SPP-EEGNET: An Input-Agnostic Self-supervised EEG Representation Model for Inter-dataset Transfer Learning
PROCEEDINGS OF THE 18TH INTERNATIONAL CONFERENCE ON COMPUTING AND INFORMATION TECHNOLOGY (IC2IT 2022)(2022)
摘要
There is currently a scarcity of labeled Electroencephalography (EEG) recordings, and different datasets usually have incompatible setups (e.g., various sampling rates, number of channels, event lengths, etc.). These issues hinder machine learning practitioners from training general-purpose EEG models that can be reused on specific EEG classification tasks through transfer learning. We present a deep convolutional neural network architecture with a spatial pyramid pooling layer that is able to take in EEG signals of varying dimensionality and extract their features to fixed-size vectors. The model is trained with a contrastive self-supervised learning task on a large unlabelled dataset. We introduce a set of EEG signal augmentation techniques to generate large amounts of sample pairs to train the feature extractor. We then transfer the trained feature extractor to new downstream tasks. The experimental results(1) show that the first few convolutional layers of the feature extractor learn the general features of the EEG data, which can significantly improve the classification performance on new datasets (The source code on Github: https://github.com/imics-lab/eeg-transfer-learning).
更多查看译文
关键词
EEG, Self-supervised learning, Contrastive learning, Transfer learning, Spatial pyramid pooling
AI 理解论文
溯源树
样例
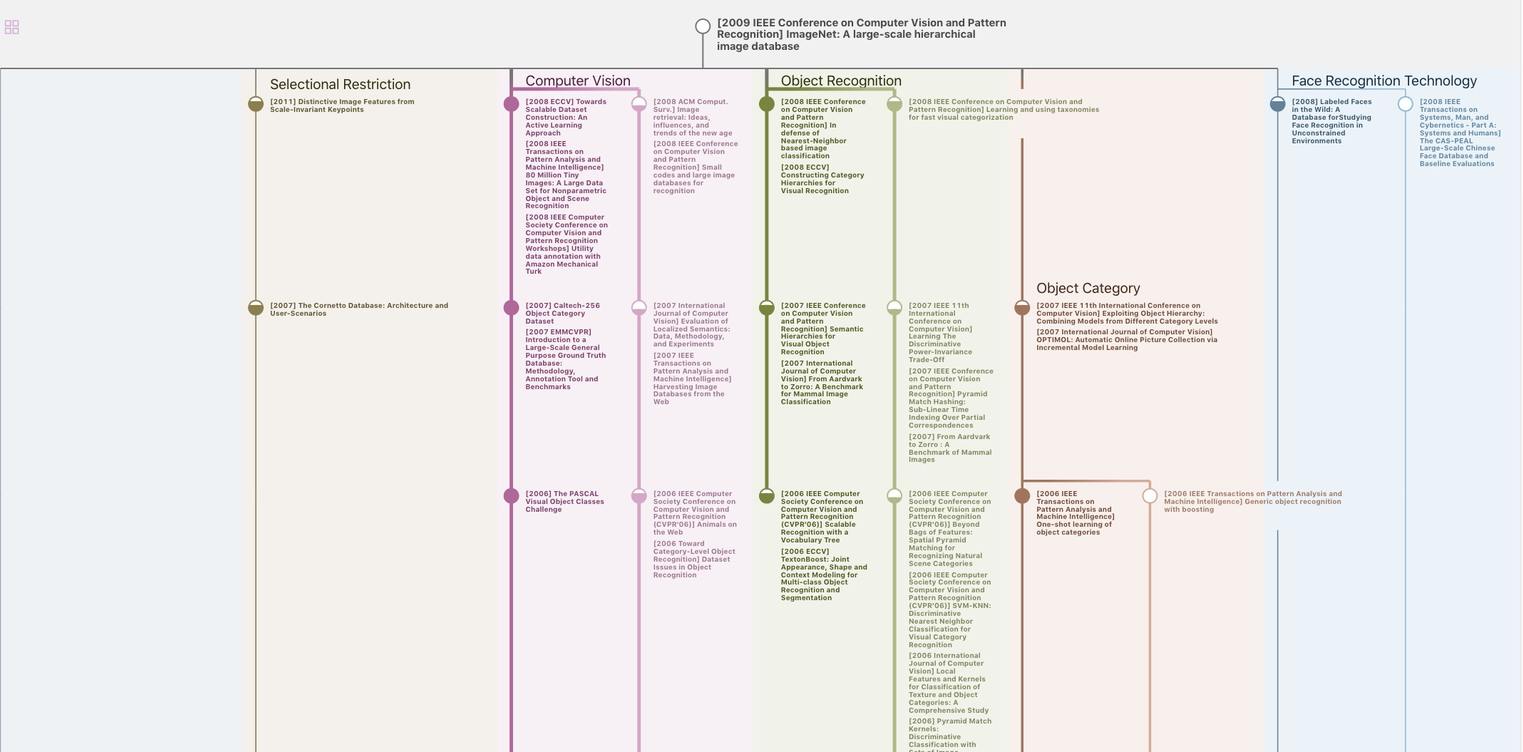
生成溯源树,研究论文发展脉络
Chat Paper
正在生成论文摘要