THE ROLE OF DEEP LEARNING IN PREDICTING COMPLEXITY AND COMPLICATIONS IN ABDOMINAL WALL RECONSTRUCTION
BRITISH JOURNAL OF SURGERY(2021)
摘要
Abstract Aim The aim of our study was to evaluate the utility of image-based deep learning models (DLMs) to predict surgical complexity and postoperative outcomes in patients undergoing AWR. Material and Methods A prospective, tertiary center, hernia database was queried for open AWR patients with adequate pre-operative CT-scans. An 8-layer convolutional neural network (CNN) analyzed image characteristics in Python utilizing the open source Tensorflow© and OpenCV frameworks. Images were analyzed and batched into a training set (80%) and validation set (20%) used to analyze the model output, which was blinded to the CNN until testing. DLMs were run to assess surgical complexity based on need for component separation, surgical site infection (SSI), and pulmonary failure. The surgical complexity DLM was validated by comparison to 6 expert AWR surgeons. Results In total, 369 patient CT scans were utilized. The surgical complexity DLM performed well (ROC=0.744;p<0.0001), and when compared to surgeon prediction on the validation set, performed better with an accuracy of 81.3% compared to 65.0% (p < 0.0001). The SSI DLM was successful with an ROC of 0.898 (p < 0.0001). The DLM for predicting pulmonary failure was less effective with an ROC of 0.545 (p = 0.03). Conclusions DLMs were able to successfully predict surgical complexity and were more accurate than expert surgeons using objective, pre-operative imaging. DLMs were also successful in predicting SSI. This breakthrough may allow for enhanced pre-operative planning, including resource utilization and possible need for tertiary center referral. AI appears to be an exciting new management tool in complex AWR.
更多查看译文
AI 理解论文
溯源树
样例
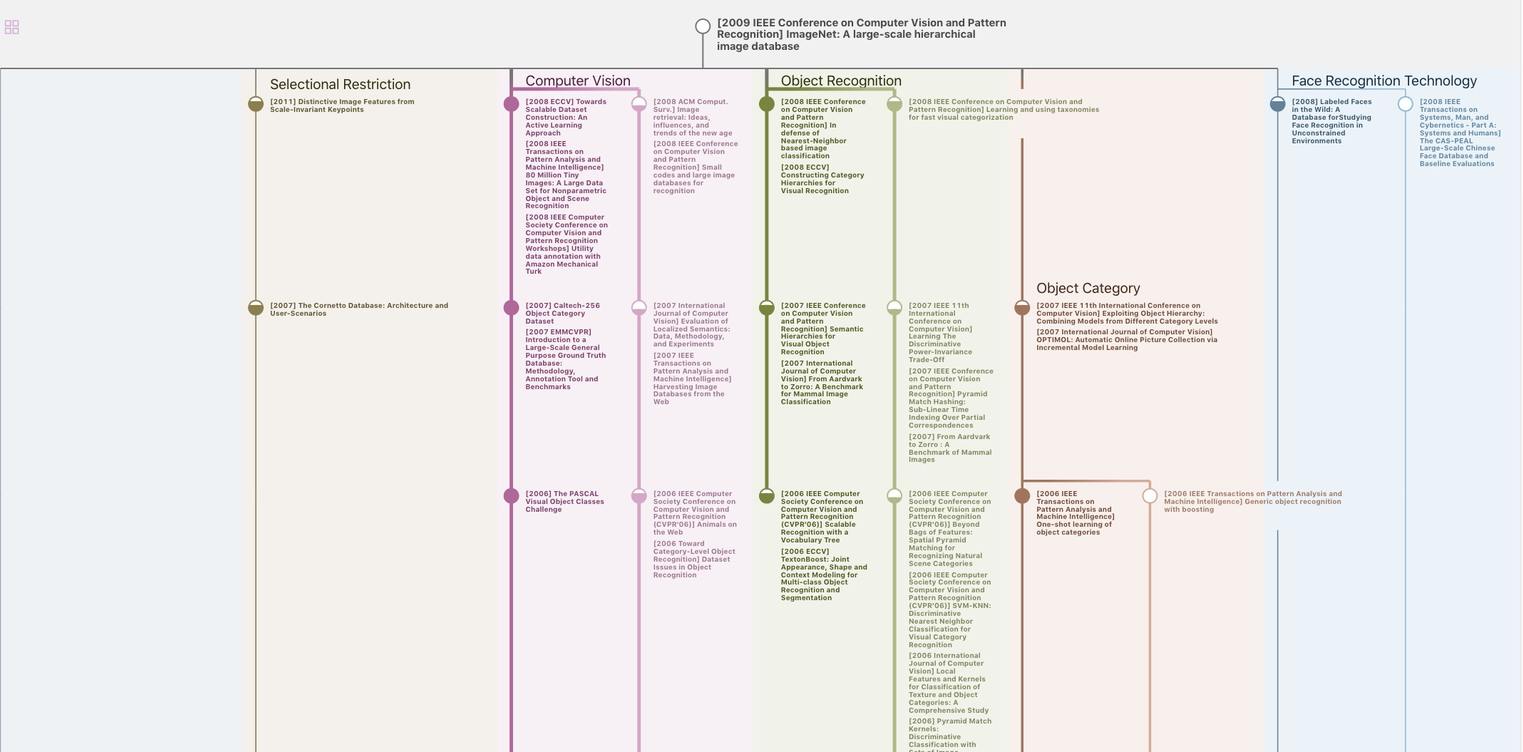
生成溯源树,研究论文发展脉络
Chat Paper
正在生成论文摘要