Protein function prediction for newly sequenced organisms
NATURE MACHINE INTELLIGENCE(2021)
摘要
Recent successes in protein function prediction have shown the superiority of approaches that integrate multiple types of experimental evidence over methods that rely solely on homology. However, newly sequenced organisms continue to represent a difficult challenge, because only their protein sequences are available and they lack data derived from large-scale experiments. Here we introduce S2F (Sequence to Function), a network propagation approach for the functional annotation of newly sequenced organisms. Our main idea is to systematically transfer functionally relevant data from model organisms to newly sequenced ones, thus allowing us to use a label propagation approach. S2F introduces a novel label diffusion algorithm that can account for the presence of overlapping communities of proteins with related functions. As most newly sequenced organisms are bacteria, we tested our approach in the context of bacterial genomes. Our extensive evaluation shows a great improvement over existing sequence-based methods, as well as four state-of-the-art general-purpose protein function prediction methods. Our work demonstrates that employing a diffusion process over networks of transferred functional data is an effective way to improve predictions over simple homology. S2F is applicable to any type of newly sequenced organism as well as to those for which experimental evidence is available. A free, easy to run version of S2F is available at https://www.paccanarolab.org/s2f .
更多查看译文
关键词
Computational science,Protein function predictions,Engineering,general
AI 理解论文
溯源树
样例
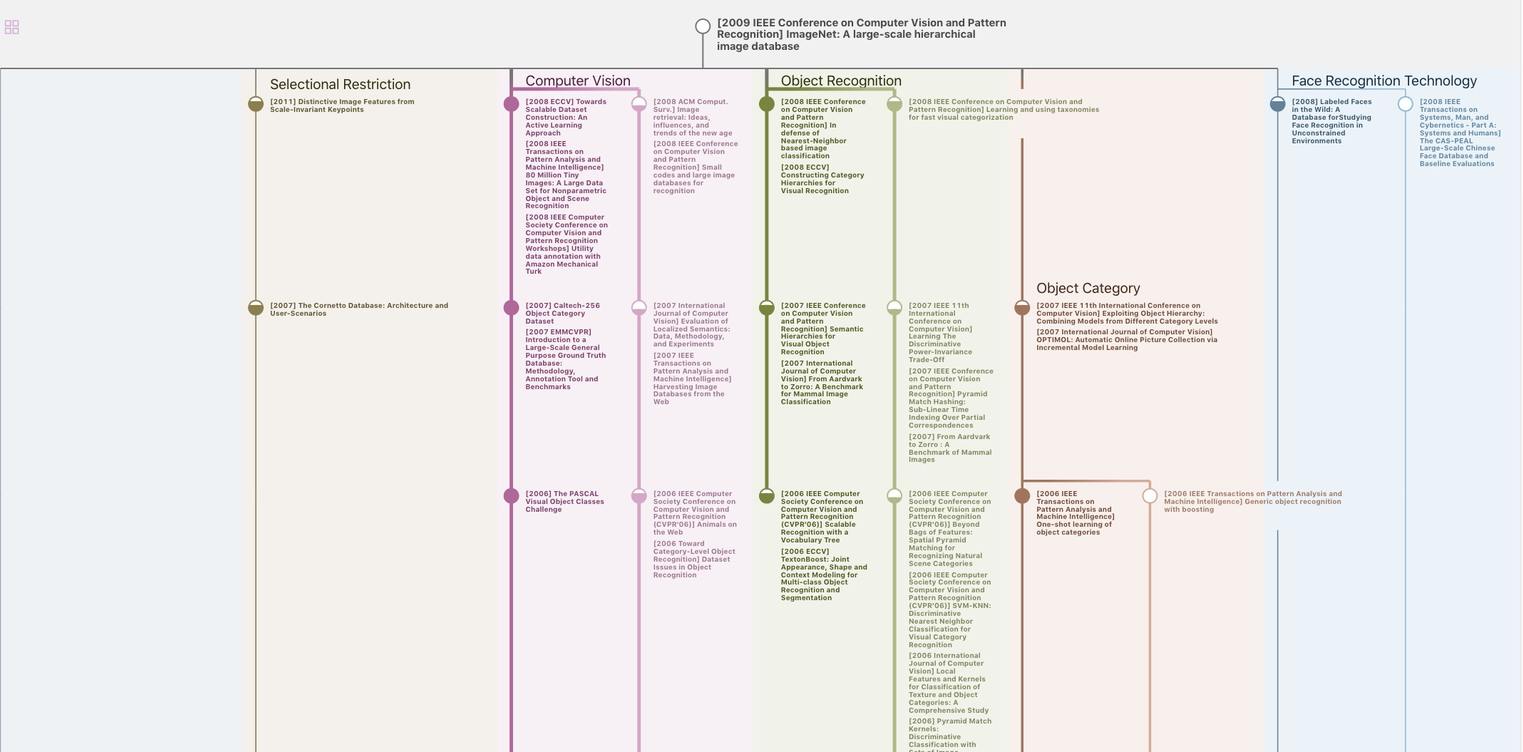
生成溯源树,研究论文发展脉络
Chat Paper
正在生成论文摘要