A Deep Learning Approach for Semantic Analysis of COVID-19-Related Stigma on Social Media
IEEE Transactions on Computational Social Systems(2023)
摘要
The rapid spread of the pandemic of coronavirus disease of 2019 (COVID-19) has created an unprecedented, global health disaster. During the outburst period, the paucity of knowledge and research aggravated devastating panic and fears that lead to social stigma and created serious obstacles to contain the disastrous epidemic. We propose a deep learning-based method to detect stigmatized contents on online social network (OSN) platforms in the early stage of COVID-19. Our method performs a semantic-based quantitative analysis to unveil essential spatial-temporal characteristics of COVID-19 stigmatization for timely alerts and risk mitigation. Empirical evaluations are carried out to examine our method’s predictive utilities. The visualization results of the co-occurrence network using Gephi indicate two distinct groups of stigmatized words that pertain to people in Wuhan and their dietary behaviors, respectively. Netizens’ participations and stigmatizations in the Hubei region, where the COVID-19 broke out, are twice (
$p < 0.05$
) and four (
$p < 0.01$
) times more frequent and intense than those in other parts of China, respectively. Also, the number of COVID-19 patients is correlated with COVID-19-related stigma significantly (correlation coefficient = 0.838,
$p < 0.01$
). The responses to individual users’ posts have the power law distribution, while posts by official media appear to attract more responses (e.g., likes, replies, and forward). Our method can help platforms and government agencies manage public health disasters through effective identification and detailed analyses of social stigma on social media.
更多查看译文
关键词
Coronavirus disease of 2019 (COVID-19),deep learning,public sentiment analysis,social media,social stigma
AI 理解论文
溯源树
样例
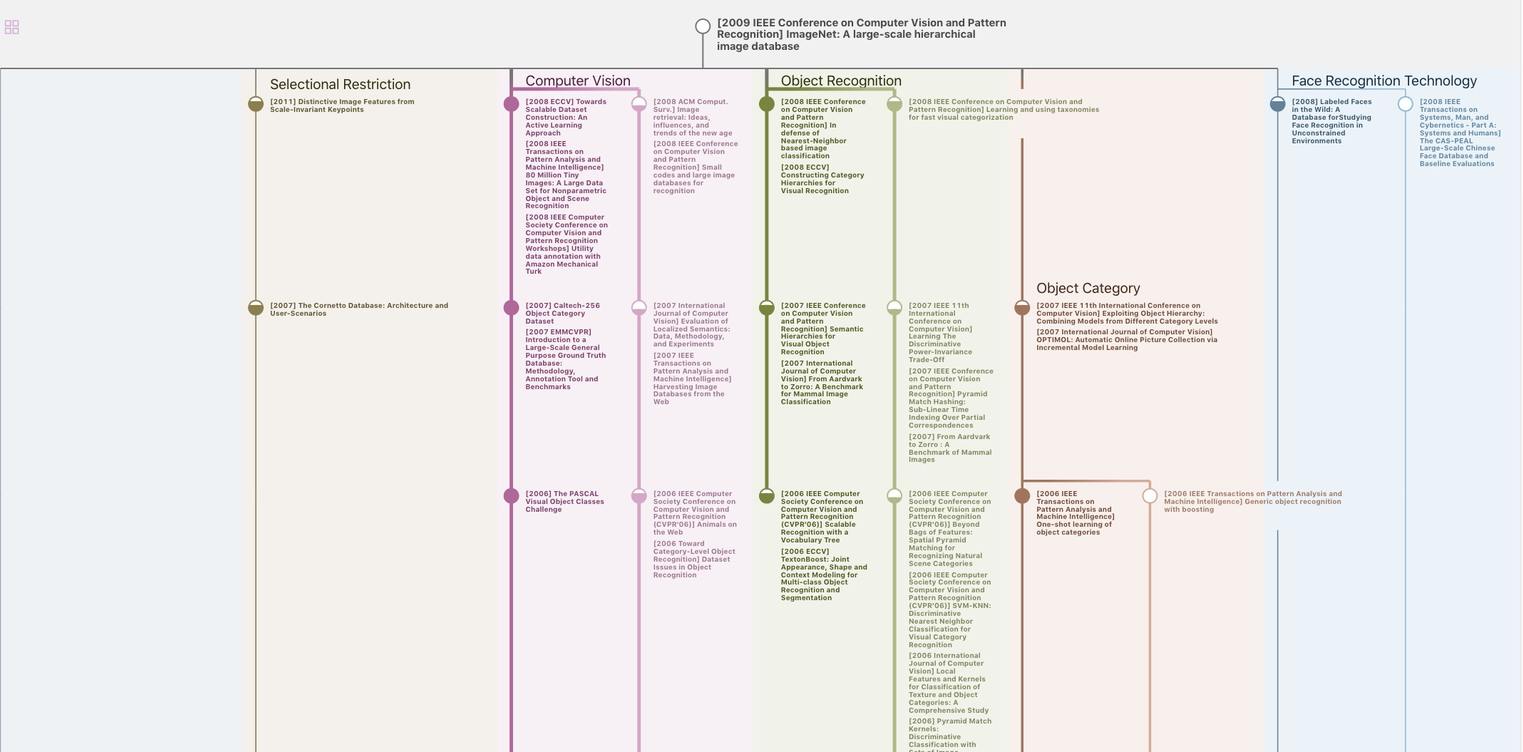
生成溯源树,研究论文发展脉络
Chat Paper
正在生成论文摘要