Global Land Cover Mapping Using Annual Clear-sky Composites from FY3D/MERSI-II
INTERNATIONAL JOURNAL OF REMOTE SENSING(2022)
摘要
Medium Resolution Spectral Imager-II (MERSI-II) on board FY-3D satellite is a visible and infrared spectral imaging instrument featured with six 250-m spatial resolution channels (blue, green, red, NIR, 10.8 mu m, 12.0 mu m). Such unique spectral and spatial attributes provide significant opportunities and challenges for land cover (LC) mapping research. This paper reports the production of a 250-m spatial resolution global LC classification (under IGBP scheme) from MERSI-II NDVI operational products from May 2019 to April 2020. To extract robust features for discriminating LC types, we adopted three classic compositing criteria (MaxNDVI, Mean8NDVI, and Mean4BT) and two new compositing criteria (3minRedBlue and 3minRedNIR) to obtain high-quality annual composites. Random Forest (RF) algorithm was used for supervised learning, and 10-fold cross validation (CV) based on 'site-splits' was implemented to evaluate classification accuracy. The derived MERIS-II LC map was compared at pixel level with two widely used LC products (MODIS and VIIRS), and consistency among the three maps was investigated. Results showed that the higher resolution afforded by 250-m MERSI-II data in combination with a refined input features produced a global LC map with an overall accuracy of 79-86%. Most classes exhibited low commission and omission errors except for some classes of mixed nature (i.e. wetland, cropland/natural vegetation mosaic, savanna, woody savanna). The MERSI-II LC map generally agreed better with MODIS than with VIIRS, and the proportion of land pixels (excluding Antarctic) for which MERSI-II shows the same LC label with either MODIS or VIIRS is very large (0.70-0.82). Composite data contributed differently to total accuracy, with Mean8NDVI being the most spatial coherent and most contributive and MaxNDVI being the opposite. The other three composites (Mean4BT, 3minRedBlue, and 3minRedNIR) showed moderate spatial coherence and contributed to classification variedly by extracting information from different phenology phases. The unique 250-m blue and green bands of MERSI-II were found to be helpful to discriminate between wetland and needleleaf forest in high latitudes and between cities and grassland. This study confirms that with the RF algorithm (one of the state-of-art supervised machine learning algorithms) and image compositing techniques, accurate LC map can be derived from MERSI-II. Future work will attempt to include more bands and compositing criteria to further improve classification accuracy.
更多查看译文
AI 理解论文
溯源树
样例
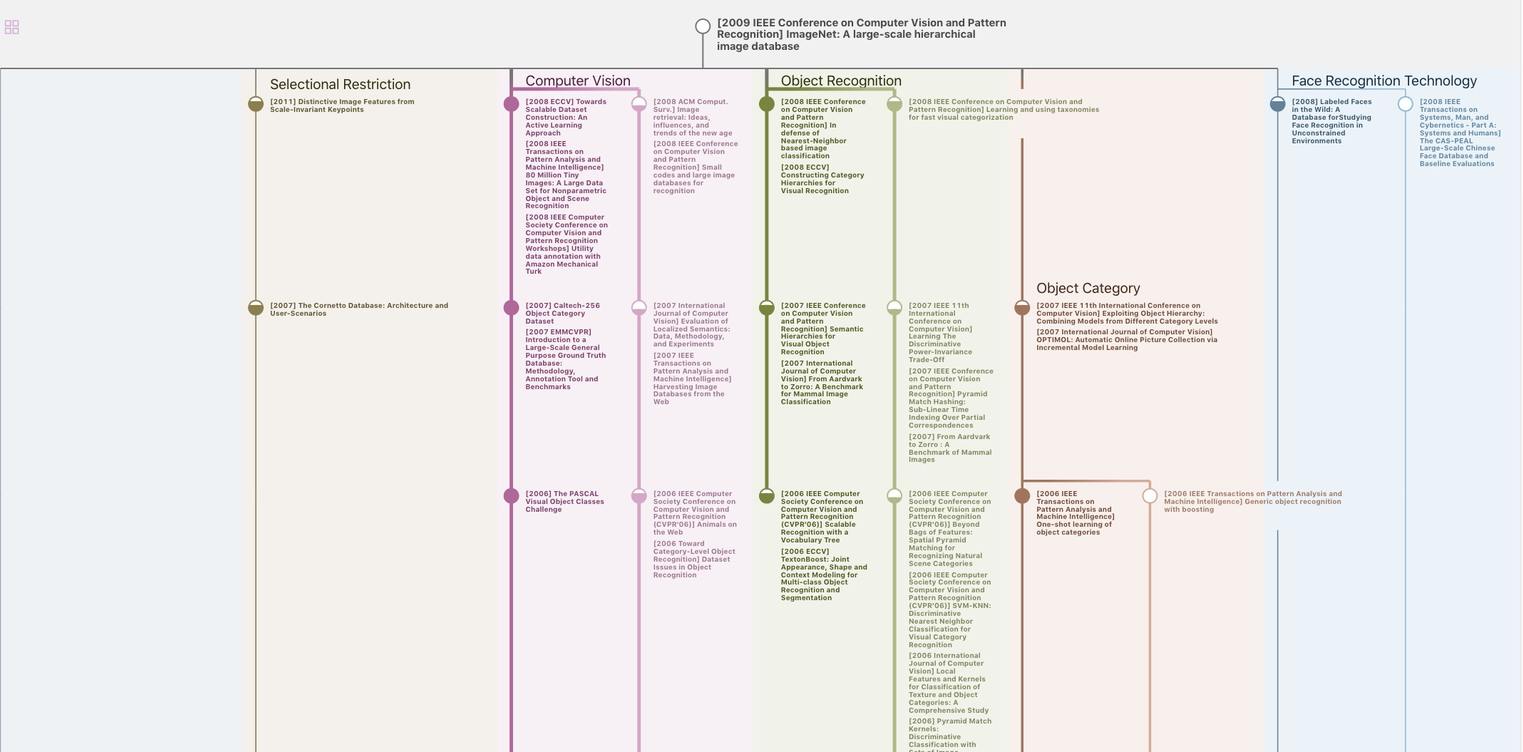
生成溯源树,研究论文发展脉络
Chat Paper
正在生成论文摘要