Long Short-Term Memory Based Subsurface Drainage Control for Rainfall-Induced Landslide Prevention
GEOSCIENCES(2022)
摘要
Subsurface drainage has been widely accepted to mitigate the hazard of landslides in areas prone to flooding. Specifically, the use of drainage wells with pumping systems has been recognized as an effective short-term solution to lower the groundwater table. However, this method has not been well considered for long-term purposes due to potentially high labor costs. This study aims to investigate the idea of an autonomous pumping system for subsurface drainage by leveraging conventional geotechnical engineering solutions and a deep learning technique-Long-Short Term Memory (LSTM)-to establish a geotechnical cyber-physical system for rainfall-induced landslide prevention. For this purpose, a typical soil slope equipped with three pumps was considered in a computer simulation. Forty-eight cases of rainfall events with a wide range of varieties in duration, total rainfall depths, and different rainfall patterns were generated. For each rainfall event, transient seepage analysis was performed using newly proposed Python code to obtain the corresponding pump's flow rate data. A policy of water pumping for maintaining groundwater at a desired level was assigned to the pumps to generate the data. The LSTM takes rainfall event data as the input and predicts the required pump's flow rate. The results from the trained model were validated using evaluation metrics of root mean square error (RMSE), mean absolute error (MAE), and R-2. The R-2-scores of 0.958, 0.962, and 0.954 for the predicted flow rates of the three pumps exhibited high accuracy of the predictions using the trained LSTM model. This study is intended to make a pioneering step toward reaching an autonomous pumping system and lowering the operational costs in controlling geosystems.
更多查看译文
关键词
landslide prevention, disaster resilience, geotechnical cyber-physical systems, long short-term memory, deep learning, transient flow
AI 理解论文
溯源树
样例
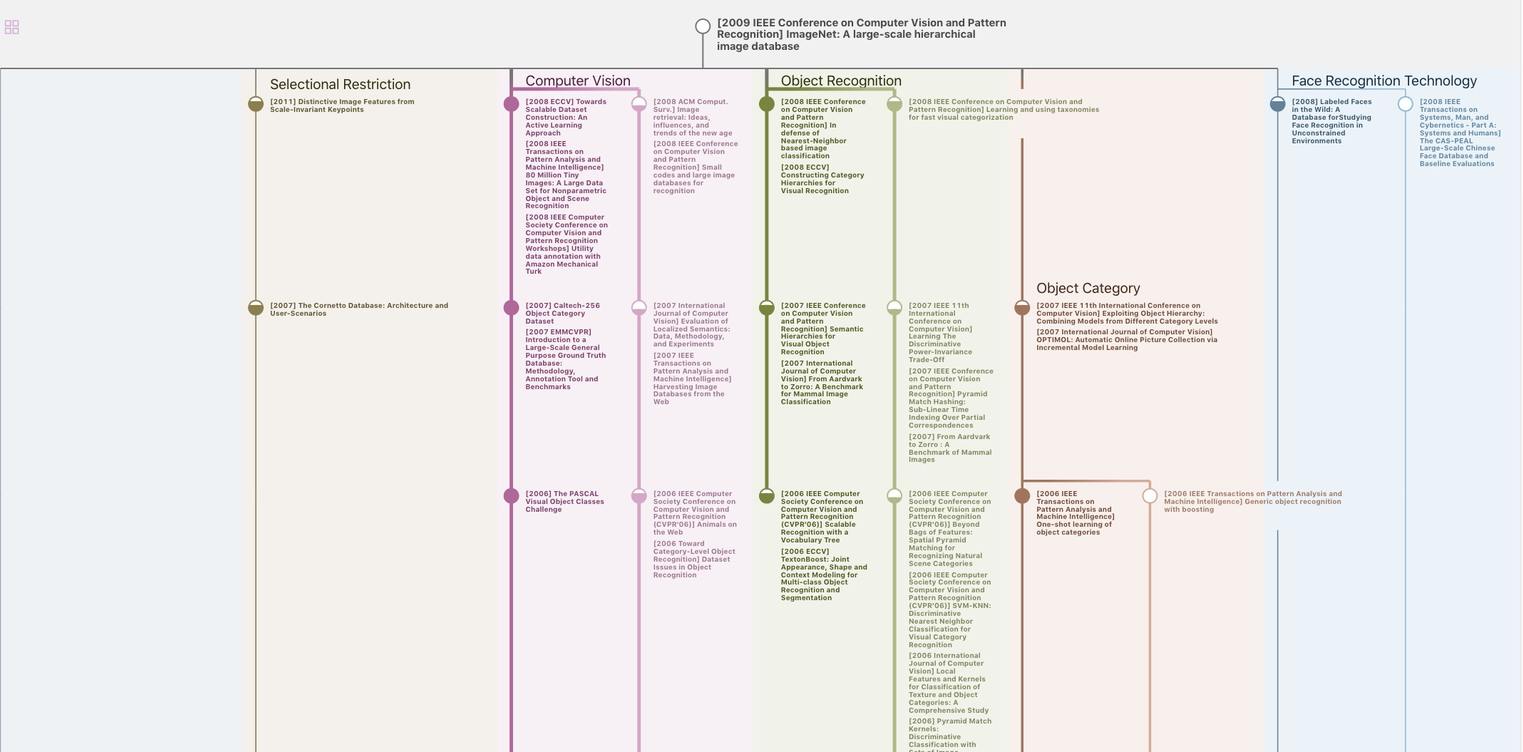
生成溯源树,研究论文发展脉络
Chat Paper
正在生成论文摘要