Privacy-preserving federated learning framework in multimedia courses recommendation
WIRELESS NETWORKS(2022)
摘要
The recommendation of multimedia courses has been identified as a potential area of growth for online education. Several of the drawbacks of existing multimedia course recommendation systems are listed below. To begin, they will generate problems if the user enrolls in several courses across many records. Second, centralizing user activity data storage poses the risk of data leakage. We provide a framework for federated recommendation model learning to address the aforementioned concerns. We employ an attention-based hierarchical reinforcement learning model to determine which parts of user data are crucial to target in the proposed method. To protect privacy, we aggregate models and coordinate round demarcation using a federated learning system. This is performed through local model training on the user device and global model parameter computation on the central server. More precisely, we retain a model copy of the recommendation on each user device and then update the global model using a group of randomly selected aggregated local factors. Additionally, we did an experiment utilizing the XuetangX dataset, which contains authentic multimedia courses. As proved by numerical findings, our federated system operates effectively in a privacy-preserving mode.
更多查看译文
关键词
Multimedia,Courses recommendation,Federated learning
AI 理解论文
溯源树
样例
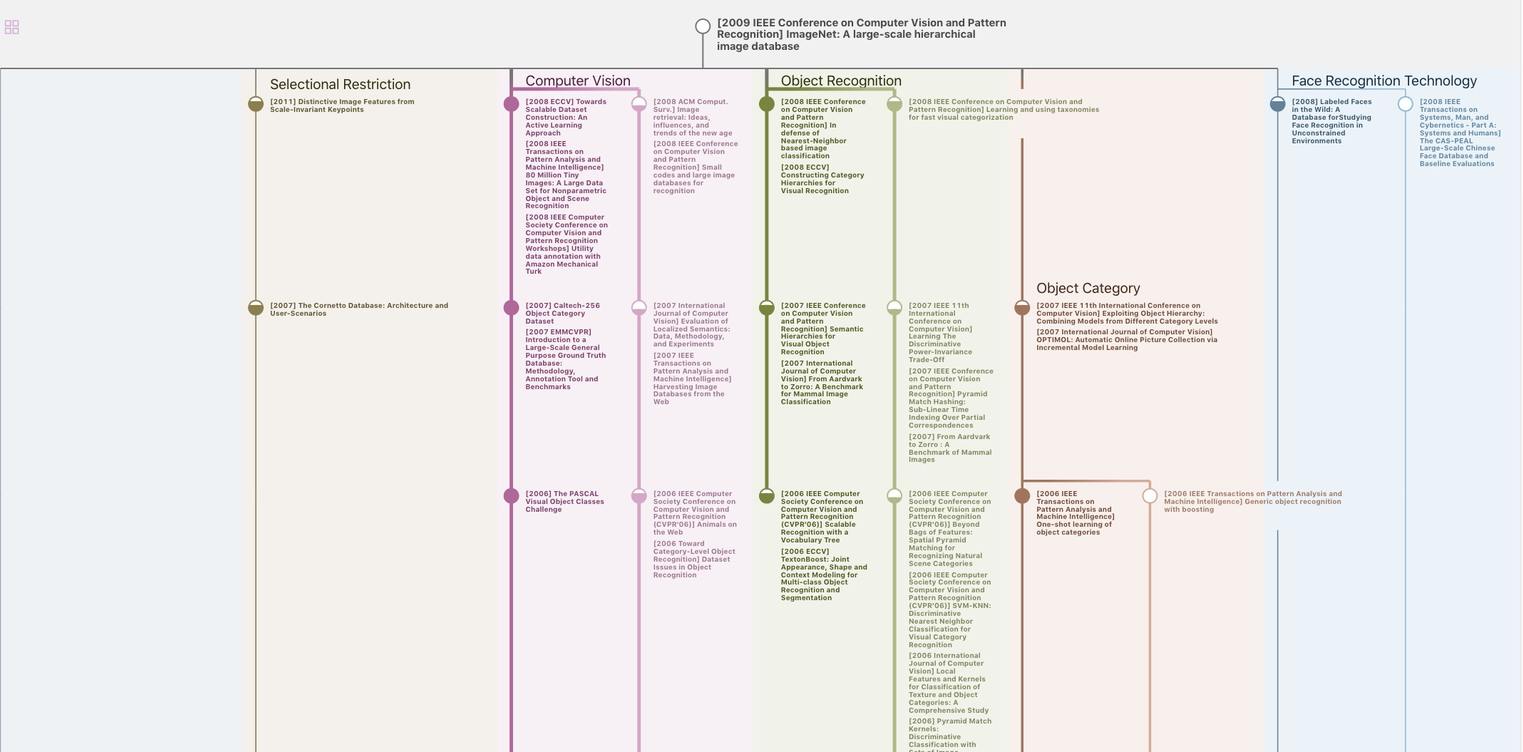
生成溯源树,研究论文发展脉络
Chat Paper
正在生成论文摘要