Inconstant Update of Reference Point Value for Parallel and Distributed MOEA/D
2021 IEEE CONGRESS ON EVOLUTIONARY COMPUTATION (CEC 2021)(2021)
摘要
This paper proposes an update method to determine the reference point and nadir point when speeding up multi-objective evolutionary optimization algorithm based on decomposition (MOEA/D) with parallel and distributed processing in a many-core environment. We have already proposed a migration method that uses a virtual overlapping zone between partitions. Compared with simply partitioning the objective space or applying the standard island migration model, this method is shown to be effective in improving the hypervolume (HV) value, increasing diversity, and reducing regions where non-dominated solutions do not exist between partitions (sparse areas). However, this proposed method could not completely deal with the sparse areas' problem in the early stage of solution searching. To resolve this problem, in addition to the virtual overlap method, we propose here a method that switches the update interval for determining the reference point and nadir point by taking into account the progress of solution searching. To evaluate our newly proposed method, we compare both convergence and diversity on single-core MOEA/D and parallel MOEA/D using a two-objective constrained knapsack problem, and show that our method is effective not only in reducing sparse areas in the early stage but also in improving diversity and increasing the HV value.
更多查看译文
关键词
MOEA/D,parallel and distributed processing,reference point,multi-objective evolutionary algorithms
AI 理解论文
溯源树
样例
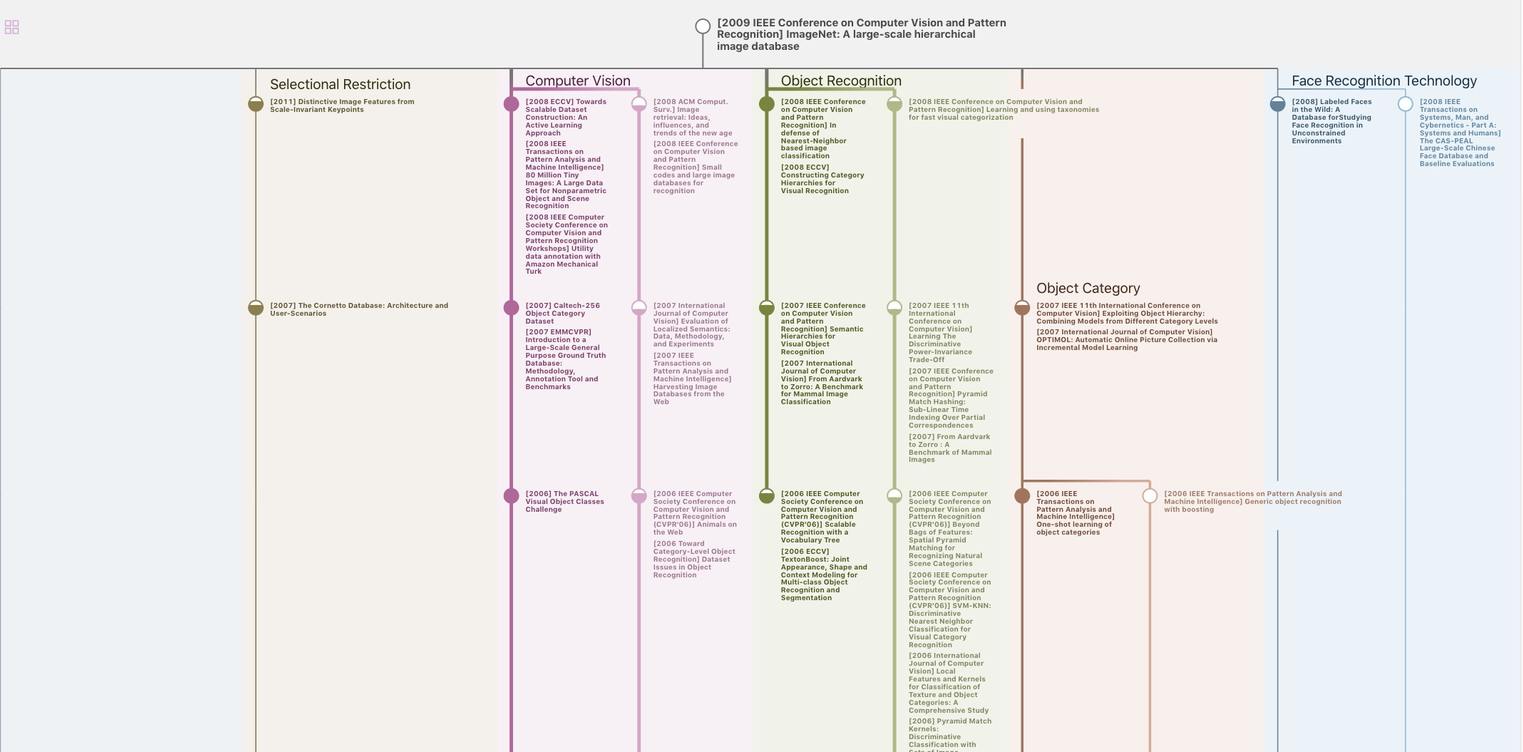
生成溯源树,研究论文发展脉络
Chat Paper
正在生成论文摘要