Text Detergent: The Systematic Combination of Text Pre-processing Techniques for Social Media Sentiment Analysis
ADVANCES ON INTELLIGENT INFORMATICS AND COMPUTING: HEALTH INFORMATICS, INTELLIGENT SYSTEMS, DATA SCIENCE AND SMART COMPUTING(2022)
摘要
During catastrophes such as natural or man-made disasters, social media services have evolved into a crucial tool utilised by communities to disseminate information. Because a vast number of social media data is being used for many applications, including sentiment analysis, sentiment analysis has become a very useful and demanding problem. Social media data cannot be applied directly because it is raw and unstructured or semi-structured data. Consequently, text pre-processing becomes one of the most important tasks because the process is strongly constrained by its dependable workflow. This reason creates a complex pattern in pre-processingworkflows. For this purpose, different text pre-processing techniques have been used on Twitter, Facebook, and YouTube datasets to study the impact of different pre-processing techniques on the accuracy of machine learning algorithms. This paper applied different text pre-processing techniques in a specific sequence based on significance testing. This study examines their influence on sentiment classification accuracy using a machine learning classifier, Support Vector Machines (SVM). Results proved that applying all 14 techniques systematically can achieve up to 82.57% of the accuracy of the SVM classifier with unigram representations. By using Text Detergent, the YouTube dataset achieve the highest accuracy compared to Facebook and Twitter datasets. This will potentially improve the quality of the text and leads to better feature extraction, which in turn helps the sentiment analyst produce a better classifier.
更多查看译文
关键词
Social media, Text pre-processing, Support vector machine, Classification, Accuracy
AI 理解论文
溯源树
样例
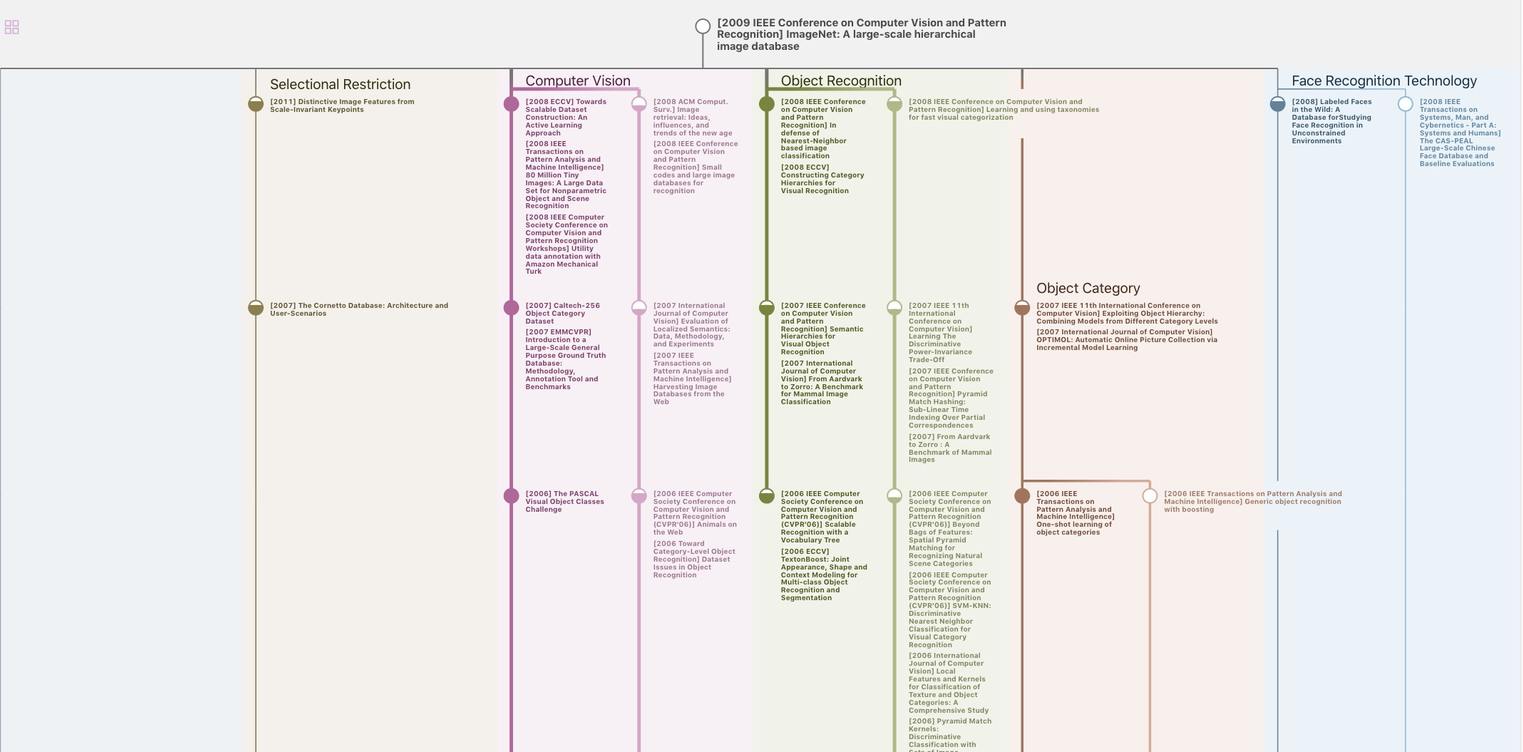
生成溯源树,研究论文发展脉络
Chat Paper
正在生成论文摘要