No such thing as the perfect match: Bayesian Model Averaging for treatment evaluation
ECONOMIC MODELLING(2022)
摘要
Propensity Score Matching is a popular approach to evaluate treatment effects in observational studies. Regrettably, practitioners often overlook the issue of model uncertainty and its consequences when building the propensity score model. We tackle this problem by Bayesian Model Averaging (BMA) with an application to the 2014 Italian tax credit reform (the so-called "Renzi bonus"). Model uncertainty has a dramatic impact on the estimated treatment effects: using standard model selection procedures may lead to choosing equally defensible models that, however, produce substantially heterogeneous results. By using BMA-based estimates, we find a much more coherent picture: significant effect of the rebate on food consumption only for liquidity constrained households, in line with most recent literature.
更多查看译文
关键词
2014 Italian tax credit reform, Bayesian model averaging, Model uncertainty, Propensity score matching, Reversible jump Markov chain Monte Carlo, Tax rebate policies
AI 理解论文
溯源树
样例
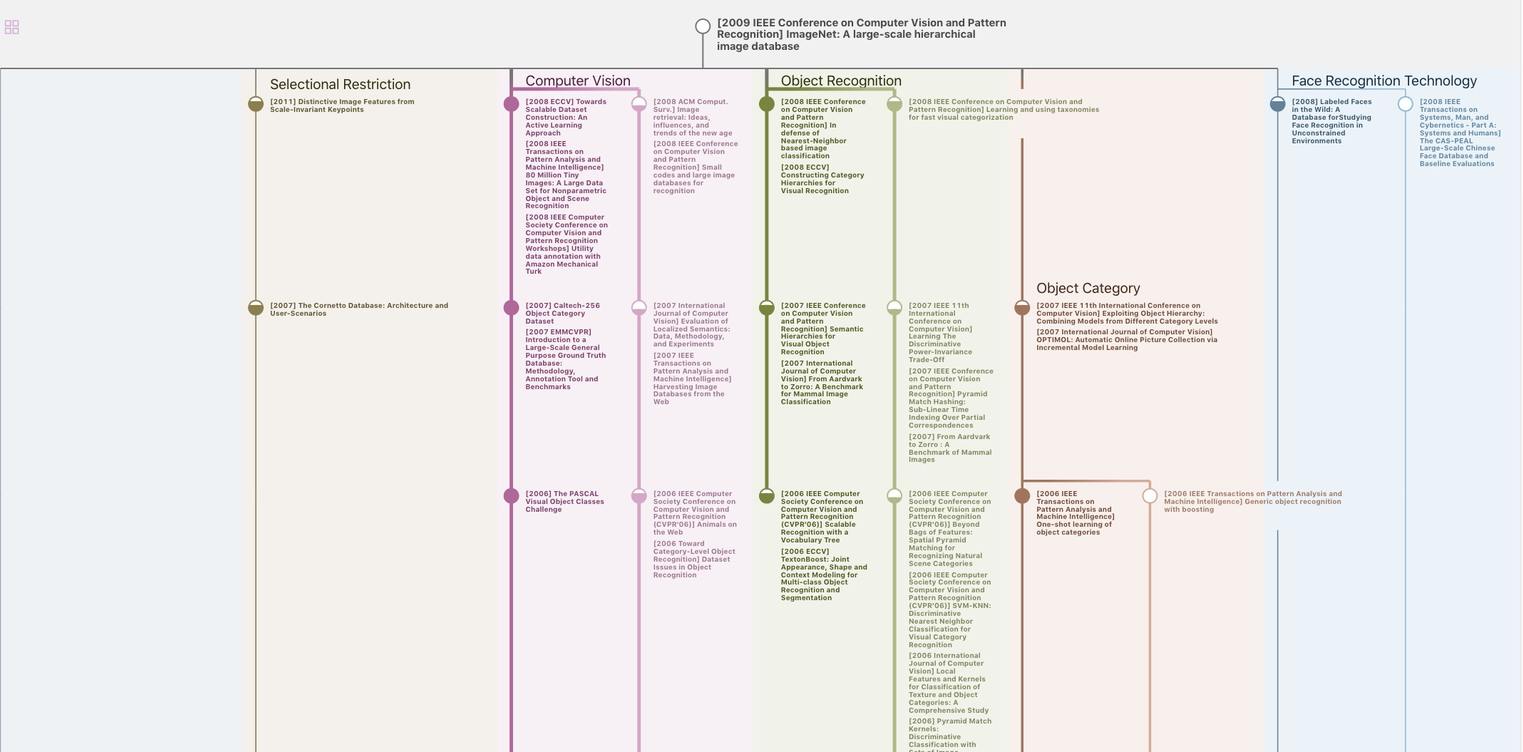
生成溯源树,研究论文发展脉络
Chat Paper
正在生成论文摘要