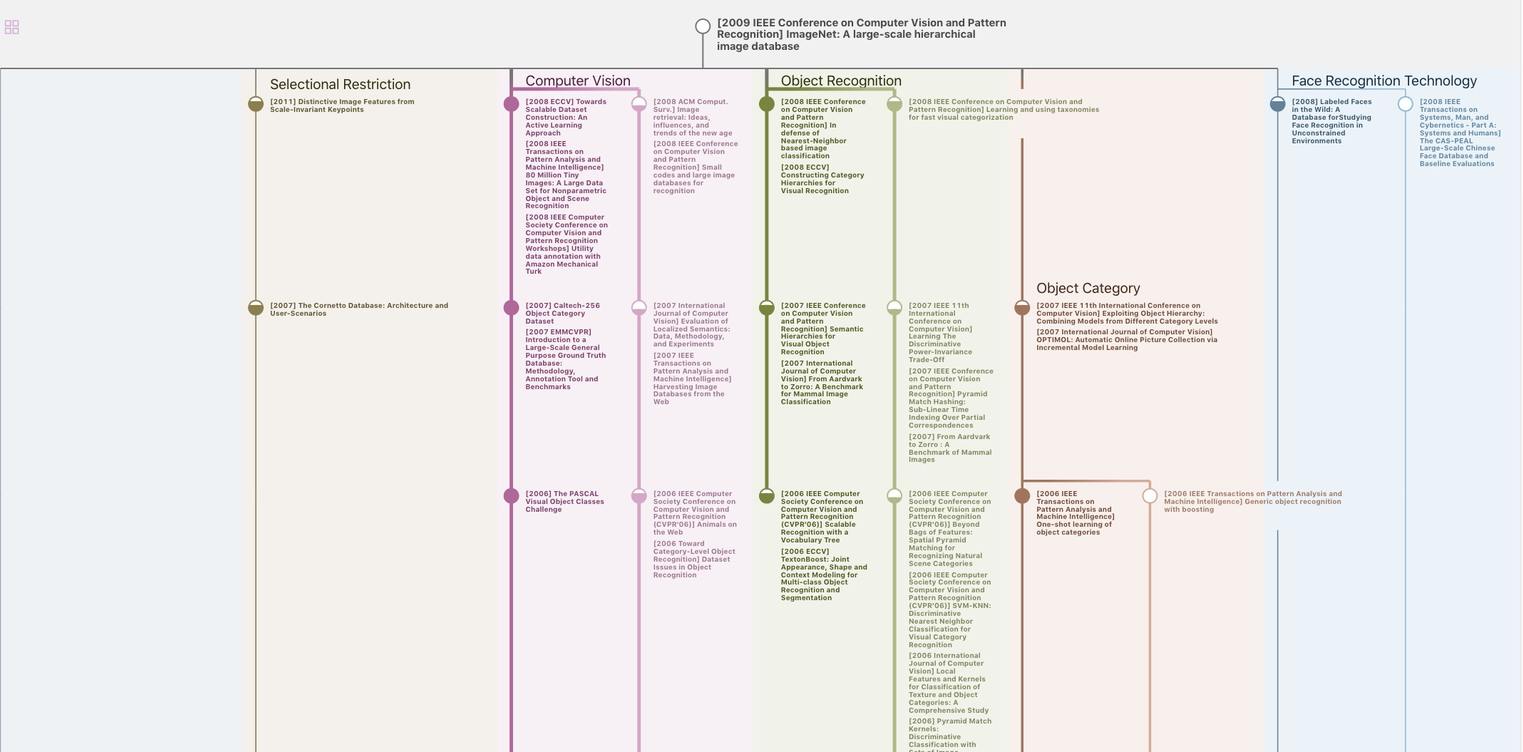
生成溯源树,研究论文发展脉络
Chat Paper
正在生成论文摘要