Noise Modeling to Build Training Sets for Robust Speech Enhancement
APPLIED SCIENCES-BASEL(2022)
摘要
DNN-based Speech Enhancement (SE) models suffer from significant performance degradation in real recordings due to the mismatch between the synthetic datasets employed for training and real test sets. To solve this problem, we propose a new Generative Adversarial Network framework for Noise Modeling (NM-GAN) that creates realistic paired training sets by imitating real noise distribution. The proposed framework combines a novel 7-layer U-Net with two bidirectional long short-term memory (LSTM) layers that act as a generator to construct complex noise. NM-GAN generates enough recall (diversity) and precision (noise quality) in its samples through adversarial and alternate training, effectively simulating real noise, which is then utilized to compose realistic paired training sets. Extensive experiments employing various qualitative and quantitative evaluation metrics verify the effectiveness of the generated noise samples and training sets, demonstrating our framework's capabilities.
更多查看译文
关键词
Noise Modeling, training set, Generative Adversarial Network, speech enhancement
AI 理解论文
溯源树
样例
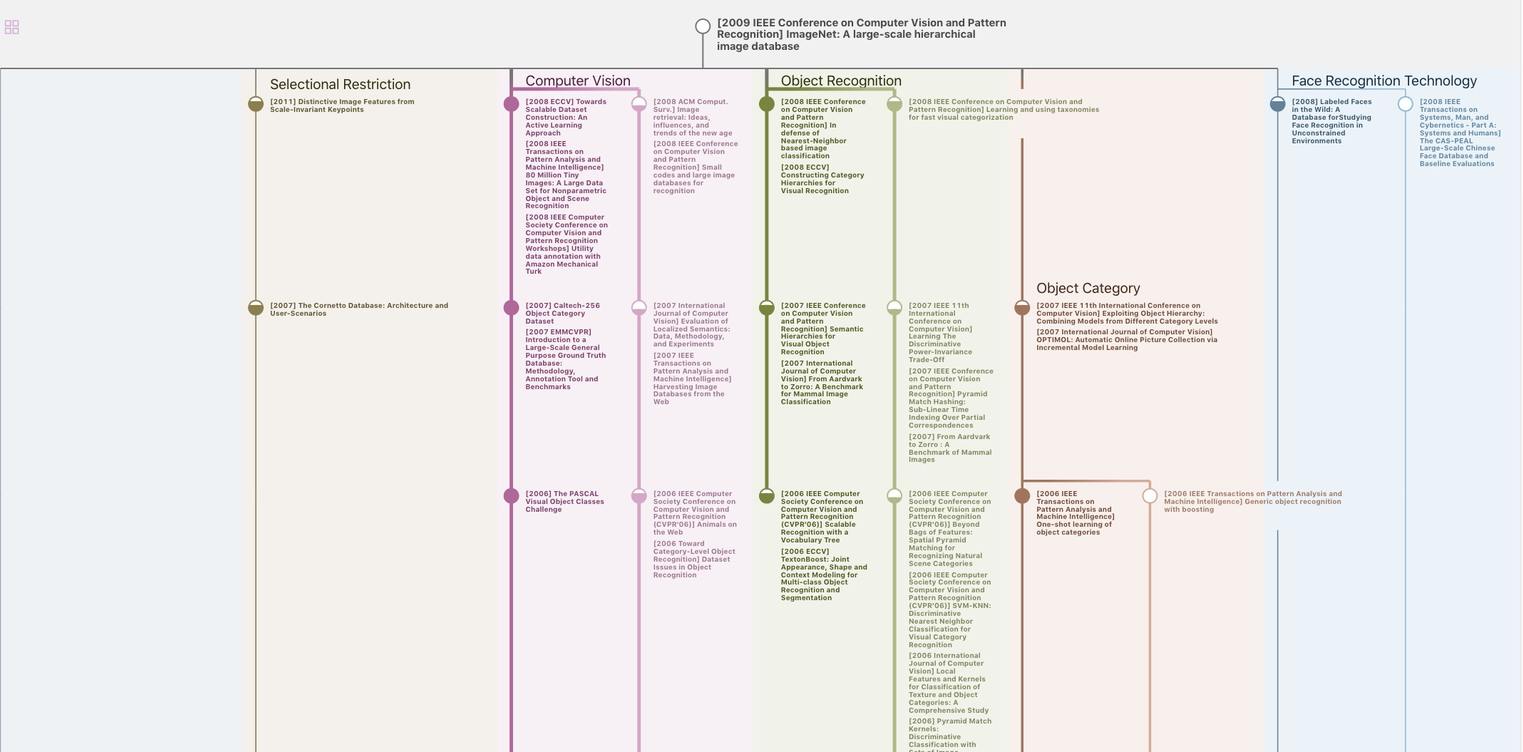
生成溯源树,研究论文发展脉络
Chat Paper
正在生成论文摘要