Mu-Net: Multi-Path Upsampling Convolution Network for Medical Image Segmentation
CMES-COMPUTER MODELING IN ENGINEERING & SCIENCES(2022)
摘要
Medical image segmentation plays an important role in clinical diagnosis, quantitative analysis, and treatment process. Since 2015, U-Net-based approaches have been widely used for medical image segmentation. The purpose of the U-Net expansive path is to map low-resolution encoder feature maps to full input resolution feature maps. However, the consecutive deconvolution and convolutional operations in the expansive path lead to the loss of some high-level information. More high-level information can make the segmentation more accurate. In this paper, we propose MU-Net, a novel, multi-path upsampling convolution network to retain more high-level information. The MU-Net mainly consists of three parts: contracting path, skip connection, and multi-expansive paths. The proposed MU-Net architecture is evaluated based on three different medical imaging datasets. Our experiments show that MU-Net improves the segmentation performance of U-Net-based methods on different datasets. At the same time, the computational efficiency is significantly improved by reducing the number of parameters by more than half.
更多查看译文
关键词
Medical image segmentation, MU-Net (multi-path upsampling convolution network), U-Net, clinical diagno-sis, encoder-decoder networks
AI 理解论文
溯源树
样例
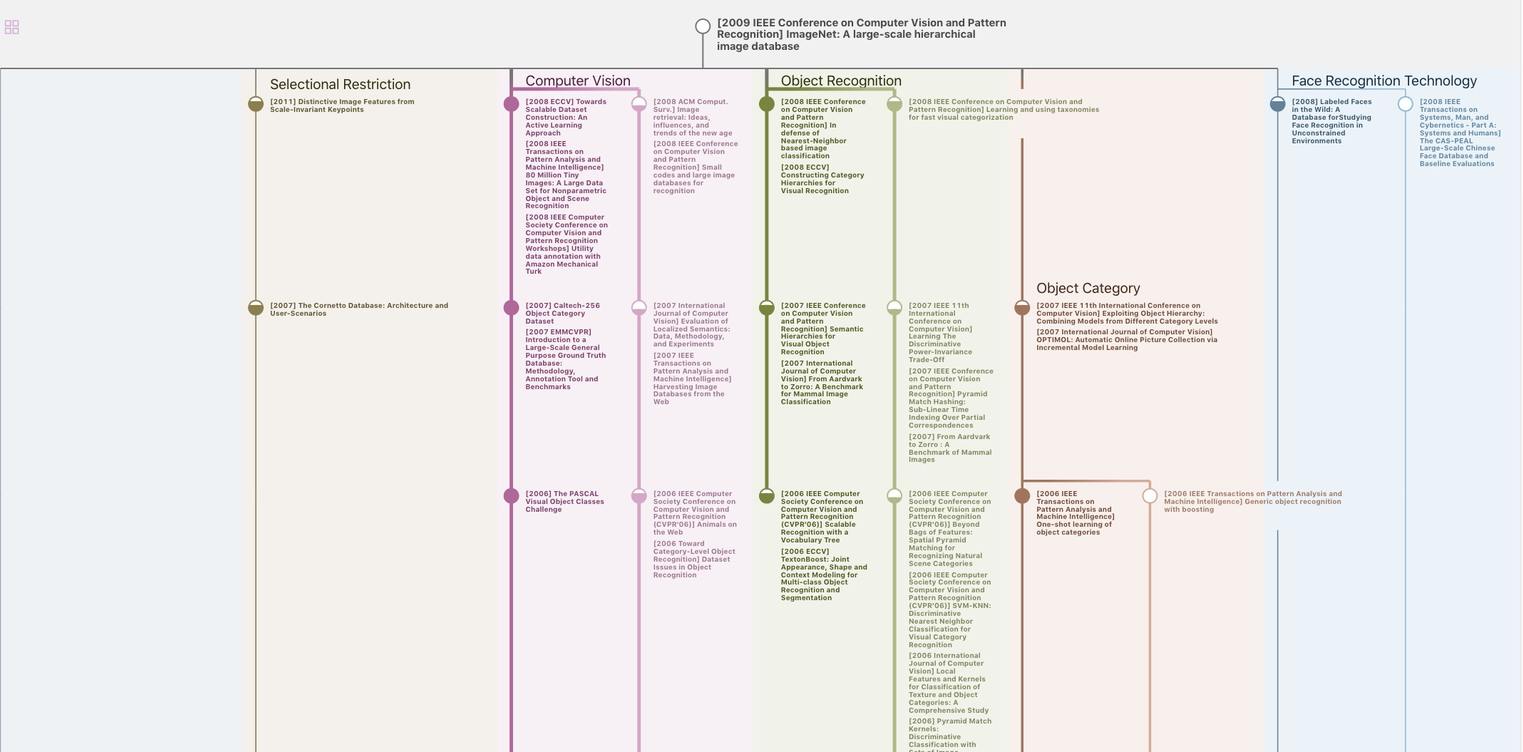
生成溯源树,研究论文发展脉络
Chat Paper
正在生成论文摘要