Gaussian process regression using partition resampling model
INTERNATIONAL JOURNAL OF MATHEMATICS AND COMPUTER SCIENCE(2022)
摘要
Gaussian process regression is an interesting probabilistic nonparametric regression method. This method is flexible in describing data patterns. Unfortunately, in the case of big data, this method has expensive computational complexity because it involves inverse matrix operations. In this study, we introduce a partition resampling method to overcome the computational complexity by reducing the dimensions of the data. The purpose of this study is to obtain a Gaussian process regression model using the partition resampling method. This method builds a model from a portion of the data. Although the model uses some data, it has the same advantages as the overall data model. This method partitions the training data and then takes one random sample from each partition to obtain data of a certain size sample. The Gaussian process regression model is a collection of functions that have hyperparameter characteristics. This study predicts new data using a hyperparameter averaging approach. We apply our method on stock price data to show that the sample size of 60% with 20 replications produces a close MAPE value of the overall data model. The computation time required is five times faster than the overall data model. So, in this case, the partition resampling method can overcome the computational complexity by reducing the data dimensions to 60%. In other cases, this method can be used as an alternative to model time series data more effectively.
更多查看译文
关键词
Gaussian processes regression, partition resampling model, computation complexity, hyperparameter, stock price prediction
AI 理解论文
溯源树
样例
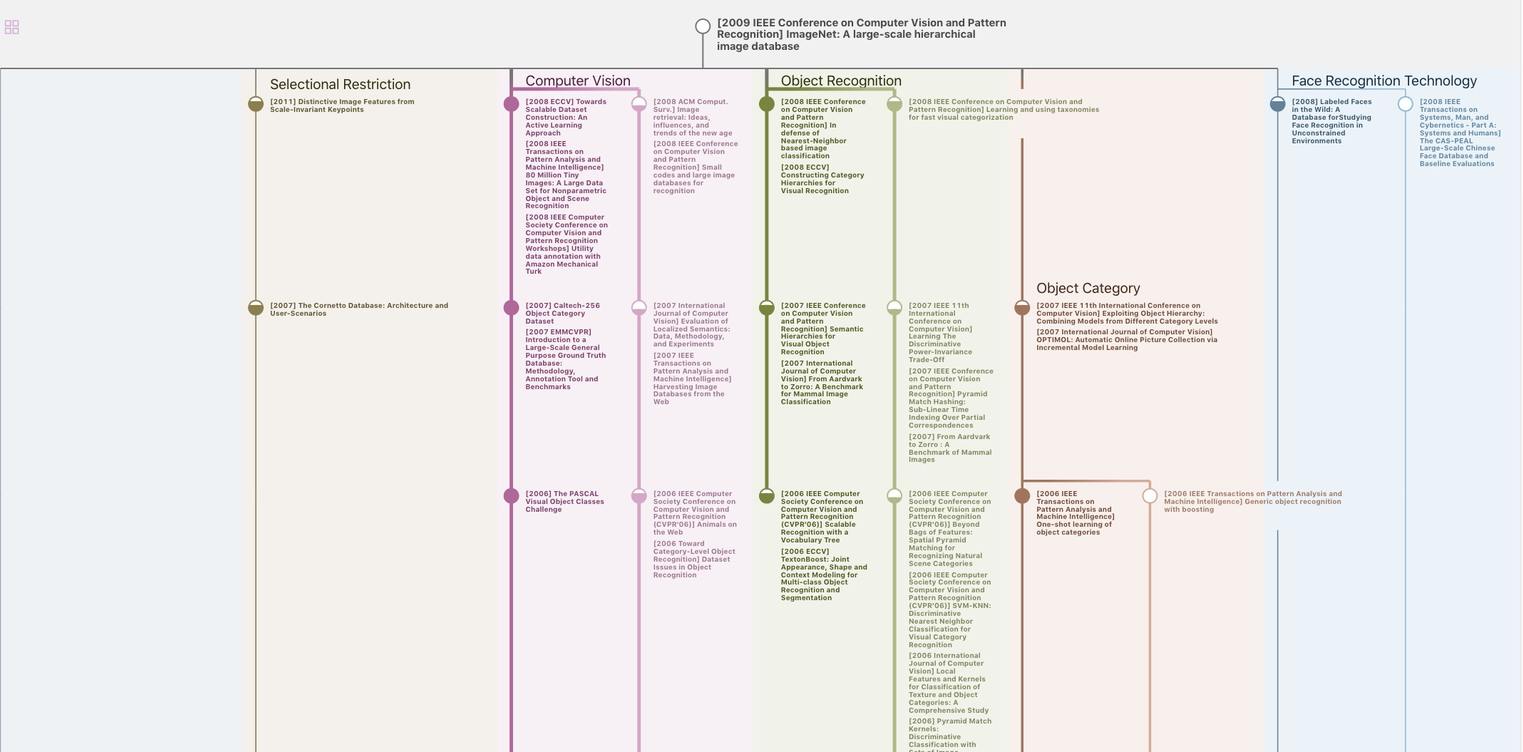
生成溯源树,研究论文发展脉络
Chat Paper
正在生成论文摘要