The validation of chest tube management after lung resection surgery using a random forest classifier
International Journal of Data Science and Analytics(2022)
摘要
After lung surgery, chest tubes and a pump are used to manage air leaks and fluid output drainage from the chest. The decision to remove or maintain chest tubes is based on drainage data collected from the digital pump which continuously monitors the patient. Follow-up data include time-stamped drainage events accompanied with corresponding chest X-ray reports and patient charts. We construct, test and validate a random forest classifier to support this clinical decision-making process by identifying patients who can have their chest tubes removed safely and timely during their follow-up. Intuitively, this problem can be modeled as a time-series fitted to monitoring data. However, we present a simpler and less demanding classifier constructed from follow-up data collected in specific, non-overlapping post-operative time frames of equal length. We hypothesize that after surgery, patients struggle to attain a stable status (favorable or adverse), which prevails after a period of discrepancies and inconsistencies in the data. We identify this time frame when the majority of patients achieve that stability. The benefits lie in improving classification performance while lowering the burden of data collection during patient treatment. We present chest tube management as a classification task performed in a sliding window over time during patient monitoring. Our results show that reliable predictions can be achieved early on in the process. Further, our classifier ensures that chest tubes that need to be maintained are not removed at the cost of potentially maintaining a few unnecessarily.
更多查看译文
关键词
Classification, Class imbalance, Machine learning, Random forests, Lung resection, Lung cancer, Digital pleural drainage, Chest tube, Thoracic surgery
AI 理解论文
溯源树
样例
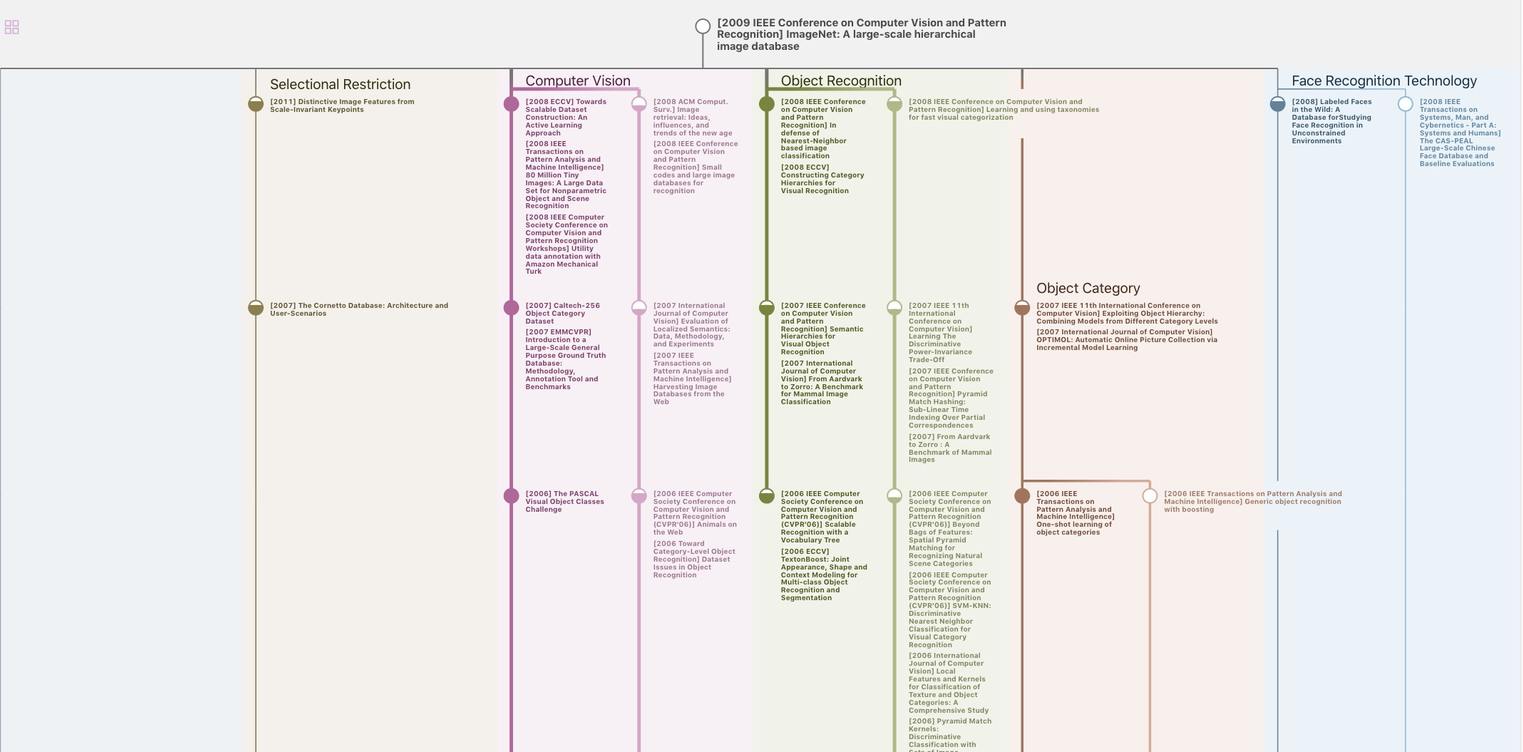
生成溯源树,研究论文发展脉络
Chat Paper
正在生成论文摘要