A Multi-Scale Convolutional Neural Network for Rotation-Invariant Recognition
ELECTRONICS(2022)
摘要
The Internet of things (IoT) enables mobile devices to connect and exchange information with others over the Internet with a lot of applications in consumer, commercial, and industrial products. With the rapid development of machine learning, IoT with image recognition capability is a new research area to assist mobile devices with processing image information. In this research, we propose the rotation-invariant multi-scale convolutional neural network (RIMS-CNN) to recognize rotated objects, which are commonly seen in real situations. Based on the dihedral group D4 transformations, the RIMS-CNN equips a CNN with multiple rotated tensors and its processing network. Furthermore, multi-scale features and shared weights are employed in the RIMS-CNN to increase performance. Compared with the data augmentation approach of using rotated images at random angles for training, our proposed method can learn inherent convolution kernels for rotational features. Experiments were conducted on the benchmark datasets: MNIST, FASHION-MNIST, CIFAR-10, and CIFAR-100. Significant improvements over the other models were achieved to show that rotational invariance could be learned.
更多查看译文
关键词
convolutional neural network, rotational invariance, multi-scale feature, dihedral group, weight sharing
AI 理解论文
溯源树
样例
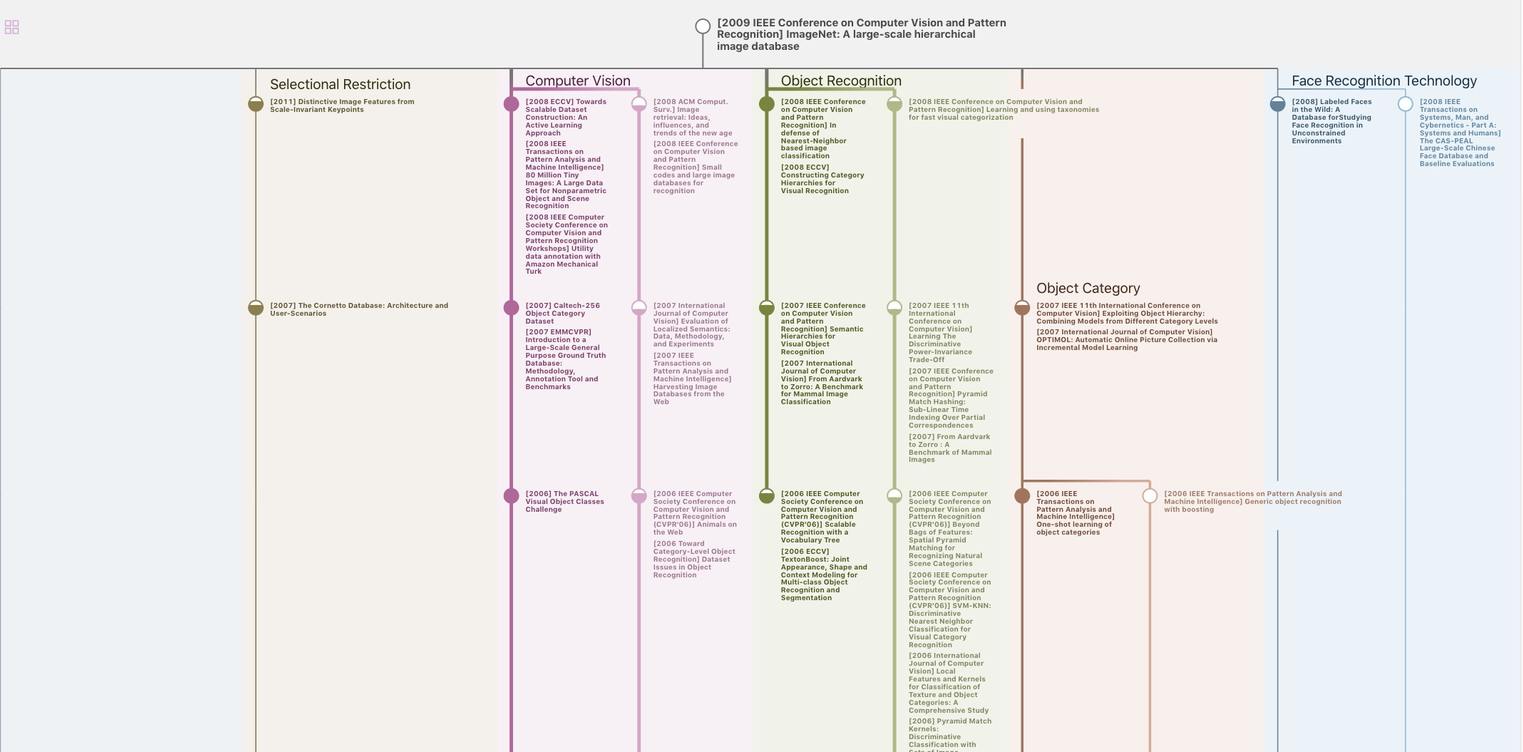
生成溯源树,研究论文发展脉络
Chat Paper
正在生成论文摘要