Removing Input Confounder for Translation Quality Estimation via a Causal Motivated Method
WEB AND BIG DATA, APWEB-WAIM 2021, PT I(2021)
摘要
Most state-of-the-art QE systems built upon neural networks have achieved promising performances on benchmark datasets. However, the performance of these methods can be easily influenced by the inherent features of the model input, such as the length of input sequence or the number of unseen tokens. In this paper, we introduce a causal inference based method to eliminate the negative impact caused by the characters of the input for a QE system. Specifically, we propose an iterative denoising framework for multiple confounding features. The confounder elimination operation at each iteration step is implemented by a Half-Sibling Regression based method. We conduct our experiments on the official datasets and submissions fromWMT 2020 Quality Estimation Shared Task of Sentence-Level Direct Assessment. Experimental results show that the denoised QE results gain better Pearson's correlation scores with human assessments compared to the original submissions.
更多查看译文
关键词
Quality estimation, Causal inference, Machine translation
AI 理解论文
溯源树
样例
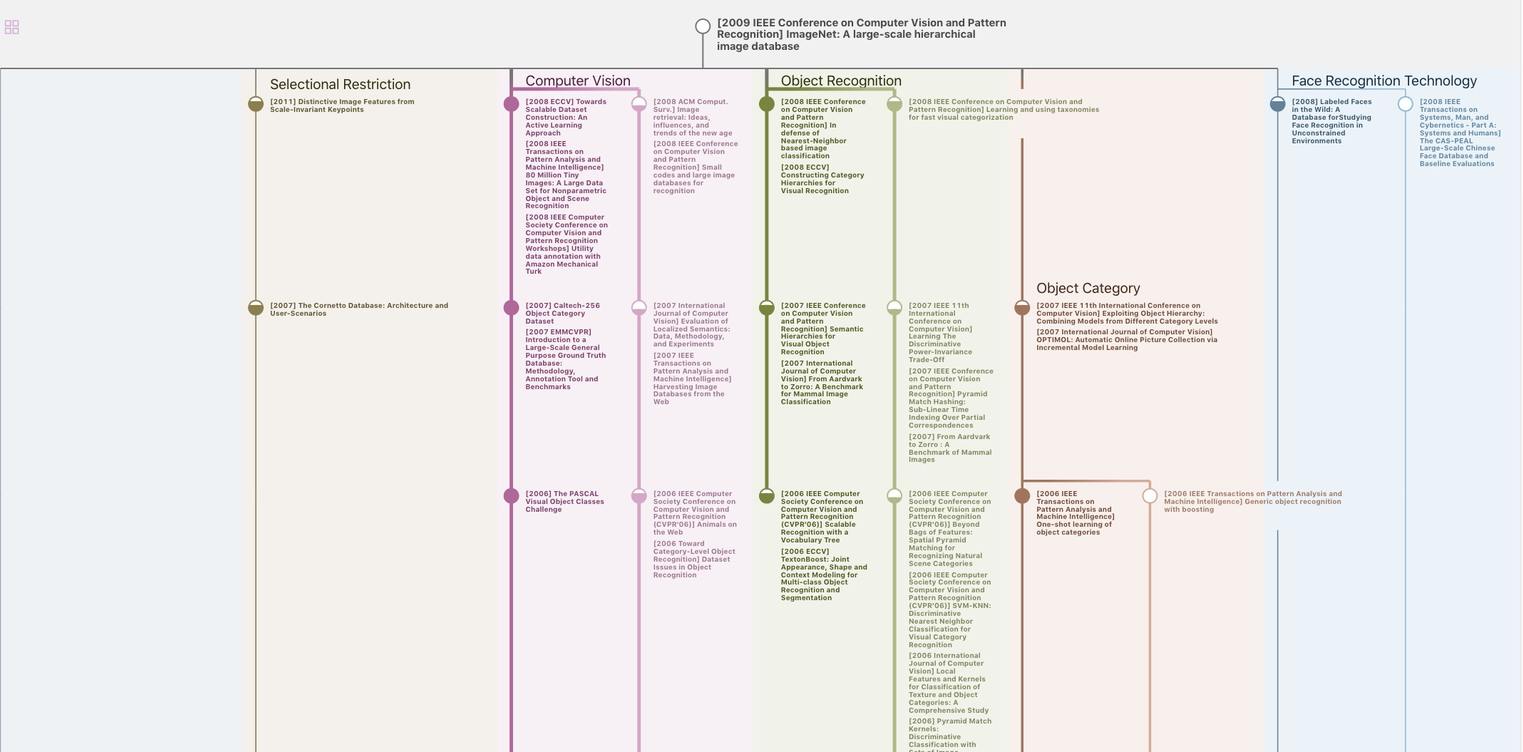
生成溯源树,研究论文发展脉络
Chat Paper
正在生成论文摘要