Scan Quality Estimation for Industrial Computed Tomography Using Convolutional Neural Networks
FIFTEENTH INTERNATIONAL CONFERENCE ON QUALITY CONTROL BY ARTIFICIAL VISION(2021)
摘要
Artefacts in industrial Computed Tomography (CT) restrict the image quality of a CT scan and limit evaluations such as inspections for material defects or dimensional measurements. Due to a large variety of scanning objects made of different materials and of various part sizes, artefacts appear in various ways in the reconstructed image. Existing analytical approaches allow quantifying the CT scan quality, but still a lack of generalizability exists. In our work, a scan quality estimation based on a Convolutional Neural Network (CNN) is proposed. The network is trained with projection images of various objects. The images are labeled by pair-wise comparison by an experienced user with a scalar quality value. The training is performed on 94 scans covering a broad range of objects. Our work showed a test accuracy of the final trained network of up to 95 %. In order to comprehend the "black box" CNN, visualizations of the network's feature maps are analyzed.
更多查看译文
关键词
Industrial Computed Tomography, Convolutional Neural Network, Scan Quality Estimation, X-Ray, Artefacts, Feature Maps
AI 理解论文
溯源树
样例
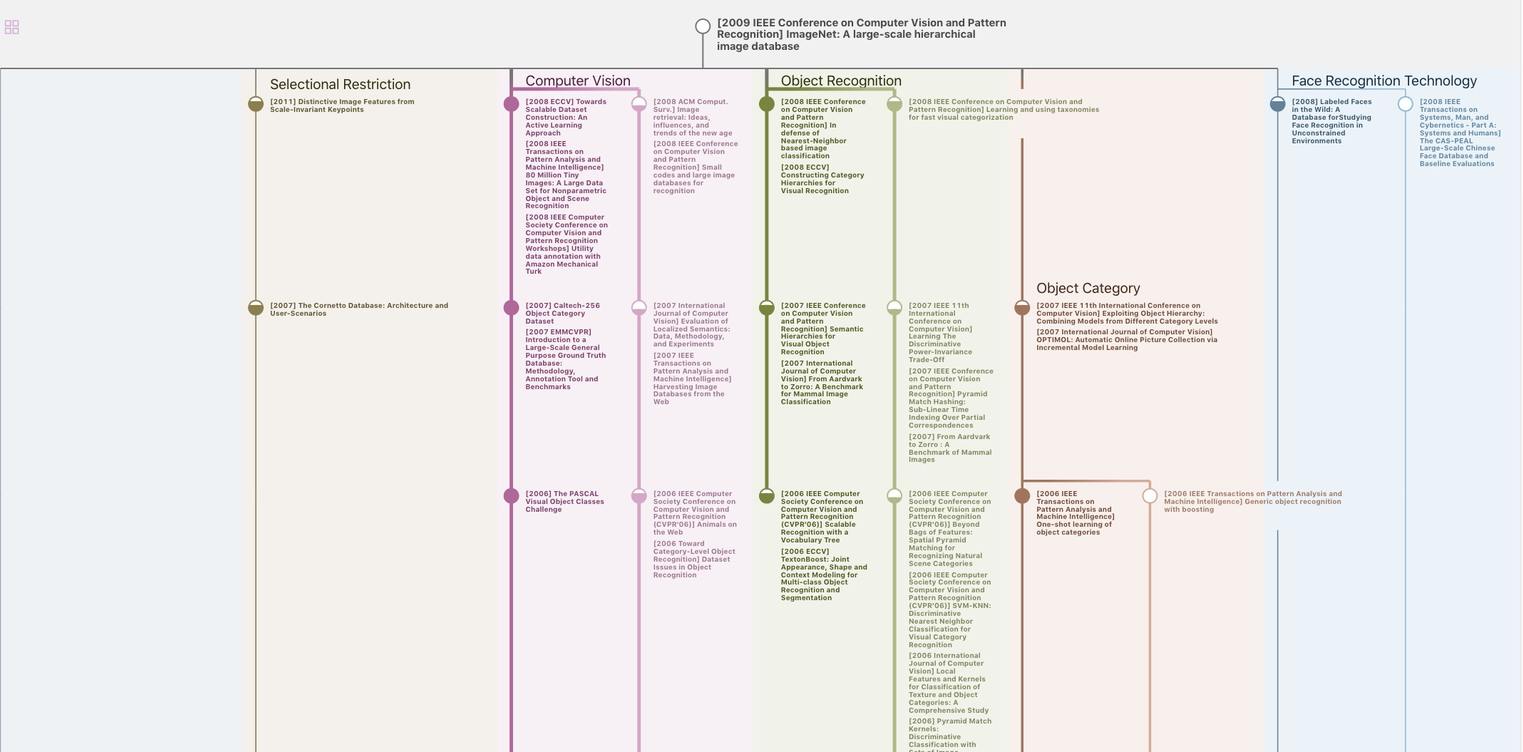
生成溯源树,研究论文发展脉络
Chat Paper
正在生成论文摘要