DeepFlood: A deep learning based flood detection framework using feature-level fusion of multi-sensor remote sensing images
JOURNAL OF UNIVERSAL COMPUTER SCIENCE(2022)
摘要
Flooding is the most common natural disaster in many countries. Remote sensing images are very much useful in disaster monitoring. The different image modalities from different satellites provide varied information about the earth. The synergistic use of optical and radar data helps in precise flood detection. The central focus of this paper is to identify the flooded regions using a dual patch-based Fully Convolutional Network (FCN) for performing deep learning-based feature fusion. The learned features of FCNs trained independently with Synthetic Aperture Radar (SAR) and Multispectral (MS) images are concatenated to represent the flooding better. A random forest classifier is employed to identify the flood from the fused features. The information retrieved is very much valuable in undertaking necessary rescue efforts in flood-affected areas. The proposed network shows superior performance in flood detection on the images from the SEN12-FLOOD dataset with an accuracy as high as 94.17%.
更多查看译文
关键词
flood detection,  , synthetic aperture radar,  , multispectral,  , convolutional neural network,  , deep learning
AI 理解论文
溯源树
样例
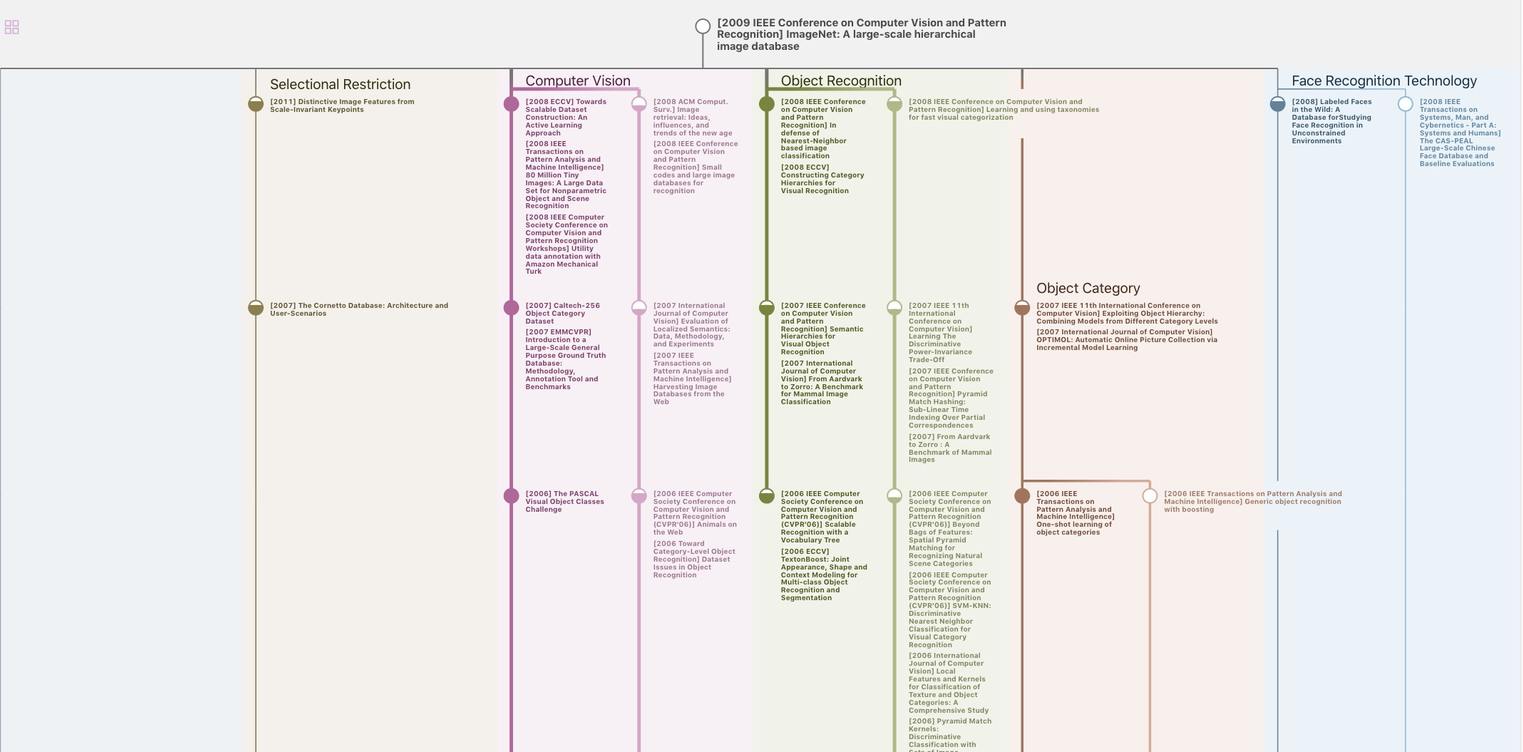
生成溯源树,研究论文发展脉络
Chat Paper
正在生成论文摘要