Deep learning for topological photonics
ADVANCES IN PHYSICS-X(2022)
摘要
In this paper, we review the specific field that combines topological photonics and deep learning (DL). Recent progress of topological photonics has attracted enormous interest for its novel and exotic properties such as unidirectional propagation of electromagnetic waves and robust manipulation of photons. These phenomena are expected to meet the growing demands of next-generation nanophotonic devices. However, to model and engineer such highly-complex systems are challenging. Recently, DL, a subset of machine learning methods using neural network (NN) algorithms, has been introduced in the field of nanophotonics as an effective way to capture a complex nonlinear relationship between design parameters and their corresponding optical properties. In particular, among various fields of nanophotonics, DL applications to topological photonics empowered by NN models have shown astonishing results in capturing the global material properties of topological systems. This review presents fundamental concepts of topological photonics and the basics of DL applied to nanophotonics in parallel. Recent studies of DL applications to topological systems using NN models are discussed thereafter. The summary and outlook showing the potential of taking data-driven approaches in topological photonics research and general physics are also discussed. [GRAPHICS] .
更多查看译文
关键词
Photonic crystal, inverse design, neural networks, nanophotonics, metamaterials
AI 理解论文
溯源树
样例
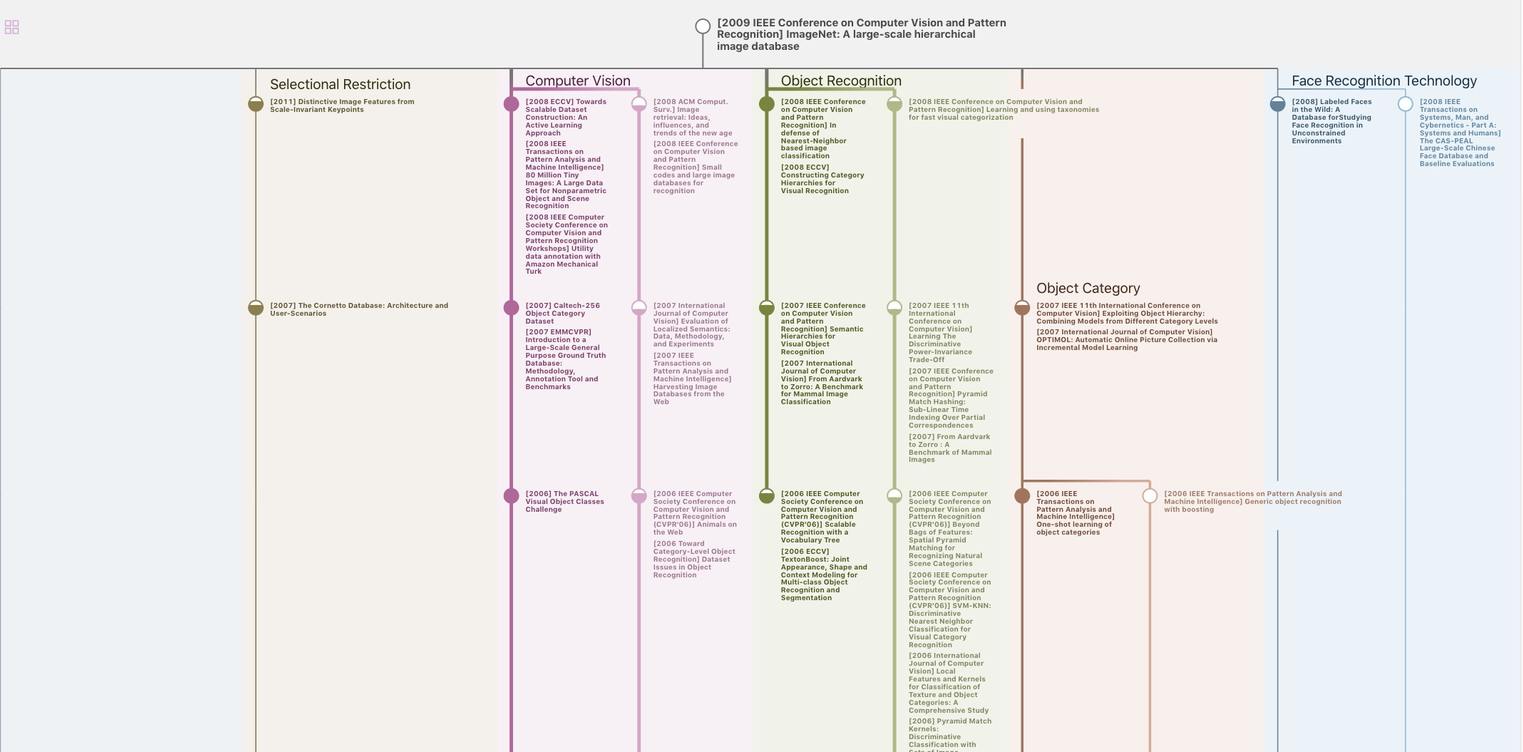
生成溯源树,研究论文发展脉络
Chat Paper
正在生成论文摘要