Evaluation of Odor Prediction Model Performance and Variable Importance according to Various Missing Imputation Methods
APPLIED SCIENCES-BASEL(2022)
摘要
The aim of this study is to ascertain the most suitable model for predicting complex odors using odor substance data that has a small number of data and a large number of missing data. First, we compared the data removal and imputation methods, and the method of imputing missing data was found to be more effective. Then, in order to recommend a suitable model, we created a total of 126 models (missing imputation: single imputation, multiple imputations, K-nearest neighbor imputation; data preprocessing: standardization, principal component analysis, partial least square; and predictive method: multiple regression, machine learning, deep learning) and compared them using R-2 and mean absolute error (MAE) values. Finally, we investigated variable importance using the best prediction model. The results identified the best model as a combination of multivariate imputation using Bayesian ridge as the missing imputation method, standardization for data preprocessing, and an extremely randomized tree as the predictive method. Among the odor compounds, Methyl mercaptan, acetic acid, and dimethyl sulfide were identified as the most important odor compounds in predicting complex odors.
更多查看译文
关键词
odor substance, variable importance, missing data, imputation, odor prediction
AI 理解论文
溯源树
样例
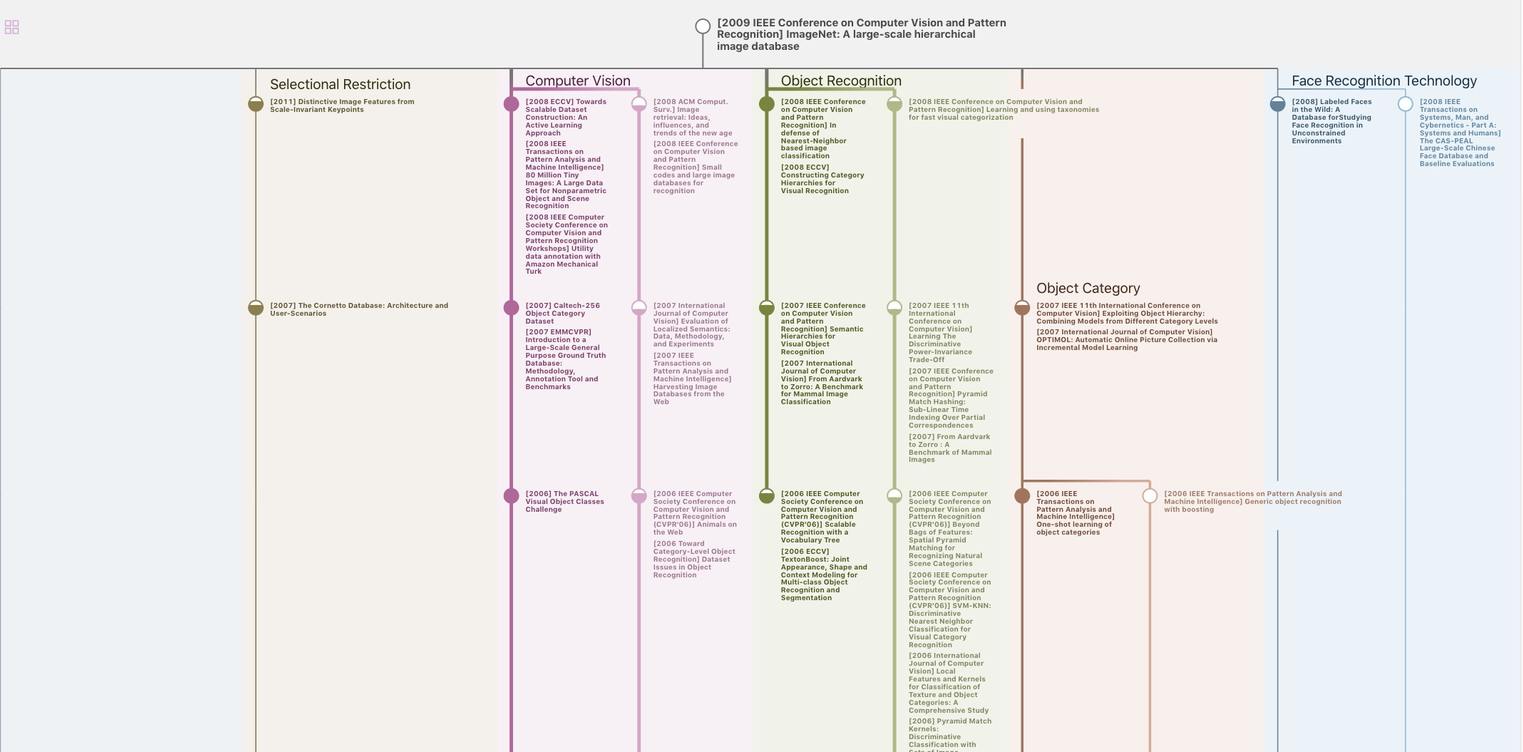
生成溯源树,研究论文发展脉络
Chat Paper
正在生成论文摘要