Multimodal 2.5D Convolutional Neural Network for Diagnosis of Alzheimer's Disease with Magnetic Resonance Imaging and Positron Emission Tomography
PROGRESS IN ELECTROMAGNETICS RESEARCH-PIER(2021)
摘要
Alzheimer's disease (AD) is a degenerative disease of the nervous system that often occurs inthe elderly. As magnetic resonance imaging (MRI) and positron emission tomography (PET) re ect thebrain's anatomical changes and functional changes caused by AD, they are often used to diagnose AD.Multimodal fusion based on these two types of images can effectively utilize complementary informationand improve diagnostic performance. To avoid the computational complexity of the 3D image andexpand training samples, this study designed an AD diagnosis framework based on a 2.5D convolutionalneural network (CNN) to fuse multimodal data. First, MRI and PET were preprocessed with skullstripping and registration. After that, multiple 2.5D patches were extracted within the hippocampusregions from both MRI and PET. Then, we constructed a multimodal 2.5D CNN to integrate themultimodal information from MRI and PET patches. We also utilized a training strategy called branchespre-training to enhance the feature extraction ability of the 2.5D CNN by pre-training two brancheswith corresponding modalities individually. Finally, the results of patches are used to diagnose AD andprogressive mild cognitive impairment (pMCI) patients from normal controls (NC). The experimentswere conducted with the ADNI dataset, and accuracies of 92.89% and 84.07% were achieved in the ADvs. NC and pMCI vs. NC tasks. The results are much better than using single modality and indicatethat the proposed multimodal 2.5D CNN could effectively integrate complementary information frommulti-modality and yield a promising AD diagnosis performance.
更多查看译文
AI 理解论文
溯源树
样例
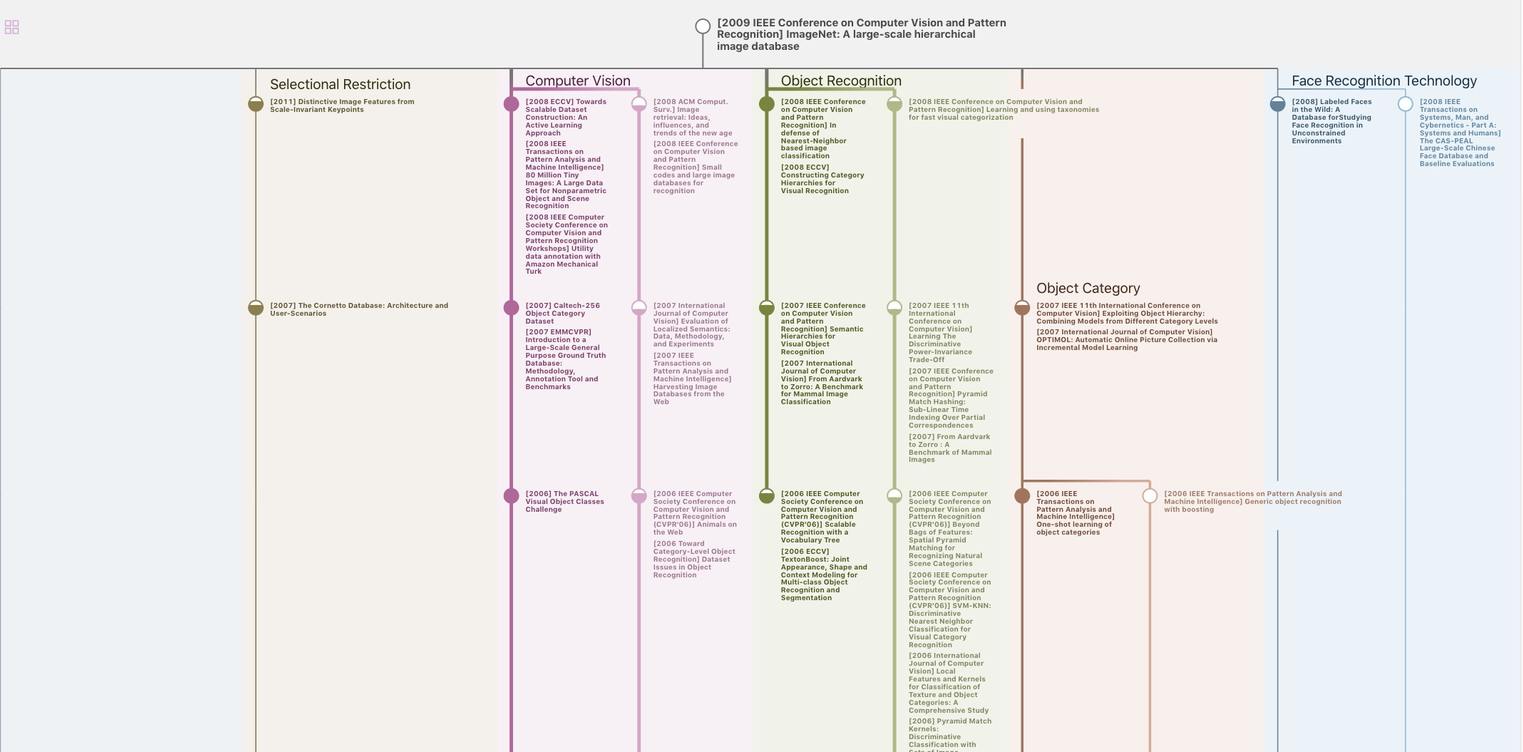
生成溯源树,研究论文发展脉络
Chat Paper
正在生成论文摘要