Machine learning aids clinical decision making in patients presenting with angina and non-obstructive coronary artery disease
EUROPEAN HEART JOURNAL(2021)
摘要
Abstract Background Coronary microvascular dysfunction (CMD) is associated with an increased risk of cardiovascular morbidity and mortality. The current gold-standard comprehensive assessment of CMD is through a limited-access invasive catheterization lab procedure. Purposes We aimed to develop a point-of-care tool to assist clinical guidance in patients presenting with chest pain and/or an abnormal functional stress test and with non-obstructive coronary artery disease (NOCAD) based on demographic data and resting ECG waveforms. Methods This study included 1,893 NOCAD patients (<50% angiographic stenosis) who underwent CMD evaluation as well as a standard ECG up to 1 year prior. Microvascular endothelial-independent dysfunction was defined by abnormal coronary flow reserve (CFR) <2.5 in response to intracoronary adenosine administration. Microvascular endothelial dysfunction was defined by a maximal percent increase in coronary blood flow (%ΔCBF) ≤50% in response to intracoronary acetylcholine infusion. We trained algorithms to distinguish between the following outcomes: CFR ≤2.5, ΔCBF (%) ≤50, and the combination of both. Two classes of algorithms were trained, one depending on ECG waveforms as input, and another using tabular style clinical data. The optimal classification threshold was determined by maximizing Youden's J. Results Mean age was 51±12 years and 66% were females (n=1,257). AUC values ranged from 0.49 to 0.67 for all the outcomes. The best performance in our analysis was for the outcome CFR ≤2.5 via logistic regression on tabular variables. AUC and accuracy were 0.67 and 60%; while sensitivity and NPV were 70% and 85%. Specificity and PPV were 56% and 0.35%. When decreasing the threshold from the “optimal” one (24%) to 15%, sensitivity and NPV increased to 92% and 90% respectively, while specificity and PPV decreased to 25% and 29% respectively (Figure 2). Conclusion An AI-enabled algorithm may be able to assist clinical guidance by ruling out CMD in patients presenting with chest pain and/or an abnormal functional stress test. This algorithm needs to be prospectively validated in different cohorts. Funding Acknowledgement Type of funding sources: Private grant(s) and/or Sponsorship. Main funding source(s): The James Nutter Family & Maria Long Family Fellowship in Cardiovascular Research and Mayo Clinic Figure 1Figure 2
更多查看译文
关键词
coronary artery disease,clinical decision making,angina,machine learning,non-obstructive
AI 理解论文
溯源树
样例
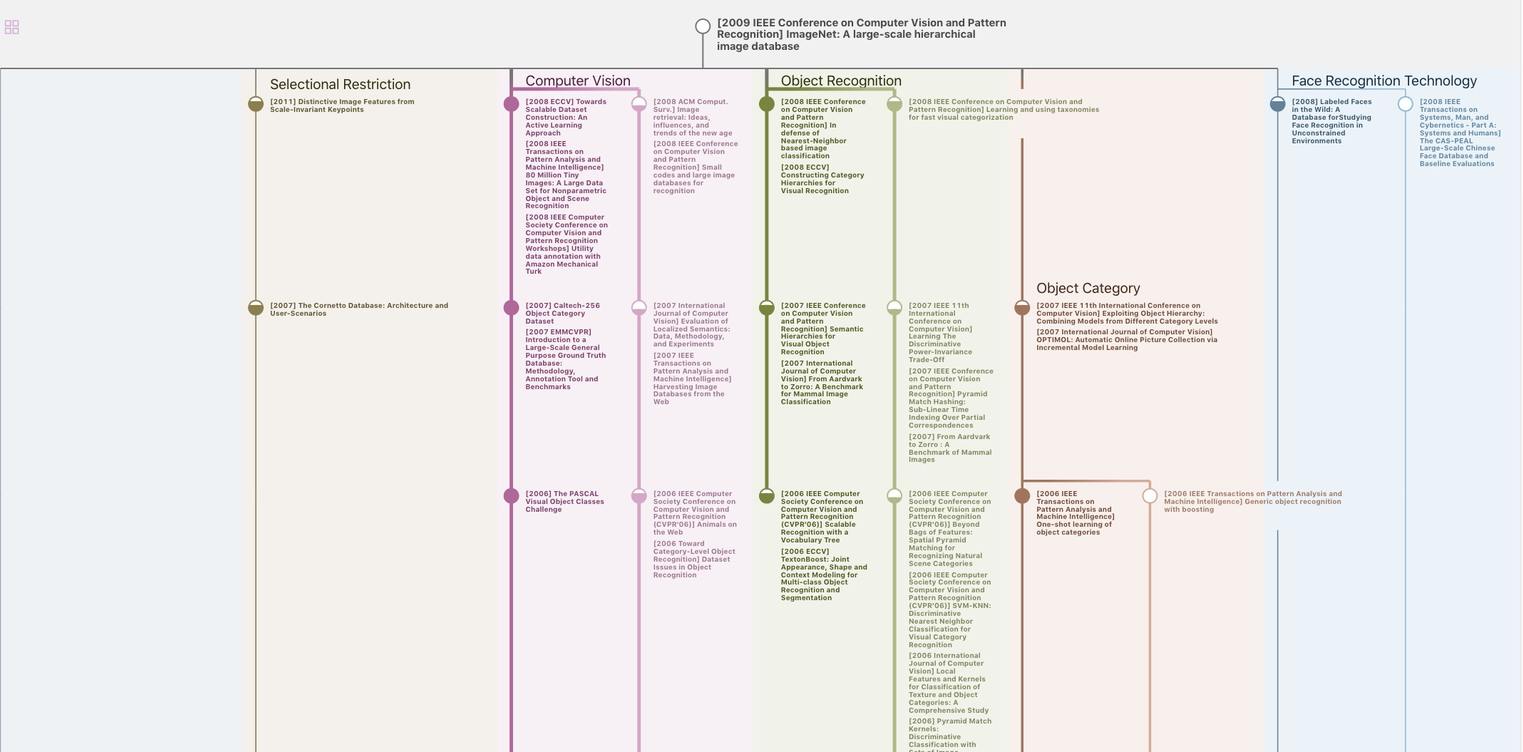
生成溯源树,研究论文发展脉络
Chat Paper
正在生成论文摘要