Performance measurements of machine learning and different neural network designs for prediction of geochemical properties based on hyperspectral core scans
AUSTRALIAN JOURNAL OF EARTH SCIENCES(2022)
摘要
Performance results of predictive models for estimating chemistry grades for gold, copper and iron in drill cores, based on the mineralogy data derived from the hyperspectral observations and using automated tools are presented. The models were built using data from more than 700 km of drill core. Ten commonly used machine learning and neural network algorithms, including convolutional neural networks (CNNs), were assessed for classifying ore grades, with accuracy and errors reported through confusion matrixes. The CNN algorithm was the outstanding performer, with an averaged classifier accuracy of 80%, outperforming the other machine-learning methods and the DenseNet deep learning method. Also discussed is the outcome of using fewer ore-grade classes that led to better predictive accuracy. This work provides insight into the potential for predicting geochemistry from hyperspectral data to support exploration geologists in target detection.
更多查看译文
关键词
exploration, hyperspectral, machine learning, neural network
AI 理解论文
溯源树
样例
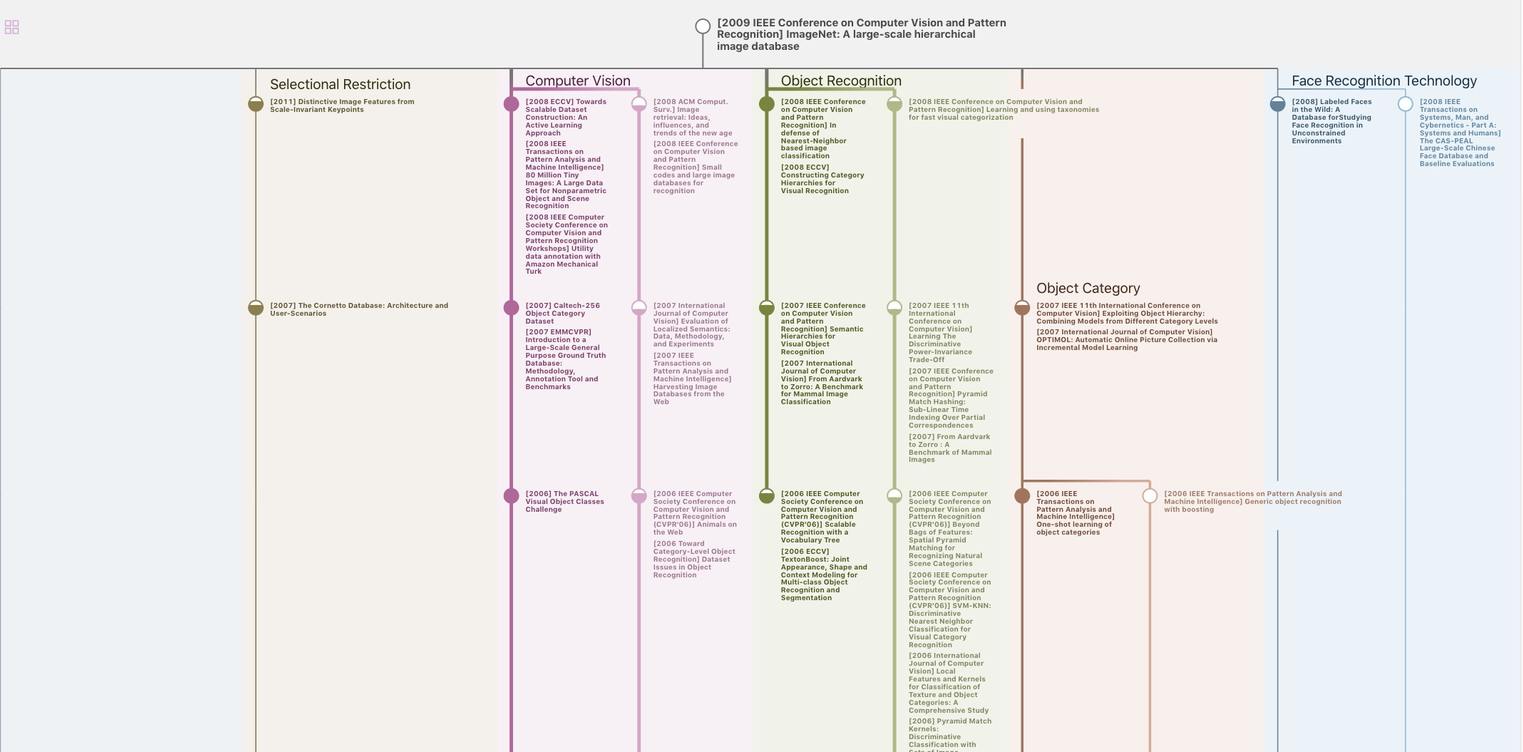
生成溯源树,研究论文发展脉络
Chat Paper
正在生成论文摘要