Bayesian Nonparametric Estimation of Ex Post Variance
JOURNAL OF FINANCIAL ECONOMETRICS(2021)
摘要
Variance estimation is central to many questions in finance and economics. Until now ex post variance estimation has been based on infill asymptotic assumptions that exploit high-frequency data. This article offers a new exact finite sample approach to estimating ex post variance using Bayesian nonparametric methods. In contrast to the classical counterpart, the proposed method exploits pooling over high-frequency observations with similar variances. Bayesian nonparametric variance estimators under no noise, heteroskedastic and serially correlated microstructure noise are introduced and discussed. Monte Carlo simulation results show that the proposed approach can increase the accuracy of variance estimation. Applications to equity data and comparison with realized variance and realized kernel estimators are included.
更多查看译文
关键词
Dirichlet process mixture, pooling, realized kernel, shrinkage
AI 理解论文
溯源树
样例
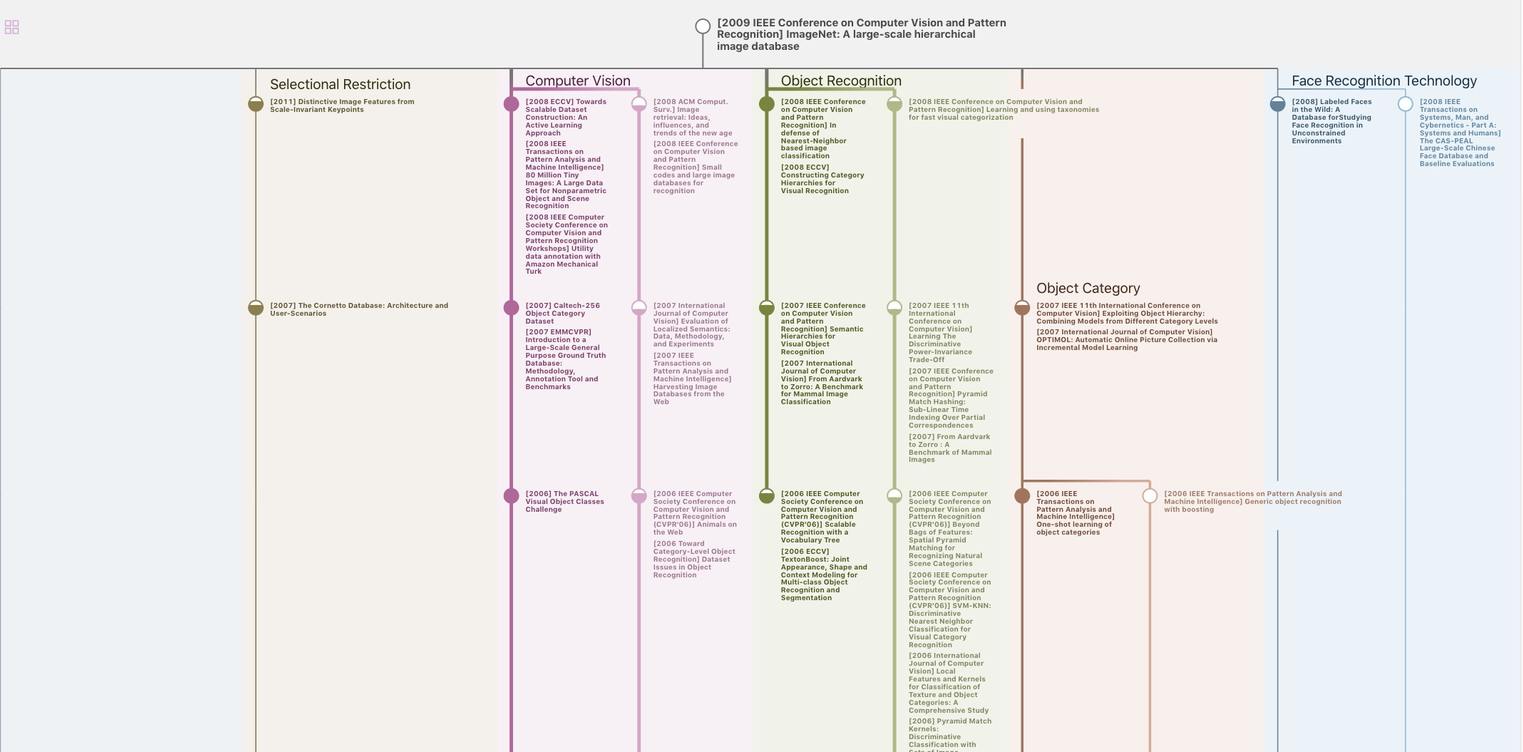
生成溯源树,研究论文发展脉络
Chat Paper
正在生成论文摘要