Improving the accuracy and robustness of RRAM-based in-memory computing against RRAM hardware noise and adversarial attacks
SEMICONDUCTOR SCIENCE AND TECHNOLOGY(2022)
摘要
We present a novel deep neural network (DNN) training scheme and resistive RAM (RRAM) in-memory computing (IMC) hardware evaluation towards achieving high accuracy against RRAM device/array variations and enhanced robustness against adversarial input attacks. We present improved IMC inference accuracy results evaluated on state-of-the-art DNNs including ResNet-18, AlexNet, and VGG with binary, 2-bit, and 4-bit activation/weight precision for the CIFAR-10 dataset. These DNNs are evaluated with measured noise data obtained from three different RRAM-based IMC prototype chips. Across these various DNNs and IMC chip measurements, we show that our proposed hardware noise-aware DNN training consistently improves DNN inference accuracy for actual IMC hardware, up to 8% accuracy improvement for the CIFAR-10 dataset. We also analyze the impact of our proposed noise injection scheme on the adversarial robustness of ResNet-18 DNNs with 1-bit, 2-bit, and 4-bit activation/weight precision. Our results show up to 6% improvement in the robustness to black-box adversarial input attacks.
更多查看译文
关键词
IMC noise-aware training, RRAM-friendly DNNs, Adversarial defense with RRAM noise
AI 理解论文
溯源树
样例
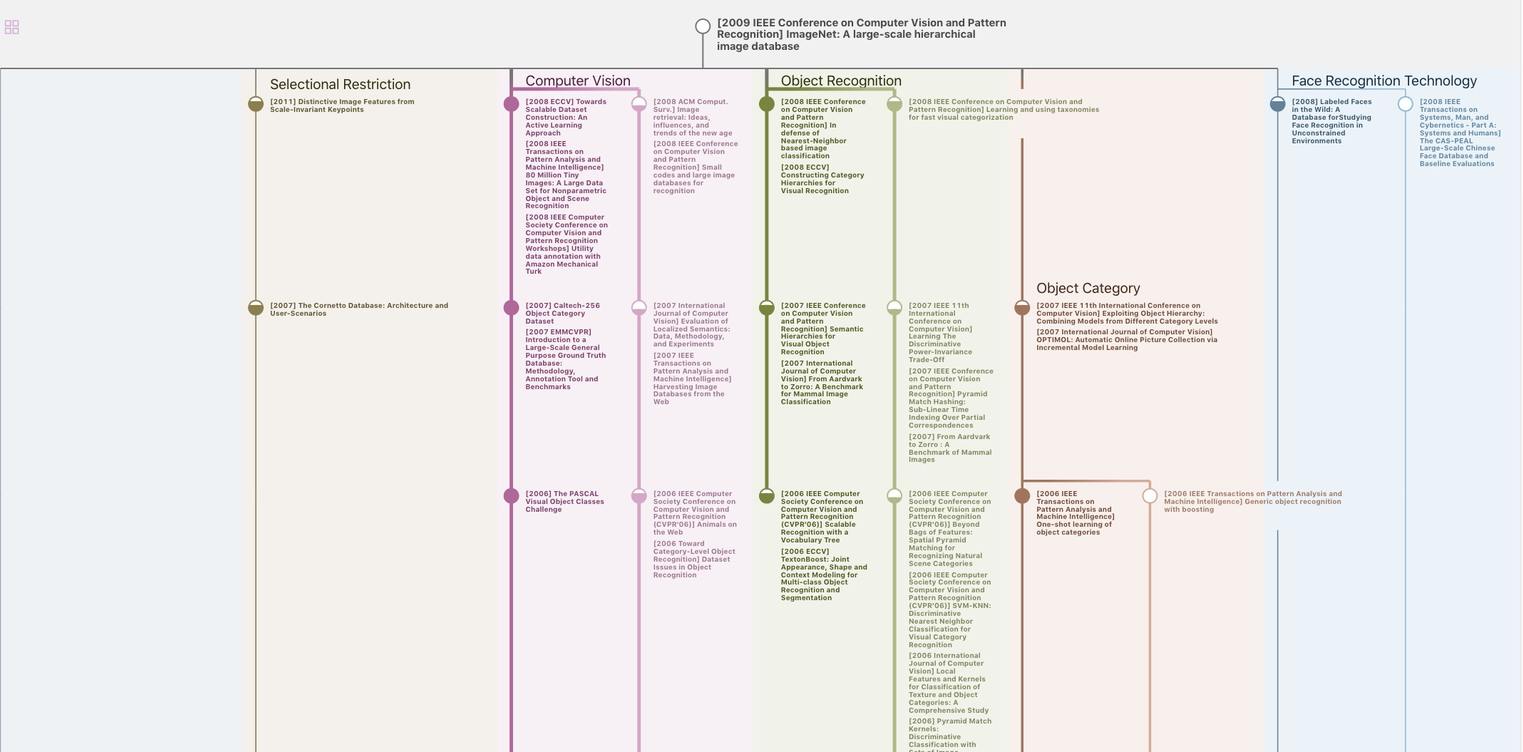
生成溯源树,研究论文发展脉络
Chat Paper
正在生成论文摘要